Machine Learning Reveals Features of Spinon Fermi Surface
Communications Physics(2024)
摘要
With rapid progress in simulation of strongly interacting quantumHamiltonians, the challenge in characterizing unknown phases becomes abottleneck for scientific progress. We demonstrate that a Quantum-Classicalhybrid approach (QuCl) of mining sampled projective snapshots withinterpretable classical machine learning can unveil signatures of seeminglyfeatureless quantum states. The Kitaev-Heisenberg model on a honeycomb latticeunder external magnetic field presents an ideal system to test QuCl, wheresimulations have found an intermediate gapless phase (IGP) sandwiched betweenknown phases, launching a debate over its elusive nature. We use the correlatorconvolutional neural network, trained on labeled projective snapshots, inconjunction with regularization path analysis to identify signatures of phases.We show that QuCl reproduces known features of established phases.Significantly, we also identify a signature of the IGP in the spin channelperpendicular to the field direction, which we interpret as a signature ofFriedel oscillations of gapless spinons forming a Fermi surface. Ourpredictions can guide future experimental searches for spin liquids.
更多查看译文
关键词
Quantum Phase Transitions,Quantum Spin Liquids
AI 理解论文
溯源树
样例
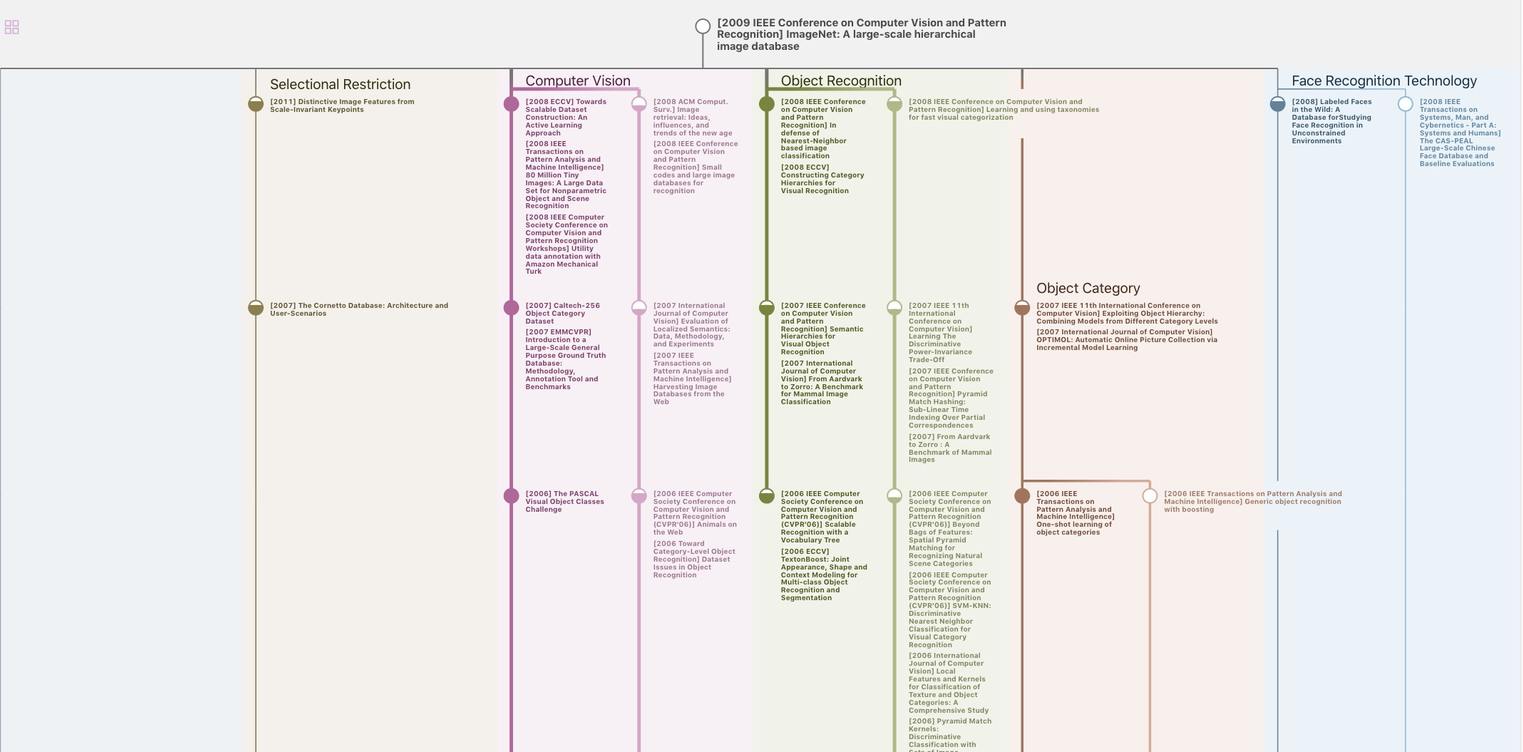
生成溯源树,研究论文发展脉络
Chat Paper
正在生成论文摘要