A reduced-rank approach to predicting multiple binary responses through machine learning
arXiv (Cornell University)(2023)
摘要
This paper investigates the problem of simultaneously predicting multiple binary responses by utilizing a shared set of covariates. Our approach incorporates machine learning techniques for binary classification, without making assumptions about the underlying observations. Instead, our focus lies on a group of predictors, aiming to identify the one that minimizes prediction error. Unlike previous studies that primarily address estimation error, we directly analyze the prediction error of our method using PAC-Bayesian bounds techniques. In this paper, we introduce a pseudo-Bayesian approach capable of handling incomplete response data. Our strategy is efficiently implemented using the Langevin Monte Carlo method. Through simulation studies and a practical application using real data, we demonstrate the effectiveness of our proposed method, producing comparable or sometimes superior results compared to the current state-of-the-art method.
更多查看译文
关键词
multiple binary responses,machine learning,reduced-rank
AI 理解论文
溯源树
样例
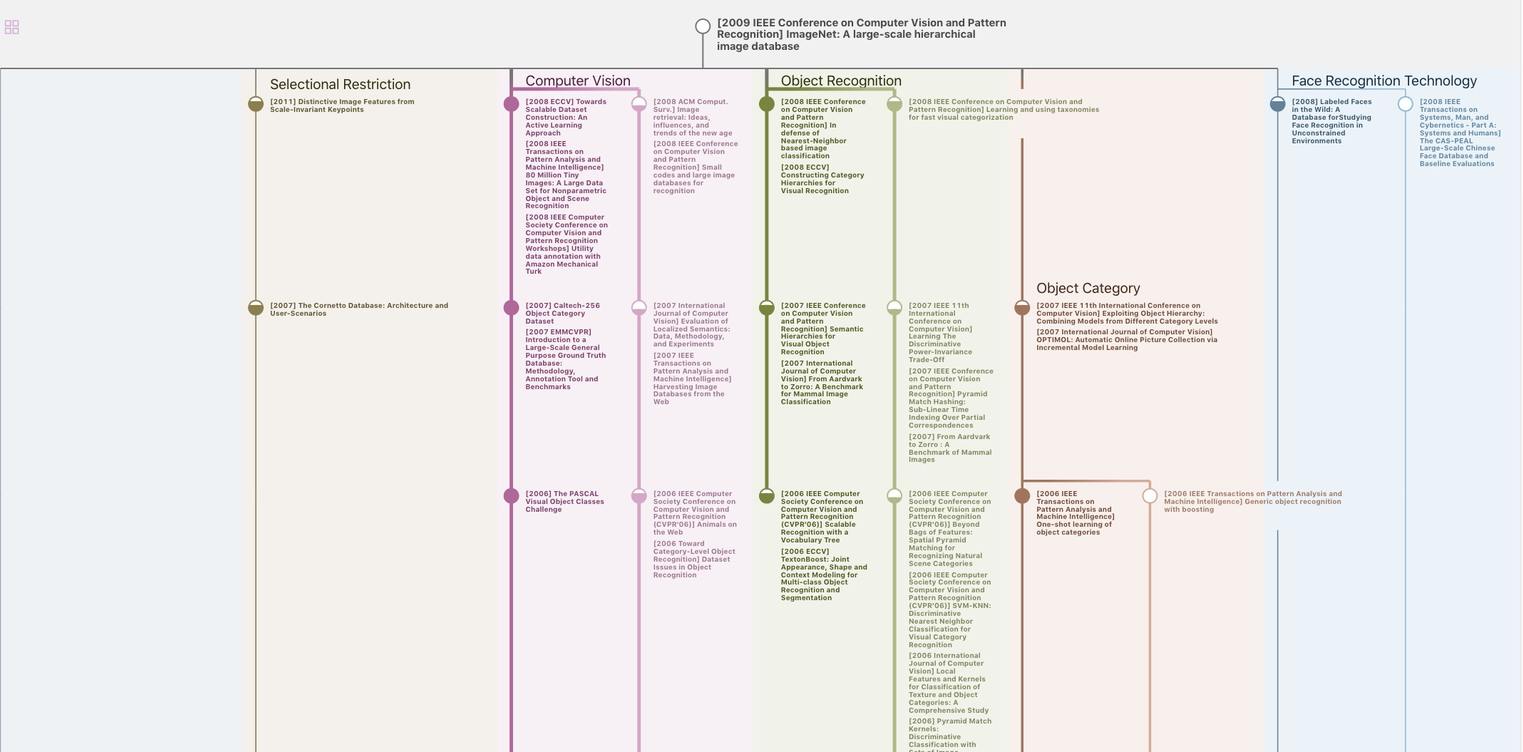
生成溯源树,研究论文发展脉络
Chat Paper
正在生成论文摘要