Recognize Anything: A Strong Image Tagging Model
CoRR(2023)
摘要
We present the Recognize Anything Model (RAM): a strong foundation model for image tagging. RAM can recognize any common category with high accuracy. RAM introduces a new paradigm for image tagging, leveraging large-scale image-text pairs for training instead of manual annotations. The development of RAM comprises four key steps. Firstly, annotation-free image tags are obtained at scale through automatic text semantic parsing. Subsequently, a preliminary model is trained for automatic annotation by unifying the caption and tagging tasks, supervised by the original texts and parsed tags, respectively. Thirdly, a data engine is employed to generate additional annotations and clean incorrect ones. Lastly, the model is retrained with the processed data and fine-tuned using a smaller but higher-quality dataset. We evaluate the tagging capabilities of RAM on numerous benchmarks and observe impressive zero-shot performance, significantly outperforming CLIP and BLIP. Remarkably, RAM even surpasses the fully supervised manners and exhibits competitive performance with the Google API. We are releasing the RAM at \url{https://recognize-anything.github.io/} to foster the advancements of large models in computer vision.
更多查看译文
关键词
strong image tagging model
AI 理解论文
溯源树
样例
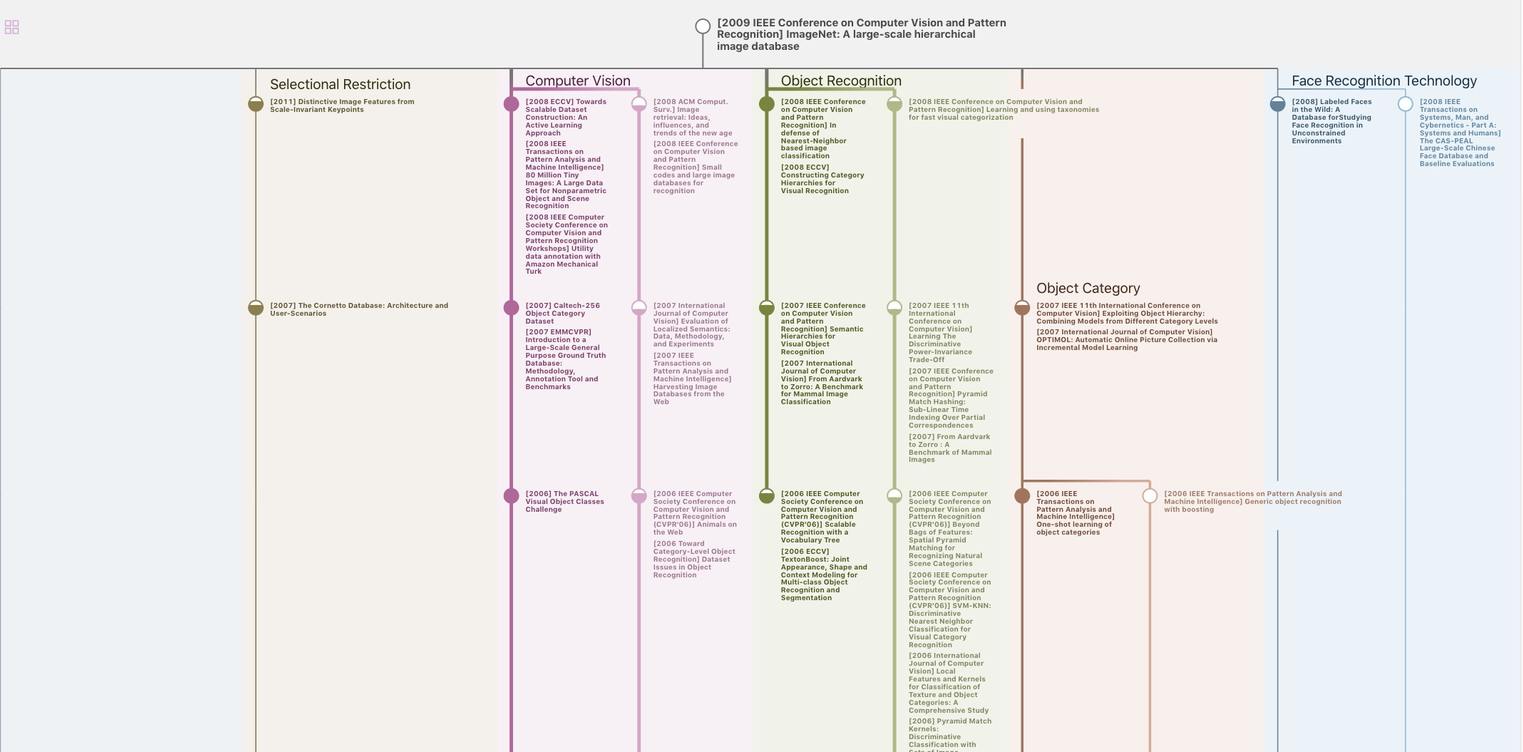
生成溯源树,研究论文发展脉络
Chat Paper
正在生成论文摘要