Magnitude Attention-based Dynamic Pruning
CoRR(2023)
摘要
Existing pruning methods utilize the importance of each weight based on specified criteria only when searching for a sparse structure but do not utilize it during training. In this work, we propose a novel approach - \textbf{M}agnitude \textbf{A}ttention-based Dynamic \textbf{P}runing (MAP) method, which applies the importance of weights throughout both the forward and backward paths to explore sparse model structures dynamically. Magnitude attention is defined based on the magnitude of weights as continuous real-valued numbers enabling a seamless transition from a redundant to an effective sparse network by promoting efficient exploration. Additionally, the attention mechanism ensures more effective updates for important layers within the sparse network. In later stages of training, our approach shifts from exploration to exploitation, exclusively updating the sparse model composed of crucial weights based on the explored structure, resulting in pruned models that not only achieve performance comparable to dense models but also outperform previous pruning methods on CIFAR-10/100 and ImageNet.
更多查看译文
关键词
magnitude,attention-based
AI 理解论文
溯源树
样例
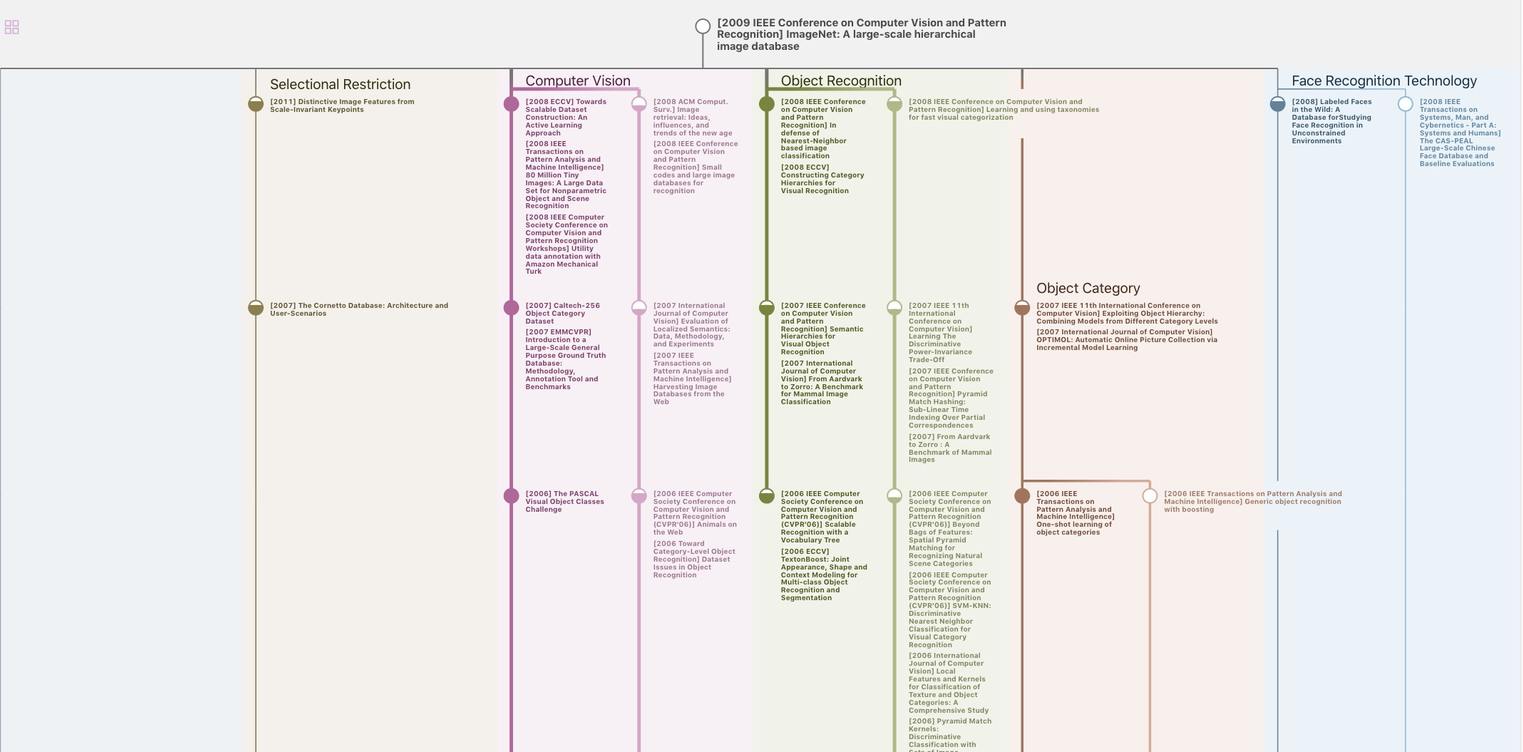
生成溯源树,研究论文发展脉络
Chat Paper
正在生成论文摘要