Multimodal camera for pedestrian detection with deep learning models
2023 IEEE International Conference on Industrial Technology (ICIT)(2023)
摘要
Road deaths are still alarmingly high. It also ranks first regarding causes of death unrelated to pre-existing health status. More than half of all road traffic deaths occur among vulnerable road users like pedestrians. The main purpose of the work was to design, implement, and evaluate pedestrian detection methods using multimodal imaging and machine learning meth-ods. Two cameras were co-calibrated using a specially designed reference chessboard. The method of superimposing multimodal images using thermal and RGB data was proposed to produce the final images. New datasets consisting of 8000 multimodal images were collected in good and limited visibility. The adapted YOLO models, which included edge device variants for TFLite, were proposed to detect pedestrians in a new representation of multimodal data. The models were trained to optimize the detection accuracy and speed performance for mobile devices compared to desktop computers in different lighting conditions. The best results obtained in tests for a subset of images related to good visibility were achieved by the adapted YOLOv5s model, reaching mAP:0.5 of 96.5% for CUDA architecture and 93.4% for edge devices. The same model achieved 87.8% and 86.8% for limited visibility. In both cases, the frame rate was approximately 7 FPS. The methods could be further improved for practical application in traditional or semi-autonomous car systems, drones, security cameras, etc.
更多查看译文
关键词
machine learning,multimodal vision,object detection,thermal imaging,human health,road safety,transportation infrastructure
AI 理解论文
溯源树
样例
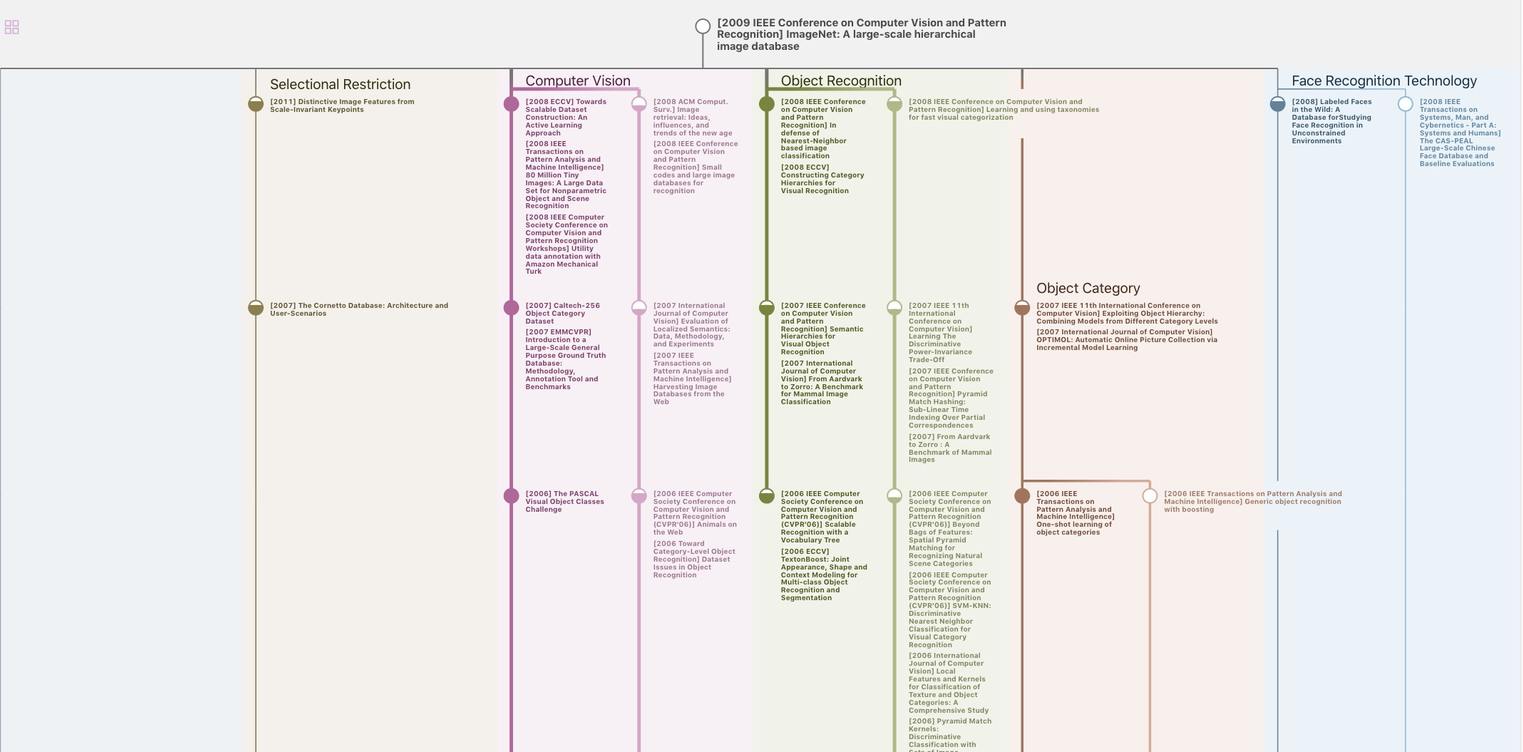
生成溯源树,研究论文发展脉络
Chat Paper
正在生成论文摘要