Learned Spatial Data Partitioning
aiDM@SIGMOD(2023)
摘要
Due to the significant increase in the size of spatial data, it is essential to use distributed parallel processing systems to efficiently analyze spatial data. In this paper, we first study learned spatial data partitioning, which effectively assigns groups of big spatial data to computers based on locations of data by using machine learning techniques. We formalize spatial data partitioning in the context of reinforcement learning and develop a novel deep reinforcement learning algorithm. Our learning algorithm leverages features of spatial data partitioning and prunes ineffective learning processes to find optimal partitions efficiently. Our experimental study, which uses Apache Sedona and real-world spatial data, demonstrates that our method efficiently finds partitions for accelerating distance join queries and reduces the workload run time by up to 59.4%.
更多查看译文
关键词
spatial data
AI 理解论文
溯源树
样例
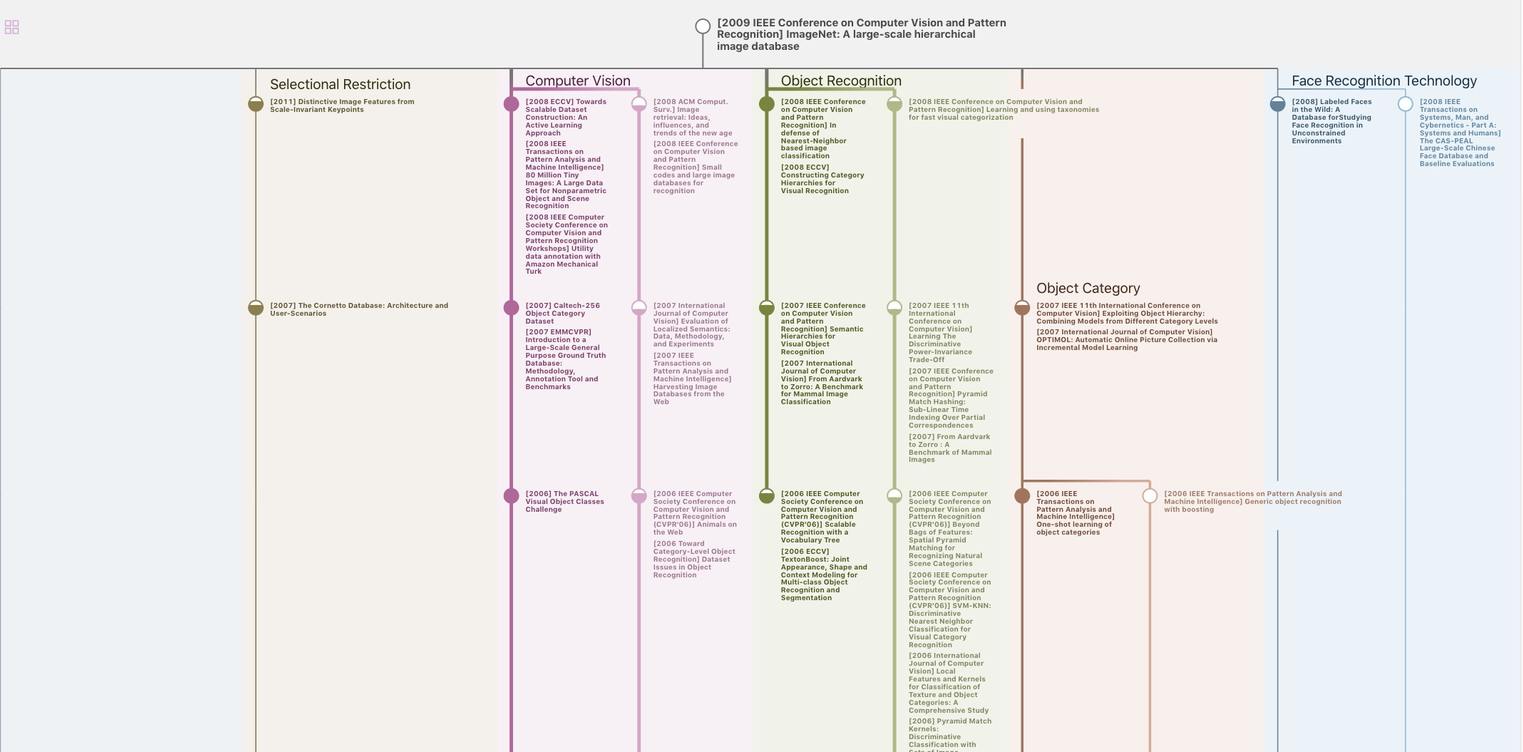
生成溯源树,研究论文发展脉络
Chat Paper
正在生成论文摘要