Analysis and Forecast of Traffic Flow between Urban Functional Areas Based on Ride-Hailing Trajectories
ISPRS INTERNATIONAL JOURNAL OF GEO-INFORMATION(2023)
摘要
Urban planning and function layout have important implications for the journeys of a large percentage of commuters, which often make up the majority of daily traffic in many cities. Therefore, the analysis and forecast of traffic flow among urban functional areas are of great significance for detecting urban traffic flow directions and traffic congestion causes, as well as helping commuters plan routes in advance. Existing methods based on ride-hailing trajectories are relatively effective solution schemes, but they often lack in-depth analyses on time and space. In the paper, to explore the rules and trends of traffic flow among functional areas, a new spatiotemporal characteristics analysis and forecast method of traffic flow among functional areas based on urban ride-hailing trajectories is proposed. Firstly, a city is divided into areas based on the actual urban road topology, and all functional areas are generated by using areas of interest (AOI); then, according to the proximity and periodicity of inter-area traffic flow data, the periodic sequence and the adjacent sequence are established, and the topological structure is learned through graph convolutional neural (GCN) networks to extract the spatial correlation of traffic flow among functional areas. Furthermore, we propose an attention-based gated graph convolutional network (AG-GCN) forecast method, which is used to extract the temporal features of traffic flow among functional areas and make predictions. In the experiment, the proposed method is verified by using real urban traffic flow data. The results show that the method can not only mine the traffic flow characteristics among functional areas under different time periods, directions, and distances, but also forecast the spatiotemporal change trend of traffic flow among functional areas in a multi-step manner, and the accuracy of the forecasting results is higher than that of common benchmark methods, reaching 96.82%.
更多查看译文
关键词
traffic flow forecast, functional area, ride-hailing trajectories, spatiotemporal correlation, graph convolution neural network
AI 理解论文
溯源树
样例
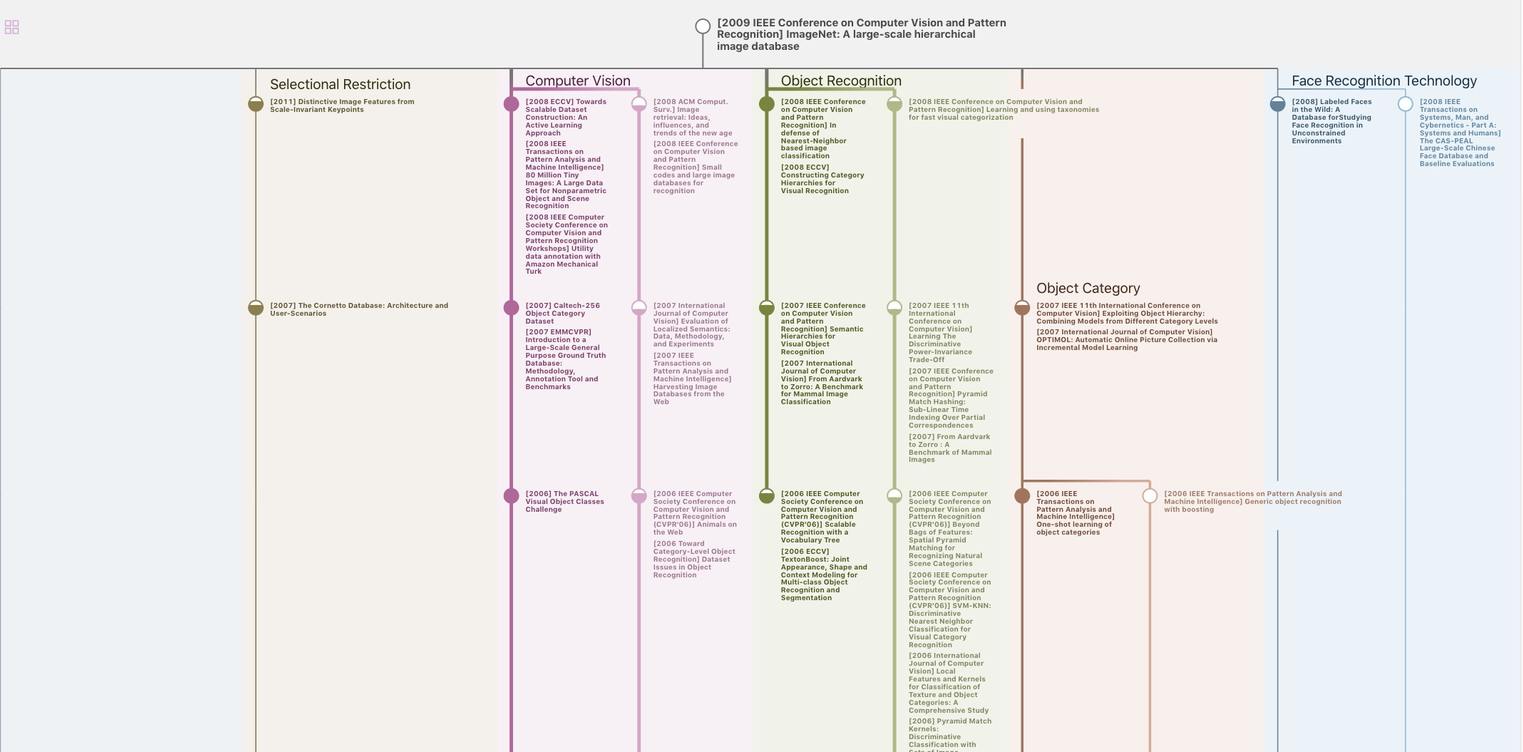
生成溯源树,研究论文发展脉络
Chat Paper
正在生成论文摘要