Finite element interpolated neural networks for solving forward and inverse problems
COMPUTER METHODS IN APPLIED MECHANICS AND ENGINEERING(2024)
摘要
We propose a general framework for solving forward and inverse problems constrained by partial differential equations, where we interpolate neural networks onto finite element spaces to represent the (partial) unknowns. The framework overcomes the challenges related to the imposition of boundary conditions, the choice of collocation points in physics-informed neural networks, and the integration of variational physics-informed neural networks. A numerical experiment set confirms the framework's capability of handling various forward and inverse problems. In particular, the trained neural network generalises well for smooth problems, beating finite element solutions by some orders of magnitude. We finally propose an effective one-loop solver with an initial data fitting step (to obtain a cheap initialisation) to solve inverse problems.
更多查看译文
关键词
Neural networks,PINNs,Finite elements,PDE approximation,Inverse problems
AI 理解论文
溯源树
样例
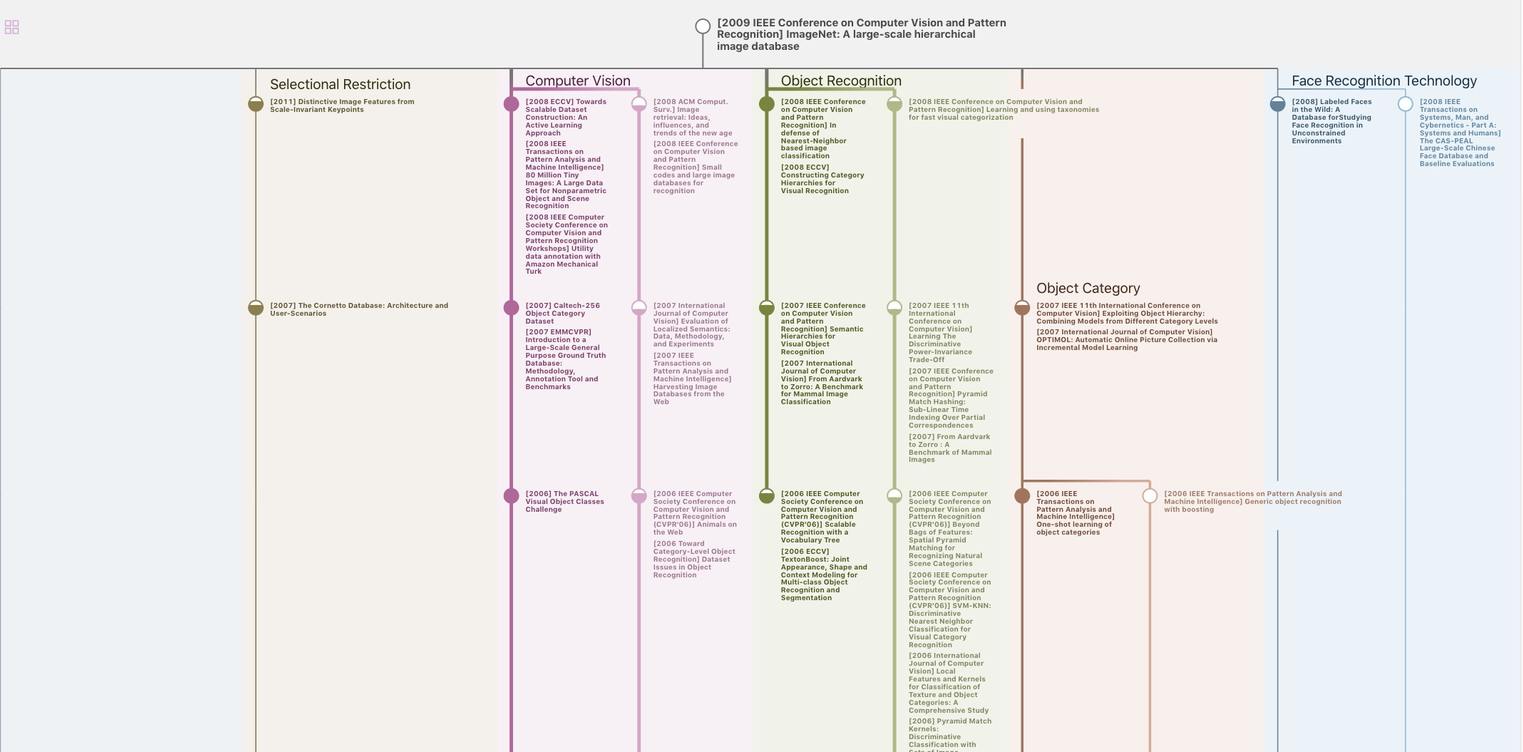
生成溯源树,研究论文发展脉络
Chat Paper
正在生成论文摘要