GraphPLBR: Protein-Ligand Binding Residue Prediction With Deep Graph Convolution Network
IEEE/ACM Transactions on Computational Biology and Bioinformatics(2023)
摘要
The intermolecular interactions between proteins and ligands occur through site-specific amino acid residues in the proteins, and the identification of these key residues plays a critical role in both interpreting protein function and facilitating drug design based on virtual screening. In general, the information about the ligands-binding residues on proteins is unknown, and the detection of the binding residues by the biological wet experiments is time consuming. Therefore, many computational methods have been developed to identify the protein-ligand binding residues in recent years. We propose GraphPLBR, a framework based on Graph Convolutional Neural (GCN) networks, to predict protein-ligand binding residues (PLBR). The proteins are represented as a graph with residues as nodes through 3D protein structure data, such that the PLBR prediction task is transformed into a graph node classification task. A deep graph convolutional network is applied to extract information from higher-order neighbors, and initial residue connection with identity mapping is applied to cope with the over-smoothing problem caused by increasing the number of graph convolutional layers. To the best of our knowledge, this is a more unique and innovative perspective that utilizes the idea of graph node classification for protein-ligand binding residues prediction. By comparing with some state-of-the-art methods, our method performs better on several metrics.
更多查看译文
关键词
Binding residues, deep learning, graph convolutional networks, protein-ligand interaction
AI 理解论文
溯源树
样例
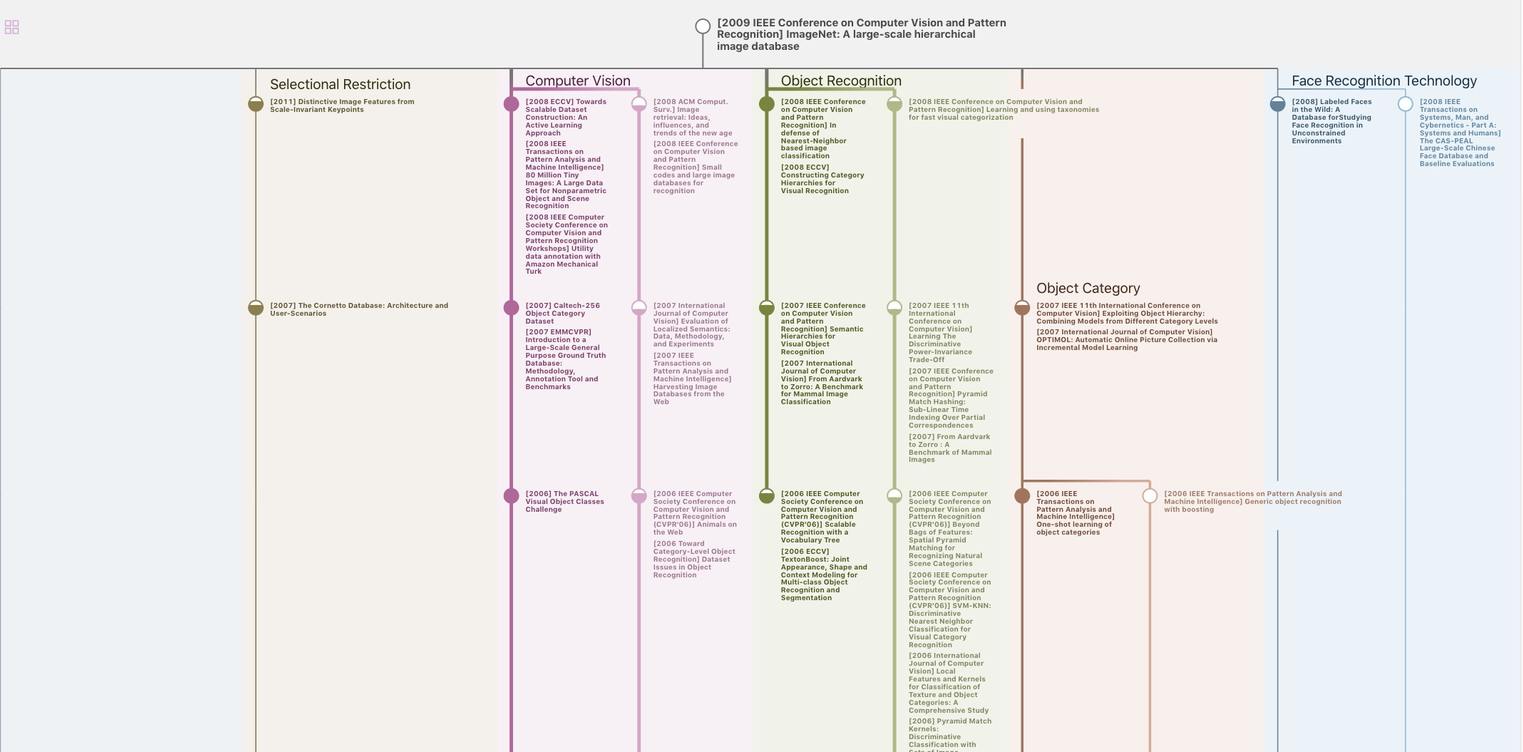
生成溯源树,研究论文发展脉络
Chat Paper
正在生成论文摘要