Multisensor Suboptimal Fusion Student's $t$ Filter
IEEE Transactions on Aerospace and Electronic Systems(2023)
摘要
A multisensor fusion Student's
$t$
filter is proposed for time-series recursive estimation in the presence of heavy-tailed process and measurement noises. It extends the single-sensor Student's
$t$
Kalman filter to the multisensor setup based on the suboptimal arithmetic average (AA) fusion approach which is driven from information-theoretic density fusion optimization and able to deal with unknown correlation among sensors. To ensure computationally efficient, closed-form
$t$
density recursion, moment matching approximation has been used for averaging the
$t$
densities aggregated from different sensors. Based on the same framework, we also extend the covariance intersection (CI) approach for
$t$
density fusion. Simulation demonstrates the strength of the proposed multisensor AA fusion-based
$t$
filter in dealing with outliers as compared with the classic Gaussian estimator, and the advantage of the AA fusion in comparison with the CI approach and the augmented measurement fusion.
更多查看译文
关键词
Correlation, Noise measurement, Probability density function, Optimization, Kalman filters, Arithmetic, Transforms, Arithmetic average (AA) fusion, covariance intersection (CI), heavy-tailed noise, multisensor fusion
AI 理解论文
溯源树
样例
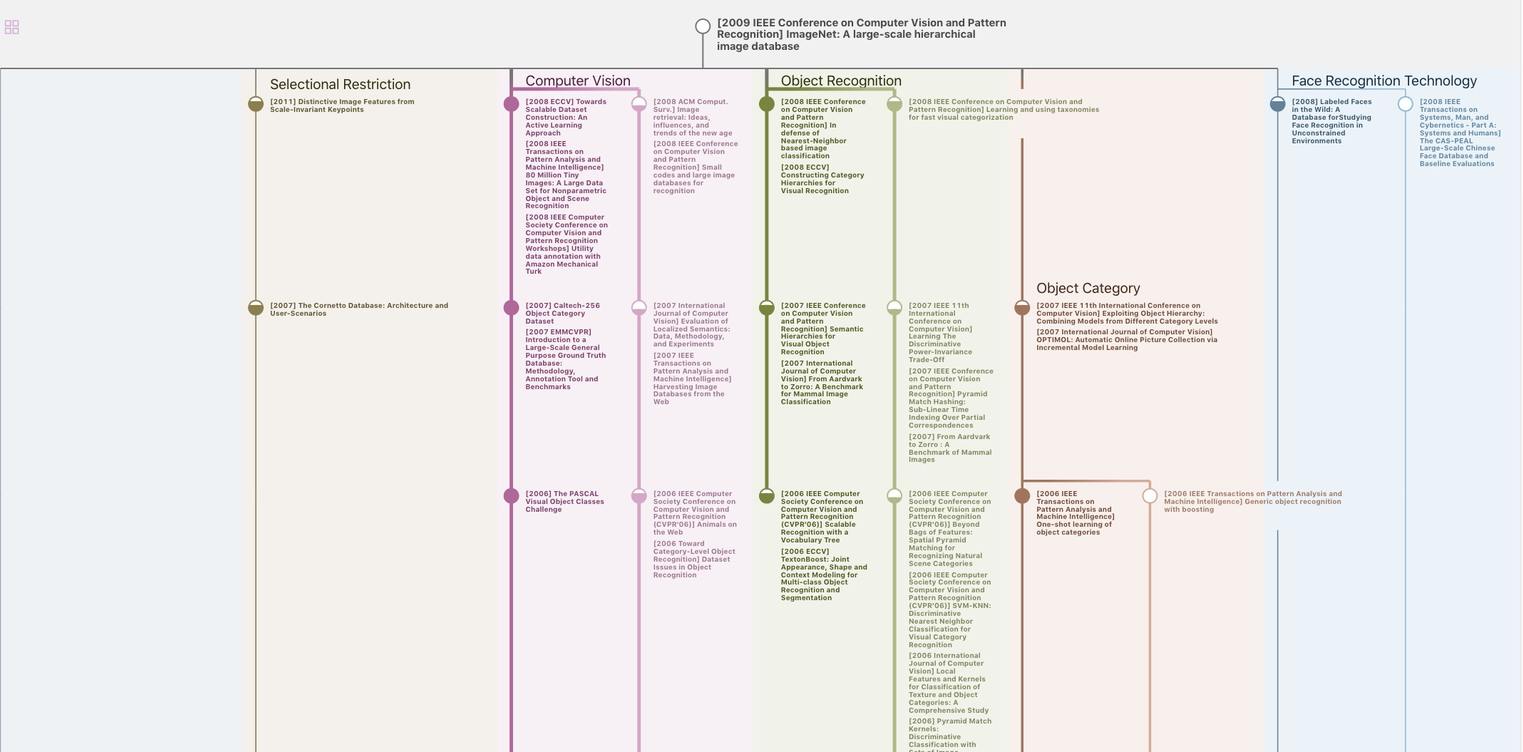
生成溯源树,研究论文发展脉络
Chat Paper
正在生成论文摘要