Tensor network algorithm for simulating experimental Gaussian boson sampling
arXiv (Cornell University)(2023)
摘要
Gaussian boson sampling is a promising candidate for showing experimental quantum advantage. While there is evidence that noiseless Gaussian boson sampling is hard to efficiently simulate using a classical computer, the current Gaussian boson sampling experiments inevitably suffer from loss and other noise models. Despite a high photon loss rate and the presence of noise, they are currently claimed to be hard to classically simulate with the best-known classical algorithm. In this work, we present a classical tensor-network algorithm that simulates Gaussian boson sampling and whose complexity can be significantly reduced when the photon loss rate is high. By generalizing the existing thermal-state approximation algorithm of lossy Gaussian boson sampling, the proposed algorithm enables us to achieve increased accuracy as the running time of the algorithm scales, as opposed to the algorithm that samples from the thermal state, which can give only a fixed accuracy. The generalization allows us to assess the computational power of current lossy experiments even though their output state is not believed to be close to a thermal state. We then simulate the largest Gaussian boson sampling implemented in experiments so far. Much like the actual experiments, classically verifying this large-scale simulation is challenging. To do this, we first observe that in our smaller-scale simulations the total variation distance, cross-entropy, and two-point correlation benchmarks all coincide. Based on this observation, we demonstrate for large-scale experiments that our sampler matches the ground-truth two-point and higher-order correlation functions better than the experiment does, exhibiting evidence that our sampler can simulate the ground-truth distribution better than the experiment can.
更多查看译文
关键词
experimental gaussian boson
AI 理解论文
溯源树
样例
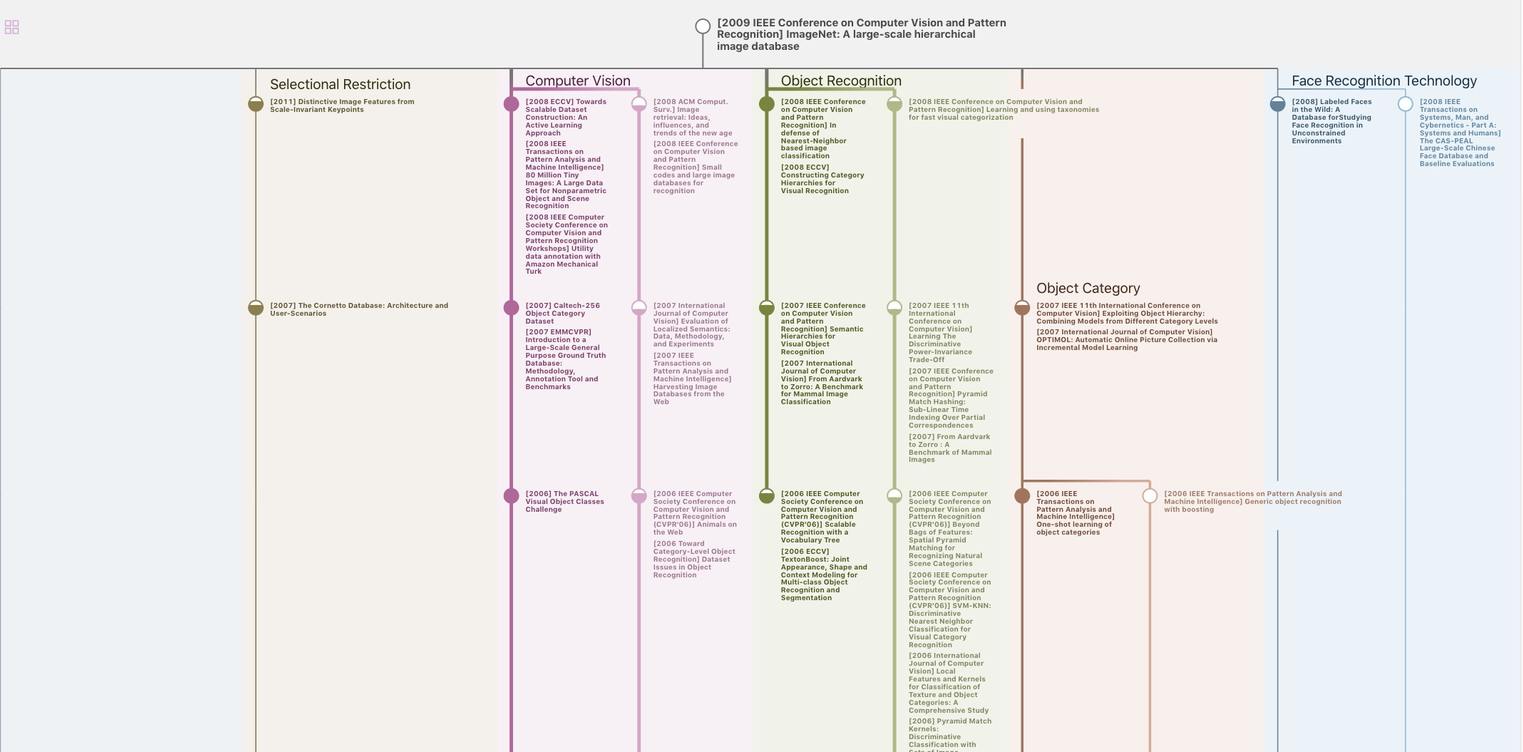
生成溯源树,研究论文发展脉络
Chat Paper
正在生成论文摘要