Toward Next-Generation Signal Intelligence: A Hybrid Knowledge and Data-Driven Deep Learning Framework for Radio Signal Classification
IEEE Transactions on Cognitive Communications and Networking(2023)
摘要
Automatic modulation classification (AMC) can generally be divided into knowledge-based methods and data-driven methods. In this paper, we explore combining the knowledge-based method and data-driven technology to take full advantage of both and propose a hybrid knowledge and data-driven deep learning framework (HKDD) for AMC. To make the handcrafted features more discriminative, various traditional features are adopted, including instantaneous features, statistical features, and spectral features. In the HKDD framework, a feature fusion mechanism is proposed to integrate the features learned from the original signal with those processed by a fully connected network from the handcrafted features. Besides, an attention mechanism is implemented on the fused features to neglect immature features and highlight important features. To evaluate the performance of the proposed method, we construct two modulation classification datasets containing both traditional features and raw IQ data. The bigger one contains 36 modulation categories, which is greater than the number of categories of any AMC dataset currently available. Simulation results show that our proposed method has significant performance gain in both adequate-sample classification scenario and few-shot classification scenario.
更多查看译文
关键词
radio,deep learning framework,deep learning,signal,next-generation,data-driven
AI 理解论文
溯源树
样例
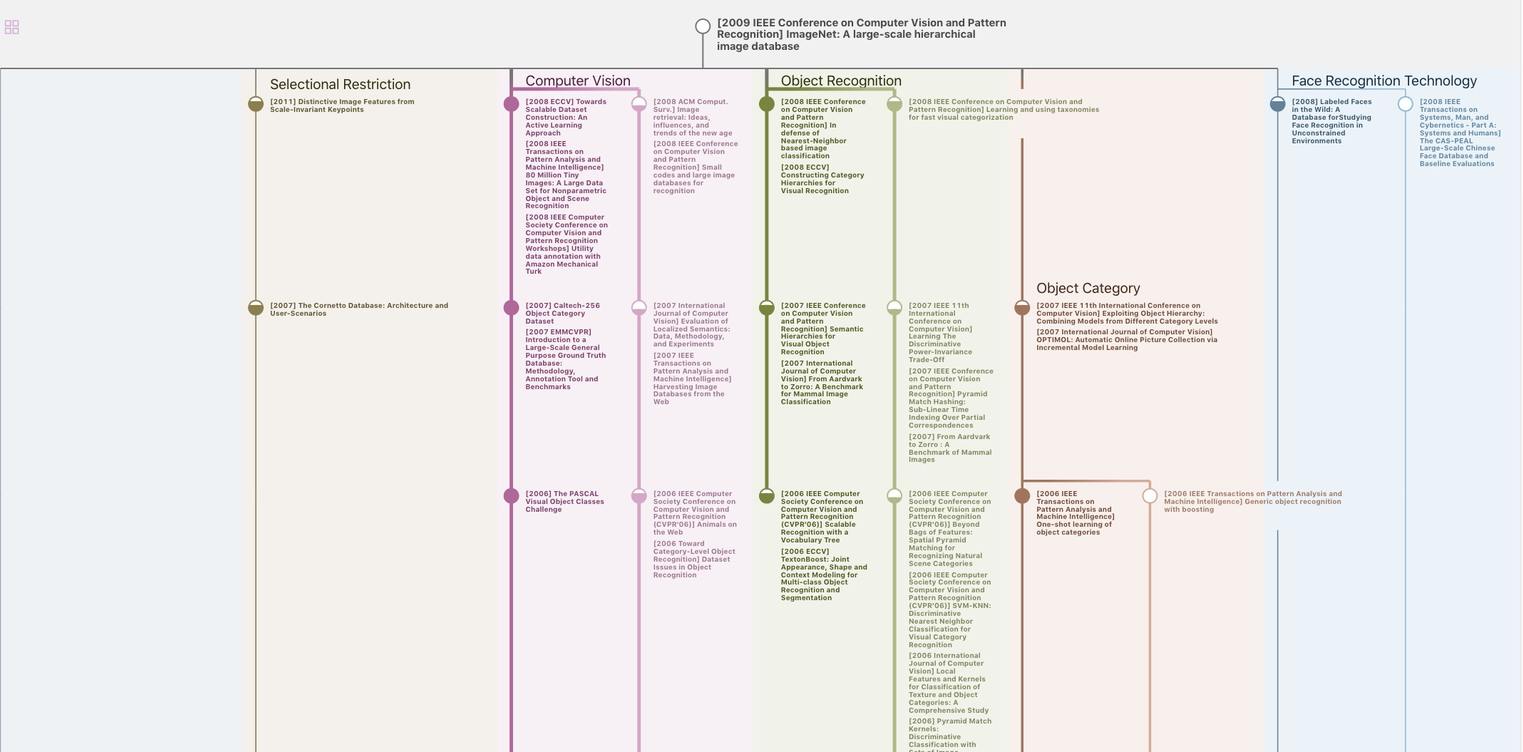
生成溯源树,研究论文发展脉络
Chat Paper
正在生成论文摘要