A Deep Learning Approach for Transgender and Gender Diverse Patient Identification in Electronic Health Records
medRxiv (Cold Spring Harbor Laboratory)(2023)
摘要
ABSTRACT Background Although accurate identification of gender identity in the electronic health record (EHR) is crucial for providing equitable health care, particularly for transgender and gender diverse (TGD) populations, it remains a challenging task due to incomplete gender information in structured EHR fields. Objective To develop a deep learning classifier to accurately identify patient gender identity using patient-level EHR data, including free-text notes. Methods This study included adult patients in a large healthcare system in Boston, MA, between 4/1/2017 to 4/1/2022. To identify relevant information from massive clinical notes and to denoise, we compiled a list of gender-related keywords through expert curation, literature review, and expansion via a fine-tuned BioWordVec model. This keyword list was used to pre-screen potential TGD individuals and create two datasets for model training, testing, and validation. Dataset I was a balanced dataset that contained clinician-confirmed TGD patients and cases without keywords. Dataset II contained cases with keywords. The performance of the deep learning model was compared to traditional machine learning and rule-based algorithms. Results The final keyword list consists of 109 keywords, of which 58 (53.2%) were expanded by the BioWordVec model. Dataset I contained 3,150 patients (50% TGD) while Dataset II contained 200 patients (90% TGD). On Dataset I the deep learning model achieved a F1 score of 0.917, sensitivity of 0.854, and a precision of 0.980; and on Dataset II a F1 score of 0.969, sensitivity of 0.967, and precision of 0.972. The deep learning model significantly outperformed rule-based algorithms. Conclusion This is the first study to show that deep learning algorithms can accurately identify gender identity using EHR data. Future work should leverage and evaluate additional diverse data sources to generate more generalizable algorithms. Graphical abstract
更多查看译文
关键词
Gender identity,Transgender persons,Sexual and gender minorities,Electronic health records,Machine learning,Natural language processing,BERT,HER,MGB,NLP,TGD,SVM,TF-IDF
AI 理解论文
溯源树
样例
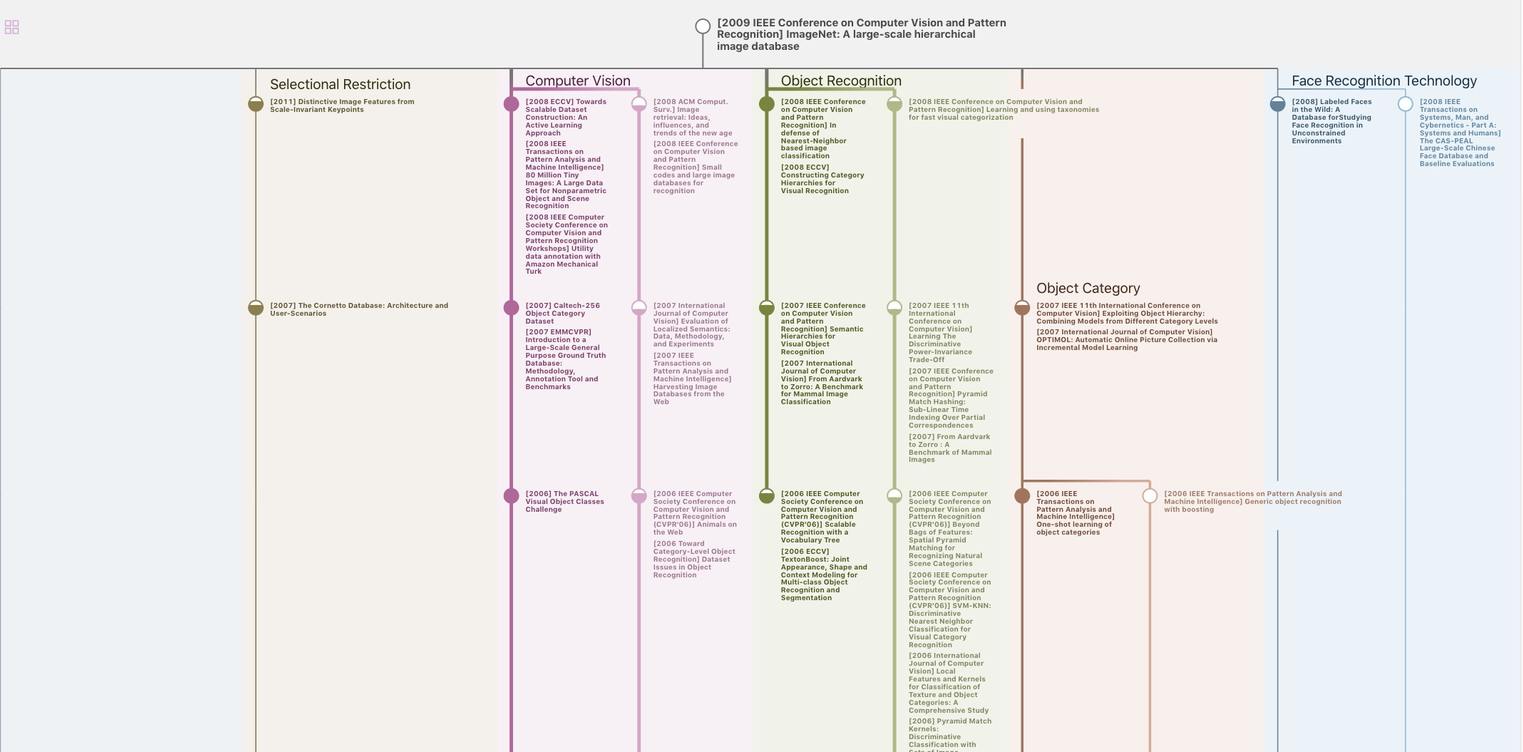
生成溯源树,研究论文发展脉络
Chat Paper
正在生成论文摘要