Improving LiDAR 3D Object Detection via Range-based Point Cloud Density Optimization
CoRR(2023)
摘要
In recent years, much progress has been made in LiDAR-based 3D object detection mainly due to advances in detector architecture designs and availability of large-scale LiDAR datasets. Existing 3D object detectors tend to perform well on the point cloud regions closer to the LiDAR sensor as opposed to on regions that are farther away. In this paper, we investigate this problem from the data perspective instead of detector architecture design. We observe that there is a learning bias in detection models towards the dense objects near the sensor and show that the detection performance can be improved by simply manipulating the input point cloud density at different distance ranges without modifying the detector architecture and without data augmentation. We propose a model-free point cloud density adjustment pre-processing mechanism that uses iterative MCMC optimization to estimate optimal parameters for altering the point density at different distance ranges. We conduct experiments using four state-of-the-art LiDAR 3D object detectors on two public LiDAR datasets, namely Waymo and ONCE. Our results demonstrate that our range-based point cloud density manipulation technique can improve the performance of the existing detectors, which in turn could potentially inspire future detector designs.
更多查看译文
关键词
lidar 3d object detection,point cloud density
AI 理解论文
溯源树
样例
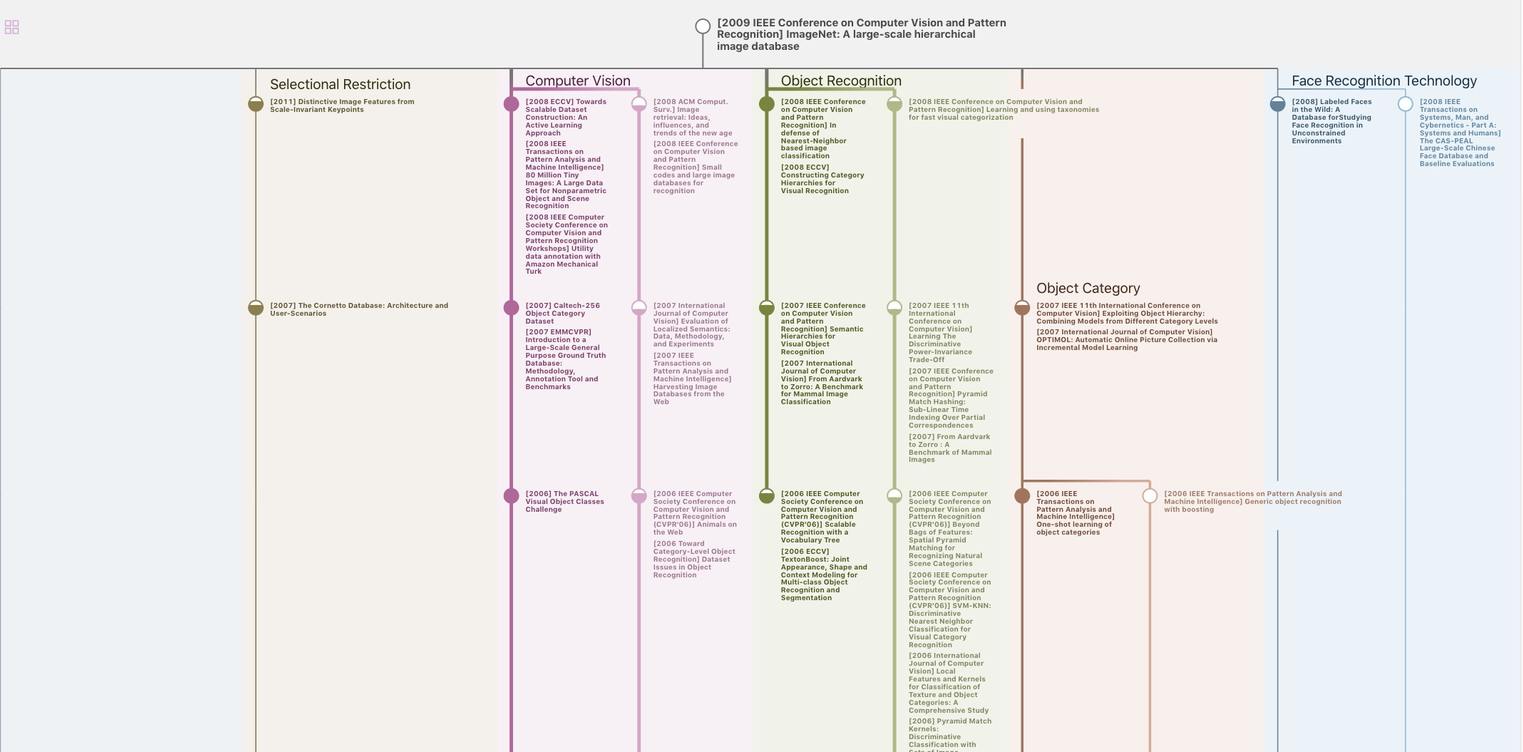
生成溯源树,研究论文发展脉络
Chat Paper
正在生成论文摘要