Trajectory Prediction with Observations of Variable-Length for Motion Planning in Highway Merging scenarios
CoRR(2023)
摘要
Accurate trajectory prediction of nearby vehicles is crucial for the safe motion planning of automated vehicles in dynamic driving scenarios such as highway merging. Existing methods cannot initiate prediction for a vehicle unless observed for a fixed duration of two or more seconds. This prevents a fast reaction by the ego vehicle to vehicles that enter its perception range, thus creating safety concerns. Therefore, this paper proposes a novel transformer-based trajectory prediction approach, specifically trained to handle any observation length larger than one frame. We perform a comprehensive evaluation of the proposed method using two large-scale highway trajectory datasets, namely the highD and exiD. In addition, we study the impact of the proposed prediction approach on motion planning and control tasks using extensive merging scenarios from the exiD dataset. To the best of our knowledge, this marks the first instance where such a large-scale highway merging dataset has been employed for this purpose. The results demonstrate that the prediction model achieves state-of-the-art performance on highD dataset and maintains lower prediction error w.r.t. the constant velocity across all observation lengths in exiD. Moreover, it significantly enhances safety, comfort, and efficiency in dense traffic scenarios, as compared to the constant velocity model.
更多查看译文
关键词
Path Planning,Trajectory Prediction,Merging Scenario,Highway Merging,Prediction Model,Motor Control,Prediction Approach,Constant Velocity,Control Task,Automated Vehicles,Constant Velocity Model,Time Step,Convolutional Neural Network,Long Short-term Memory,Recurrent Neural Network,Autonomous Vehicles,Tracking Data,Model Predictive Control,Planning Algorithm,Vehicle Trajectory,Transformer Decoder,Road Boundary,Velocity Prediction,Longitudinal Velocity,Lane Markings,Reference Path,Learning-based Prediction,Target Vehicle,Vehicle Center
AI 理解论文
溯源树
样例
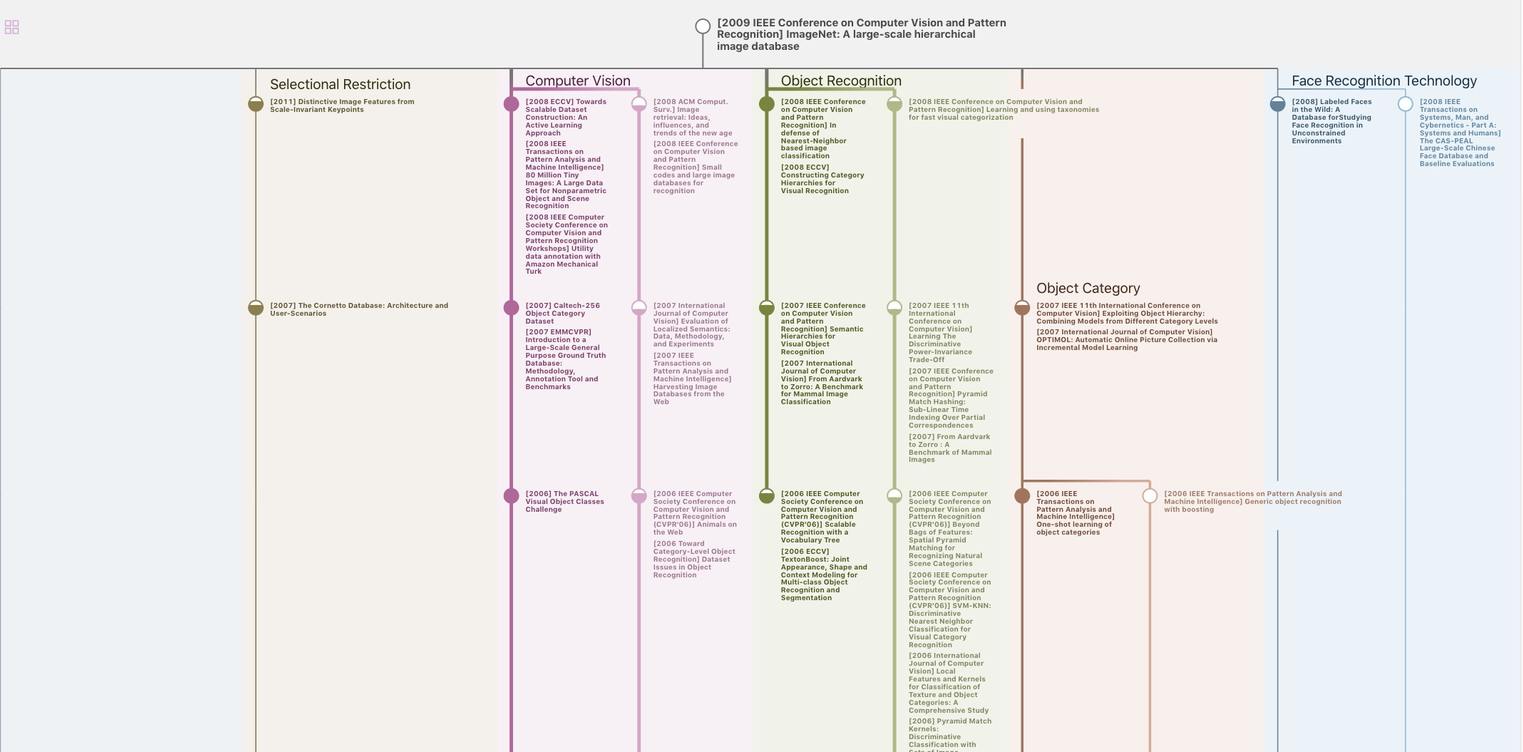
生成溯源树,研究论文发展脉络
Chat Paper
正在生成论文摘要