Rel-CNN: Learning Relationship Features in Time Series for Classification
IEEE Transactions on Knowledge and Data Engineering(2023)
摘要
Time series classification has ubiquitous applications in the real world. Owing to its importance, many time series classification techniques have been proposed over years. Among them, neural network based methods have attracted significant research attention due to their ability to automatically extract latent and discriminative features from data. In this paper, we explore relationship features, which provide valuable global information for time series analytics, and propose a general neural network architecture, namely Rel-CNN, to learn both global and local subsequence features for time series classification. Moreover, we provide two detailed model designs, Relationship Feature based Convolution Filtering and Latent Relationship Feature based Convolution Filtering, and address technical issues due to excessive parameters to learn in these models. We evaluate our models and baselines on time series classification, with extensive experiments on the widely-used 85 uni-variate "bake-off" datasets and 8 multi-variate UEA datasets. Experimental results show that our Rel-CNN models are superior to the representative time series classifiers, in terms of average accuracy, average Macro-f1 and ranking metrics. In addition, an ensemble version of Rel-CNN also outperforms the state-of-the-art ensemble classifiers in terms of average rank, average accuracy and average Macro-f1 on the bake-off datasets.
更多查看译文
关键词
Neural networks,time series classification,relationship features
AI 理解论文
溯源树
样例
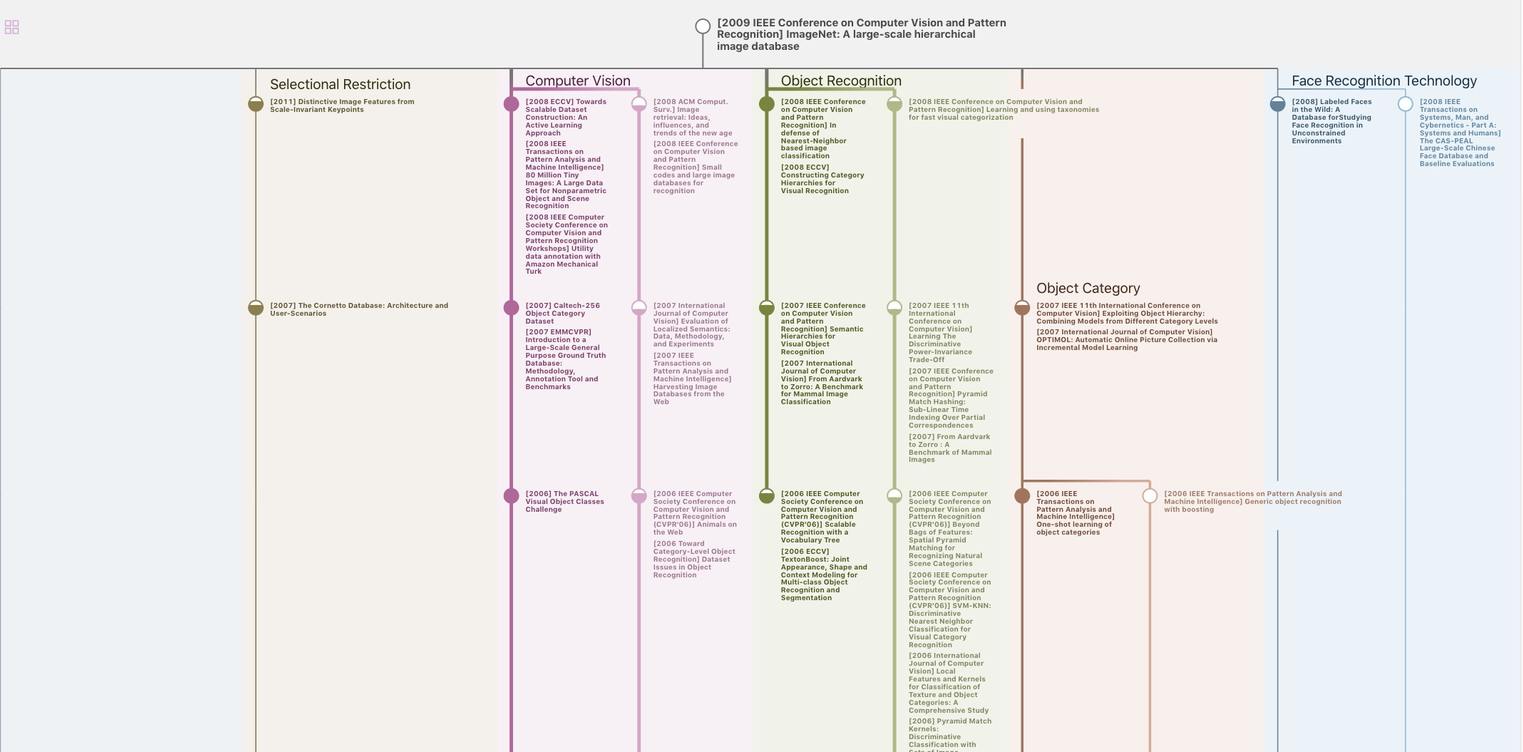
生成溯源树,研究论文发展脉络
Chat Paper
正在生成论文摘要