Effective Intrusion Detection in Highly Imbalanced IoT Networks with Lightweight S2CGAN-IDS
CoRR(2023)
摘要
Since the advent of the Internet of Things (IoT), exchanging vast amounts of information has increased the number of security threats in networks. As a result, intrusion detection based on deep learning (DL) has been developed to achieve high throughput and high precision. Unlike general deep learning-based scenarios, IoT networks contain benign traffic far more than abnormal traffic, with some rare attacks. However, most existing studies have been focused on sacrificing the detection rate of the majority class in order to improve the detection rate of the minority class in class-imbalanced IoT networks. Although this way can reduce the false negative rate of minority classes, it both wastes resources and reduces the credibility of the intrusion detection systems. To address this issue, we propose a lightweight framework named S2CGAN-IDS. The proposed framework leverages the distribution characteristics of network traffic to expand the number of minority categories in both data space and feature space, resulting in a substantial increase in the detection rate of minority categories while simultaneously ensuring the detection precision of majority categories. To reduce the impact of sparsity on the experiments, the CICIDS2017 numeric dataset is utilized to demonstrate the effectiveness of the proposed method. The experimental results indicate that our proposed approach outperforms the superior method in both Precision and Recall, particularly with a 10.2% improvement in the F1-score.
更多查看译文
关键词
Deep learning,class imbalance,intrusion detection,generative adversarial networks,internet of things
AI 理解论文
溯源树
样例
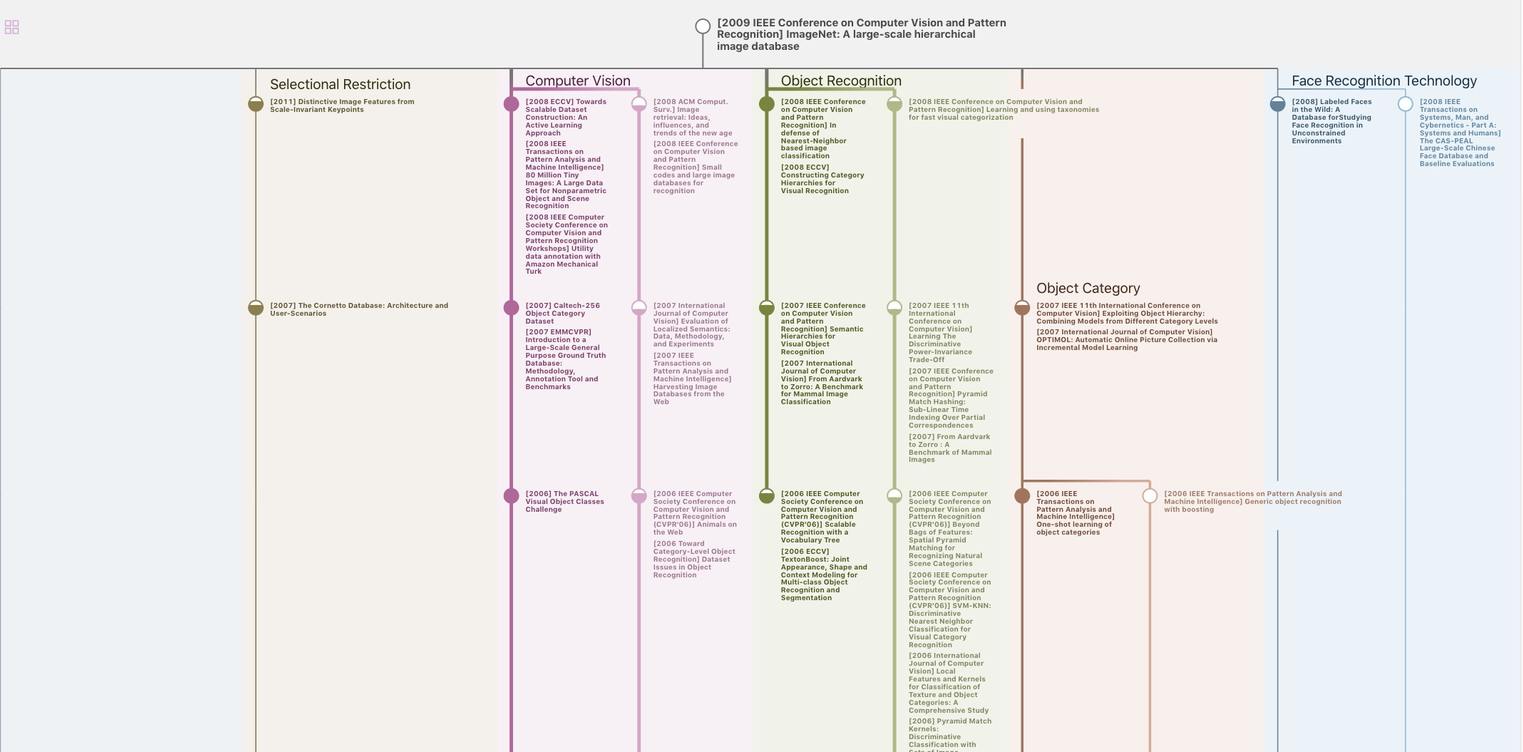
生成溯源树,研究论文发展脉络
Chat Paper
正在生成论文摘要