GPT Self-Supervision for a Better Data Annotator.
CoRR(2023)
摘要
The task of annotating data into concise summaries poses a significant challenge across various domains, frequently requiring the allocation of significant time and specialized knowledge by human experts. Despite existing efforts to use large language models for annotation tasks, significant problems such as limited applicability to unlabeled data, the absence of self-supervised methods, and the lack of focus on complex structured data still persist. In this work, we propose a GPT self-supervision annotation method. This method embodies a generating-recovering paradigm that leverages the capabilities of one-shot learning capabilities in Generative Pretrained Transformer (GPT). The proposed approach comprises a one-shot tuning phase followed by a generation phase. In the one-shot tuning phase, we sample a data from the support set as part of the prompt for GPT to generate a textual summary, which is then used to recover the original data. The alignment score between the recovered and original data serves as a self-supervision navigator to refine the process. In the generation stage, the optimally selected one-shot sample serves as a template in the prompt and is applied to generating summaries from challenging datasets. The annotation performance is evaluated by tuning several human feedback reward networks and by calculating alignment scores between original and recovered data at both sentence and structure levels. Our self-supervised annotation method consistently achieves competitive scores, convincingly demonstrating its robust strength in various data-to-summary annotation tasks.
更多查看译文
关键词
data,self-supervision
AI 理解论文
溯源树
样例
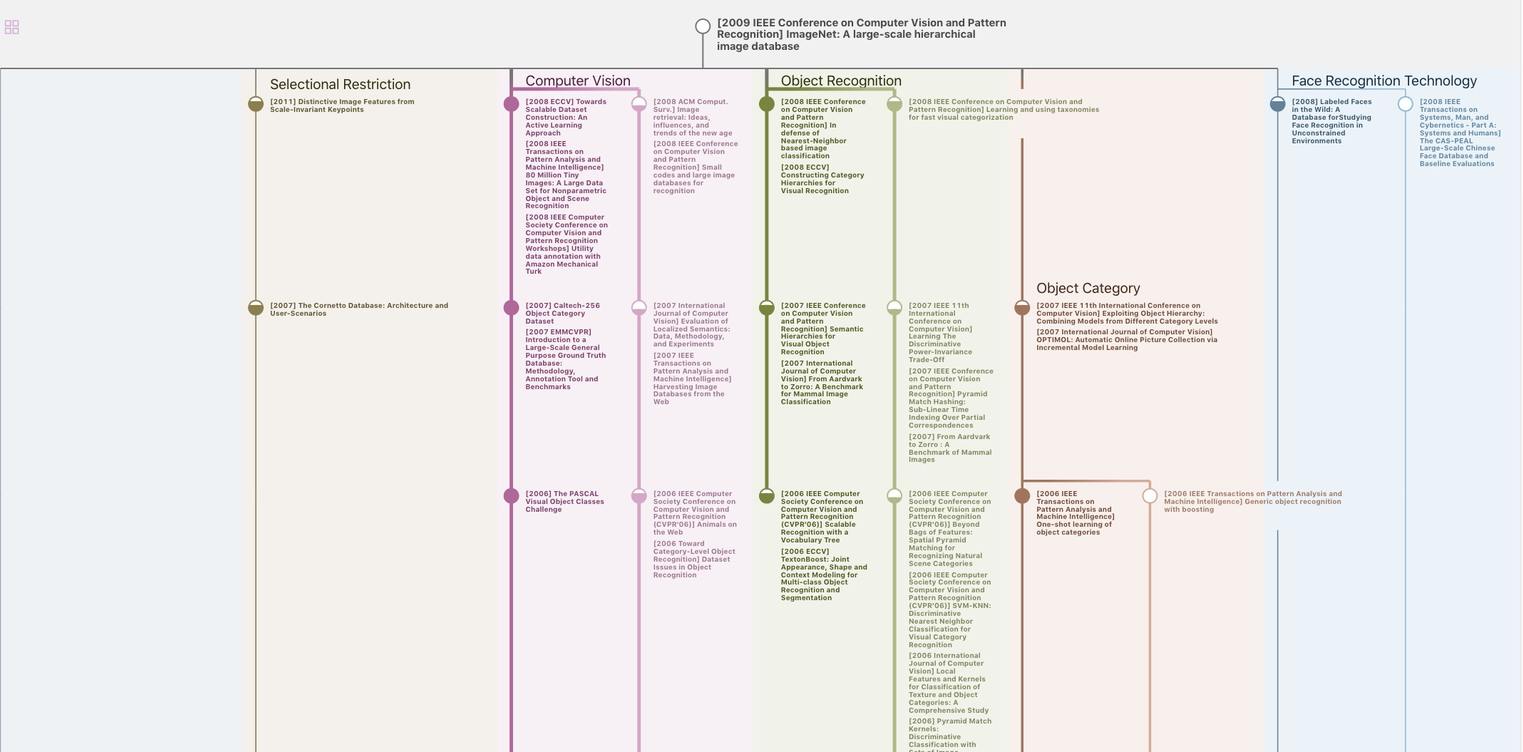
生成溯源树,研究论文发展脉络
Chat Paper
正在生成论文摘要