Knowledge-enhanced prototypical network with class cluster loss for few-shot relation classification
PLoS ONE(2023)
摘要
Few-shot Relation Classification identifies the relation between target entity pairs in unstructured natural language texts by training on a small number of labeled samples. Recent prototype network-based studies have focused on enhancing the prototype representation capability of models by incorporating external knowledge. However, the majority of these works constrain the representation of class prototypes implicitly through complex network structures, such as multi-attention mechanisms, graph neural networks, and contrastive learning, which constrict the model's ability to generalize. In addition, most models with triplet loss disregard intra-class compactness during model training, thereby limiting the model's ability to handle outlier samples with low semantic similarity. Therefore, this paper proposes a non-weighted prototype enhancement module that uses the feature-level similarity between prototypes and relation information as a gate to filter and complete features. Meanwhile, we design a class cluster loss that samples difficult positive and negative samples and explicitly constrains both intra-class compactness and inter-class separability to learn a metric space with high discriminability. Extensive experiments were done on the publicly available dataset FewRel 1.0 and 2.0, and the results show the effectiveness of the proposed model.
更多查看译文
关键词
prototypical network,relation,class cluster loss,classification,knowledge-enhanced,few-shot
AI 理解论文
溯源树
样例
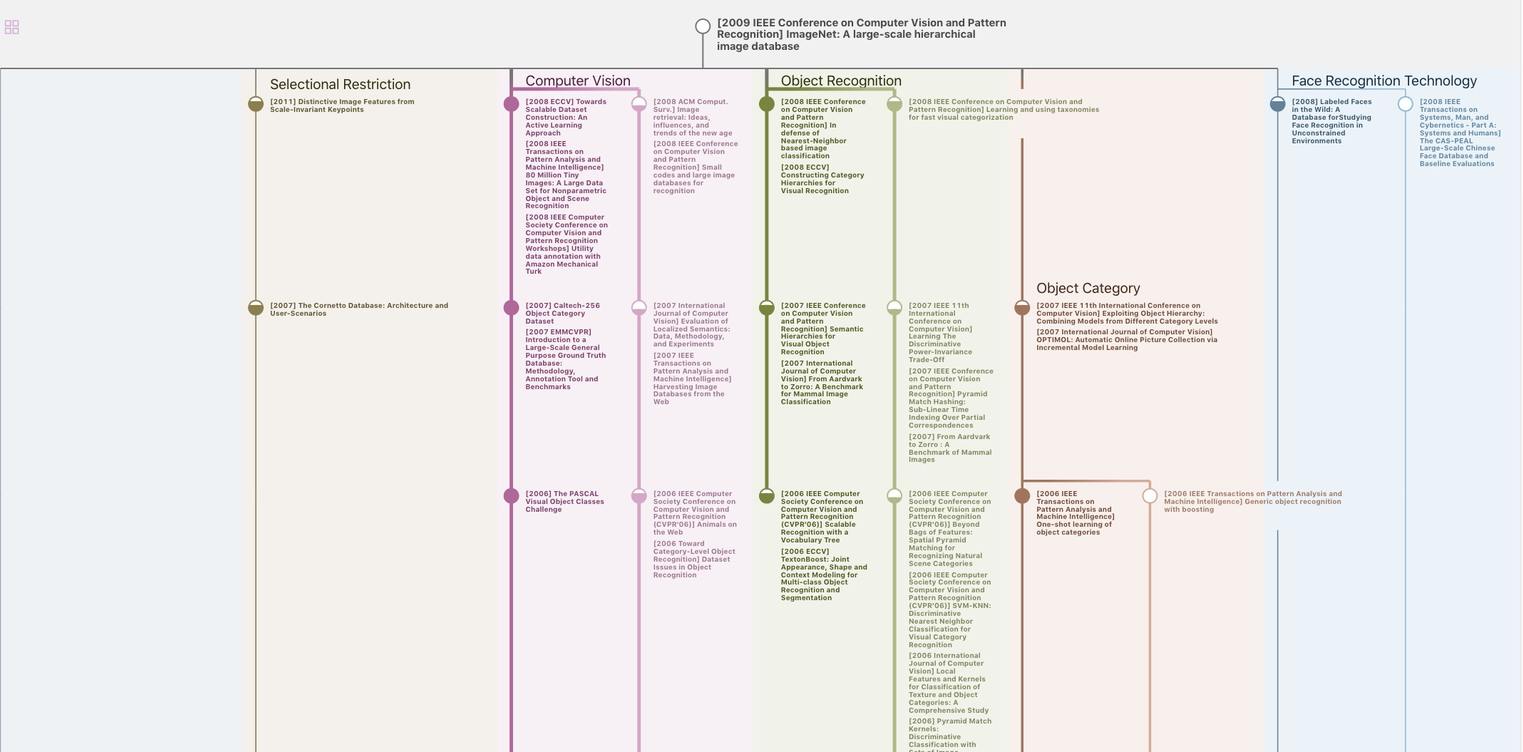
生成溯源树,研究论文发展脉络
Chat Paper
正在生成论文摘要