A novel Pseudo-label based domain adaptation method on tabular data
JOURNAL OF INTELLIGENT & FUZZY SYSTEMS(2023)
摘要
Tabular data is a widely used data form in many fields such as product marketing. In some cases, the domain shift between source and target domain of tabular data may occur with the changing of collection conditions such as time. The extant methods on tabular data mainly consist of neural-network-based methods and tree-based methods. They both meet challenges induced by domain shift on tabular data. First, neural-network-based methods are lack of effective mechanism to extract the features of tabular data and the performance may not be higher than tree-based models. Second, tree-based methods are lack of effective feature representations to model the associations between source domain and target domain. To improve the performance of tree-based methods for domain shift, a novel pseudo-label based domain adaptation method is proposed for the tree-based method called Xgboost. The proposed method consists of pseudo-label generation and selection strategies. The pseudo-label generation strategy can control the effects of pseudo-labels on Xgboost in a more flexible way by setting proper values of pseudo-labels. The pseudo-label selection strategy can select the pseudo-labels with high confidences under a consistency condition based on the outputs of Xgboost. The quality of pseudo-labels for the data in target domain is improved and so does the performance of Xgboost trained by the data in both source domain and target domain. In the experiment, several UCI datasets and 5G terminal datasets are used to showthat the proposed methods can effectively improve the performance of Xgboost.
更多查看译文
关键词
domain adaptation method,tabular data,pseudo-label
AI 理解论文
溯源树
样例
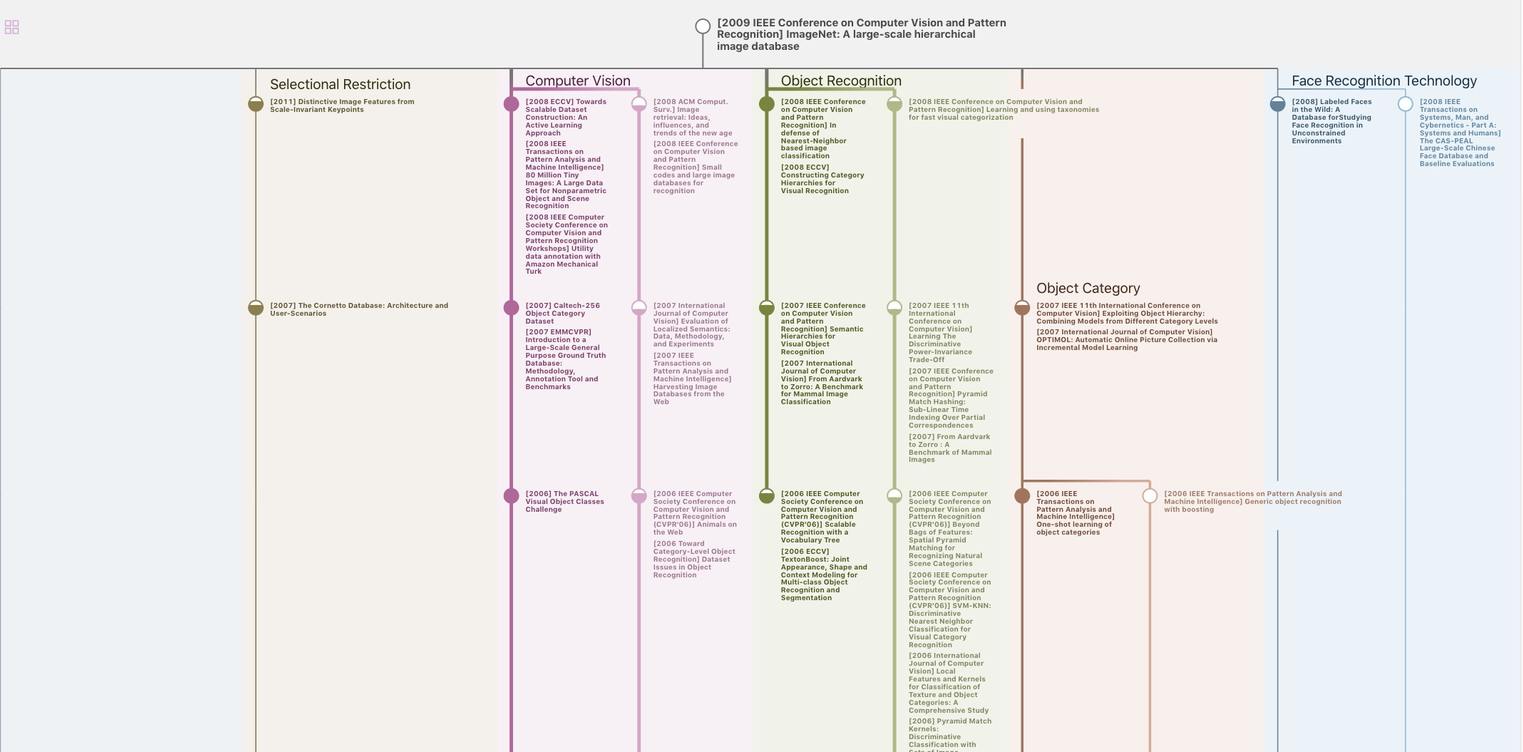
生成溯源树,研究论文发展脉络
Chat Paper
正在生成论文摘要