Towards Fairness in Personalized Ads Using Impression Variance Aware Reinforcement Learning
PROCEEDINGS OF THE 29TH ACM SIGKDD CONFERENCE ON KNOWLEDGE DISCOVERY AND DATA MINING, KDD 2023(2023)
摘要
Variances in ad impression outcomes across demographic groups are increasingly considered to be potentially indicative of algorithmic bias in personalized ads systems. While there are many definitions of fairness that could be applicable in the context of personalized systems, we present a framework which we call the Variance Reduction System (VRS) for achieving more equitable outcomes in Meta's ads systems. VRS seeks to achieve a distribution of impressions with respect to selected protected class (PC) attributes that more closely aligns the demographics of an ad's eligible audience (a function of advertiser targeting criteria) with the audience who sees that ad, in a privacy-preserving manner. We first define metrics to quantify fairness gaps in terms of ad impression variances with respect to PC attributes including gender and estimated race. We then present the VRS for re-ranking ads in an impression variance-aware manner. We evaluate VRS via extensive simulations over different parameter choices and study the effect of the VRS on the chosen fairness metric. We finally present online A/B testing results from applying VRS to Meta's ads systems, concluding with a discussion of future work. We have deployed the VRS to all users in the US for housing ads, resulting in significant improvement in our fairness metric. VRS is the first large-scale deployed framework for pursuing fairness for multiple PC attributes in online advertising.
更多查看译文
关键词
fairness,differential privacy,reinforcement learning,ads
AI 理解论文
溯源树
样例
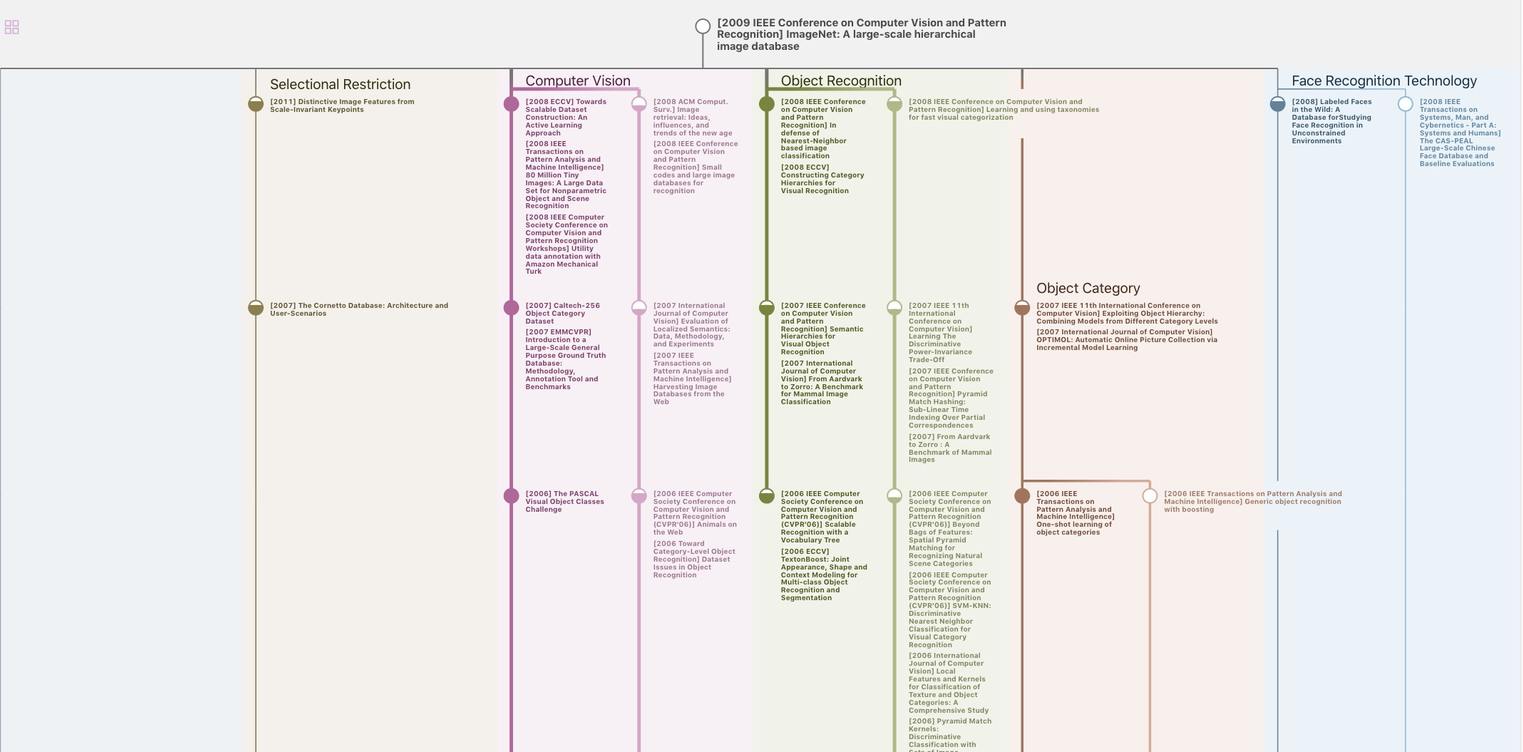
生成溯源树,研究论文发展脉络
Chat Paper
正在生成论文摘要