Multimodal Cascaded Framework with Metric Learning Robust to Missing Modalities for Person Classification
PROCEEDINGS OF THE 2023 PROCEEDINGS OF THE 14TH ACM MULTIMEDIA SYSTEMS CONFERENCE, MMSYS 2023(2023)
摘要
This paper addresses the missing modality problem in multimodal person classification, where an incomplete multimodal input with one modality missing is classified into predefined person classes. A multimodal cascaded framework with three deep learning models is proposed, where model parameters, outputs, and latent space learnt at a given step are transferred to the model in a subsequent step. The cascaded framework addresses the missing modality problem by, firstly, generating the complete multimodal data from the incomplete multimodal data in the feature space via a latent space. Subsequently, the generated and original multimodal features are effectively merged and embedded into a final latent space to estimate the person label. During the learning phase, the cascaded framework uses two novel latent loss functions, the missing modality joint loss, and latent prior loss to learn the different latent spaces. The missing modality joint loss ensures that the similar class latent data are close to each other, even if a modality is missing. In the cascaded framework, the latent prior loss learns the final latent space using a previously learnt latent space as a prior. The proposed framework is validated on the audio-visible RAVDESS and the visible-thermal Speaking Faces datasets. A detailed comparative analysis and an ablation analysis are performed, which demonstrate that the proposed framework enhances the robustness of person classification even under conditions of missing modalities, reporting an average of 21.75% increase and 25.73% increase over the baseline algorithms on the RAVDESS and Speaking Faces datasets.
更多查看译文
关键词
Multimodal Learning,Missing Modality,Metric Learning,Deep Learning,Person Classification
AI 理解论文
溯源树
样例
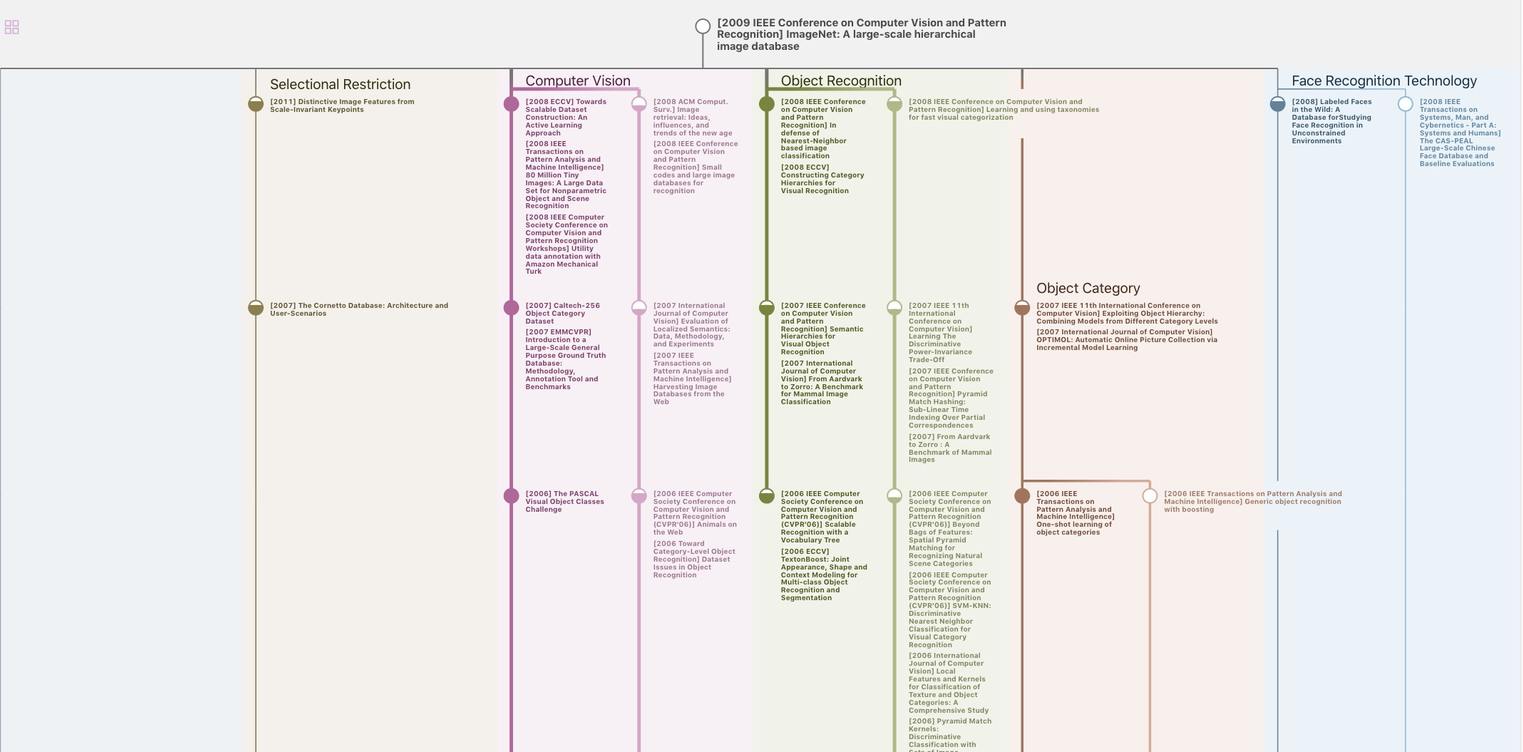
生成溯源树,研究论文发展脉络
Chat Paper
正在生成论文摘要