Spatial regression of multi-fidelity meteorological observations using a proxy-based measurement error model
Advances in Science and Research(2023)
摘要
High-resolution weather maps are fundamental components of early warning systems, since they enable the (near) real-time tracking of extreme weather events. In this context, crowd-sourced weather networks producing low-fidelity observations are often the only type of data available at local (e.g. neighborhood) scales. In this work, we demonstrate that we can provide such maps by combining high-fidelity official weather data with low-fidelity crowd-sourced weather data and high-resolution covariate information. Because the crowd-sourced data contains significant bias and noise, we develop an approach to include a bias budget and noise budget in the multi-fidelity Bayesian spatial data analysis. The weights of the different components of these bias and noise budgets are tuned to the data set. We apply this approach to 24 hours of weather data in the Netherlands, for a day that had a "code orange" (i.e. "be prepared for extreme weather with high risk of impact") weather warning for heavy precipitation. From our analysis, we see a significant - qualitative and quantitative - synergy effect when introducing low-fidelity data and high-resolution covariate information.
更多查看译文
关键词
spatial regression,measurement error model,observations,multi-fidelity,proxy-based
AI 理解论文
溯源树
样例
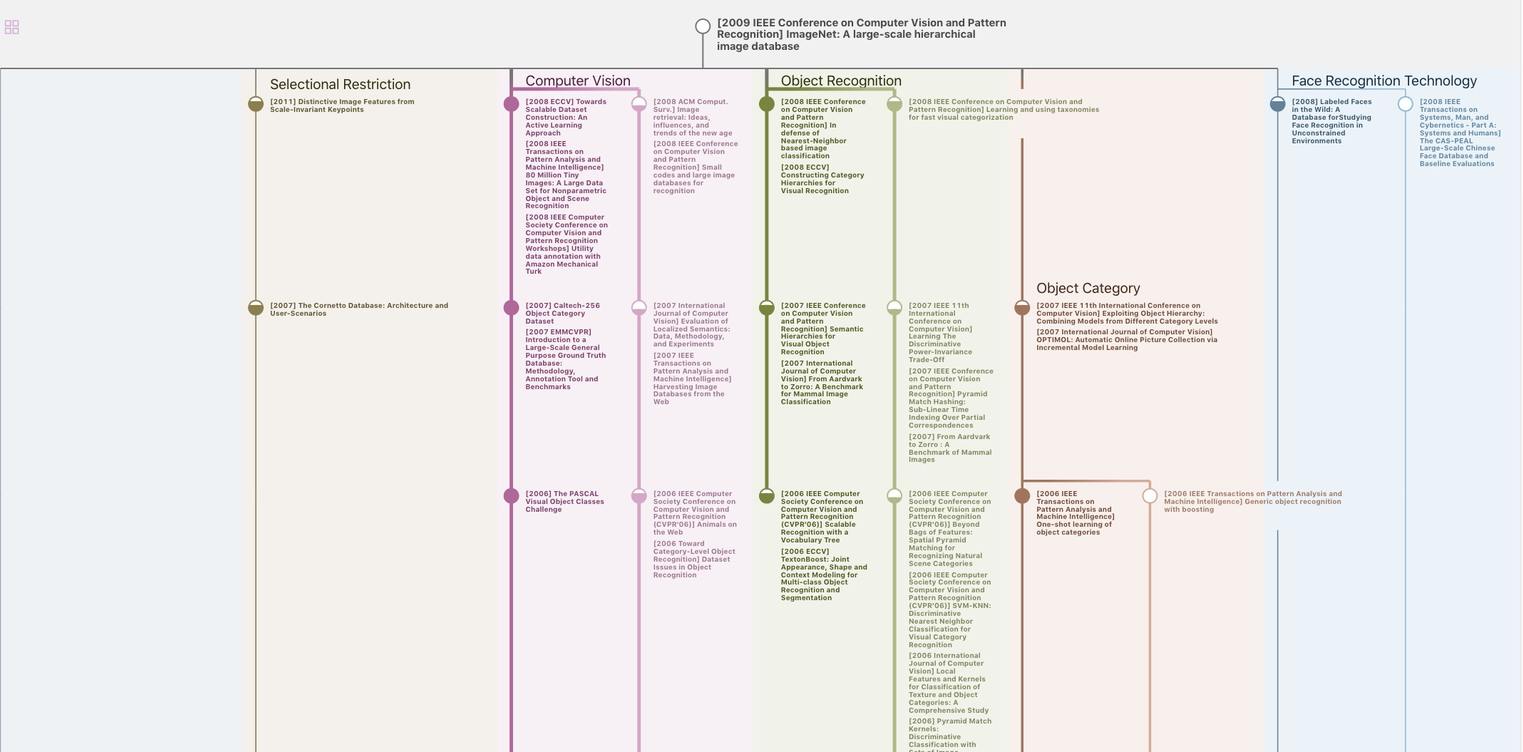
生成溯源树,研究论文发展脉络
Chat Paper
正在生成论文摘要