On robot grasp learning using equivariant models
CoRR(2023)
摘要
Real-world grasp detection is challenging due to the stochasticity in grasp dynamics and the noise in hardware. Ideally, the system would adapt to the real world by training directly on physical systems. However, this is generally difficult due to the large amount of training data required by most grasp learning models. In this paper, we note that the planar grasp function is SE(2) -equivariant and demonstrate that this structure can be used to constrain the neural network used during learning. This creates an inductive bias that can significantly improve the sample efficiency of grasp learning and enable end-to-end training from scratch on a physical robot with as few as 600 grasp attempts. We call this method Symmetric Grasp learning (SymGrasp) and show that it can learn to grasp “from scratch” in less that 1.5 h of physical robot time. This paper represents an expanded and revised version of the conference paper Zhu et al. ( 2022 ).
更多查看译文
关键词
Grasping,Equivariant models,On robot learning,Sample efficiency,Reinforcement learning,Transparent object grasping
AI 理解论文
溯源树
样例
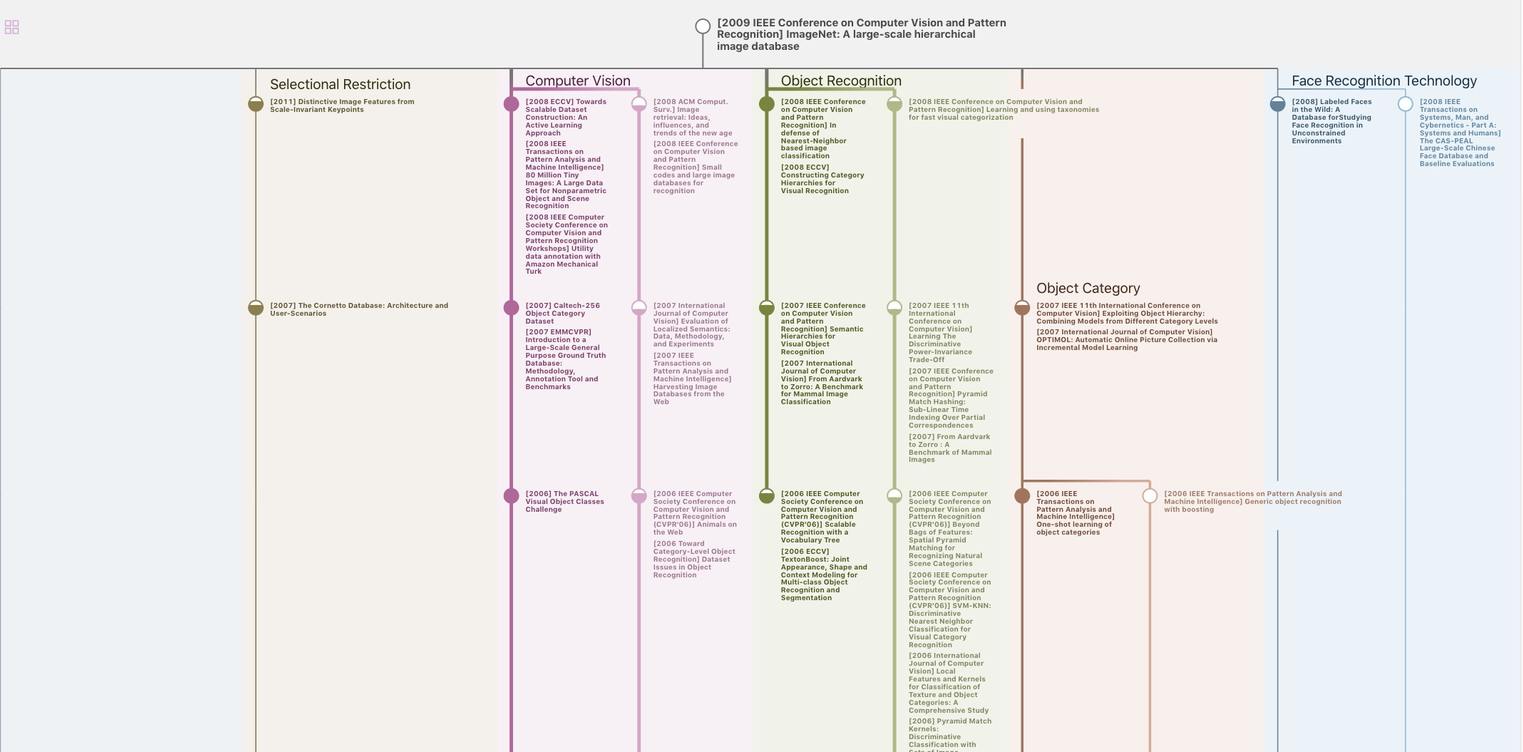
生成溯源树,研究论文发展脉络
Chat Paper
正在生成论文摘要