Are Intermediate Layers and Labels Really Necessary? A General Language Model Distillation Method
conf_acl(2023)
摘要
The large scale of pre-trained language models poses a challenge for their deployment on various devices, with a growing emphasis on methods to compress these models, particularly knowledge distillation. However, current knowledge distillation methods rely on the model's intermediate layer features and the golden labels (also called hard labels), which usually require aligned model architecture and enough labeled data respectively. Moreover, the parameters of vocabulary are usually neglected in existing methods. To address these problems, we propose a general language model distillation (GLMD) method that performs two-stage word prediction distillation and vocabulary compression, which is simple and surprisingly shows extremely strong performance. Specifically, GLMD supports more general application scenarios by eliminating the constraints of dimension and structure between models and the need for labeled datasets through the absence of intermediate layers and golden labels. Meanwhile, based on the long-tailed distribution of word frequencies in the data, GLMD designs a strategy of vocabulary compression through decreasing vocabulary size instead of dimensionality. Experimental results show that our method outperforms 25 state-of-the-art methods on the SuperGLUE benchmark, achieving an average score that surpasses the best method by 3%.
更多查看译文
关键词
intermediate layers,general language
AI 理解论文
溯源树
样例
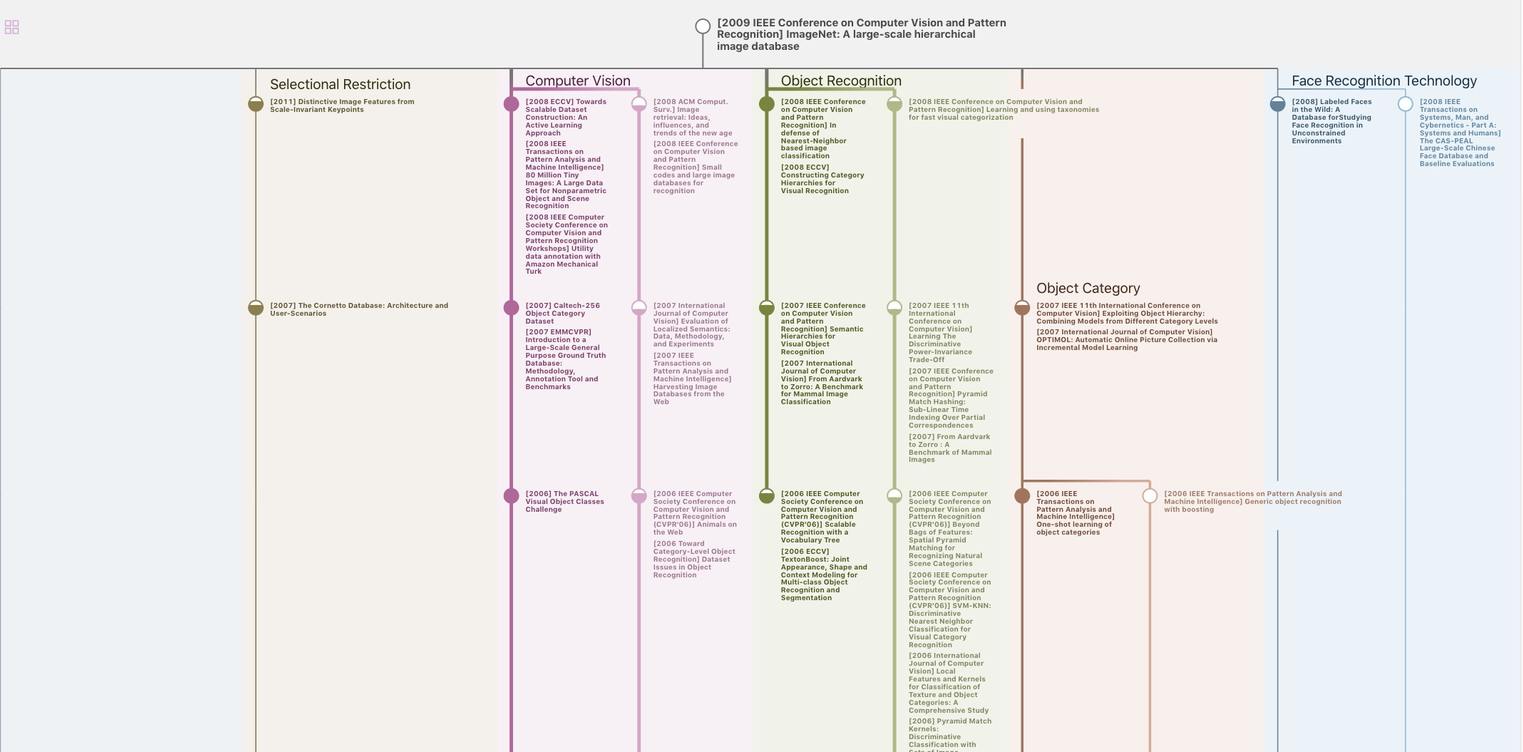
生成溯源树,研究论文发展脉络
Chat Paper
正在生成论文摘要