Maximizing the Influence of Social Networks Based on Graph Attention Networks
2023 3rd Asia-Pacific Conference on Communications Technology and Computer Science (ACCTCS)(2023)
摘要
It is a crucial algorithmic challenge in influence analysis to choose k important members to maximize the influence spread. This is known as the influence maximization (IM) problem in social networks. There are two main problems with the previous research for the IM problem: (1) the complex graph data can not be effectively used in the graph topology, (2) the problem of overlapping influences is prone to occur, which will lead to the solutions of the seed set being suboptimal. This paper suggests an influence maximization approach based on graph attention embedding (GAEIM) to address the aforementioned issues. GAEIM learns the shallow graph structure by Node2Vec and uses the graph attention mechanism to learn the deeper graph structure to extract information related to IM problems. Then, to reduce influence overlapping, we apply the cost-effective lazy forward algorithm (CELF) to choose the most influential seed nodes after selecting prospective seed nodes using the heuristic algorithm. In addition, we have demonstrated the monotone and submodular nature of the GAEIM. Thus, the proposed approach guarantees the approximate optimal solution to the IM problem. Across various datasets, the GAEIM algorithm exhibits superior influence propagation and effectiveness compared to existing IM algorithms, according to experimental results.
更多查看译文
关键词
graph neural network, social networks, graph topology, node2Vec, influence maximization
AI 理解论文
溯源树
样例
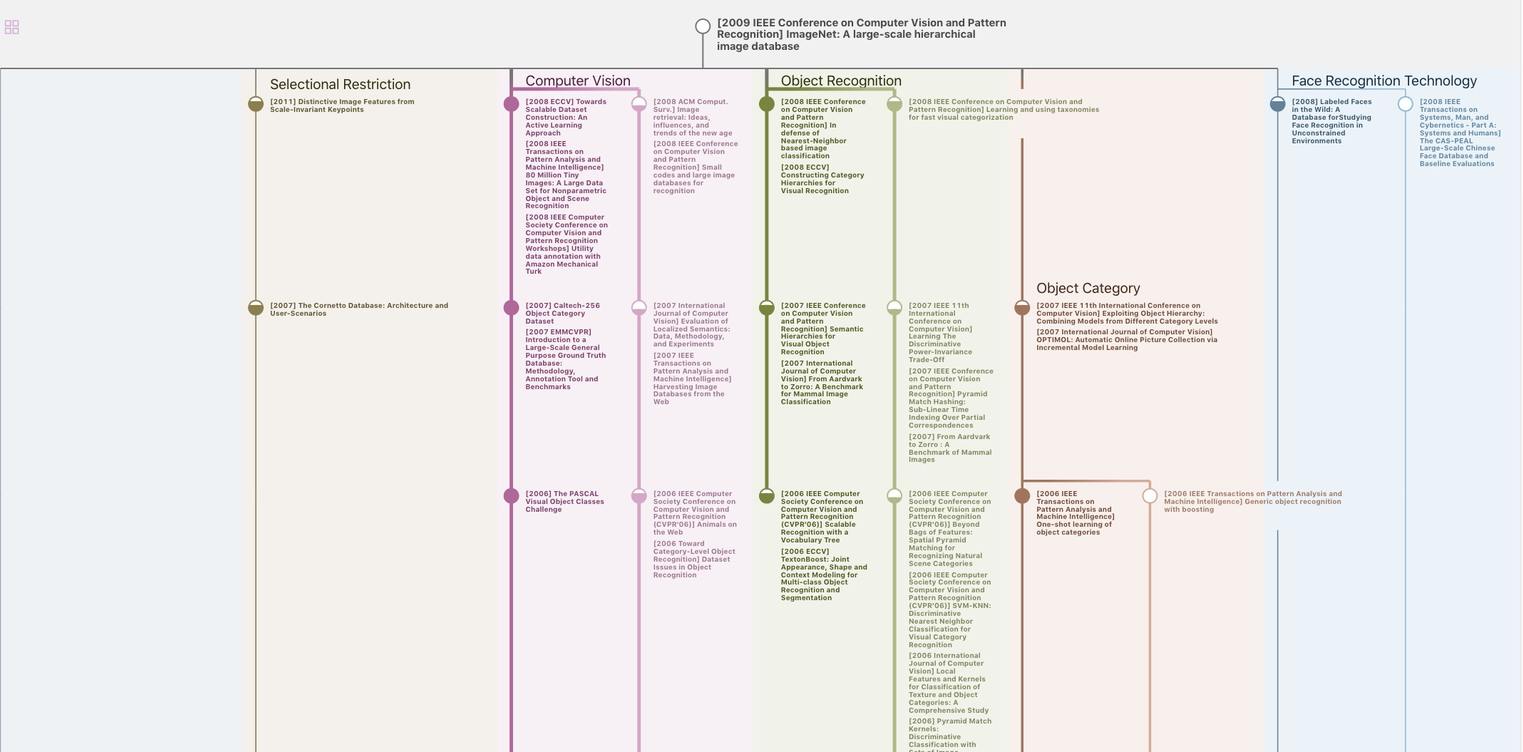
生成溯源树,研究论文发展脉络
Chat Paper
正在生成论文摘要