2-D SSM: A General Spatial Layer for Visual Transformers
CoRR(2023)
摘要
A central objective in computer vision is to design models with appropriate 2-D inductive bias. Desiderata for 2D inductive bias include two-dimensional position awareness, dynamic spatial locality, and translation and permutation invariance. To address these goals, we leverage an expressive variation of the multidimensional State Space Model (SSM). Our approach introduces efficient parameterization, accelerated computation, and a suitable normalization scheme. Empirically, we observe that incorporating our layer at the beginning of each transformer block of Vision Transformers (ViT) significantly enhances performance for multiple ViT backbones and across datasets. The new layer is effective even with a negligible amount of additional parameters and inference time. Ablation studies and visualizations demonstrate that the layer has a strong 2-D inductive bias. For example, vision transformers equipped with our layer exhibit effective performance even without positional encoding
更多查看译文
关键词
visual transformers,general spatial layer
AI 理解论文
溯源树
样例
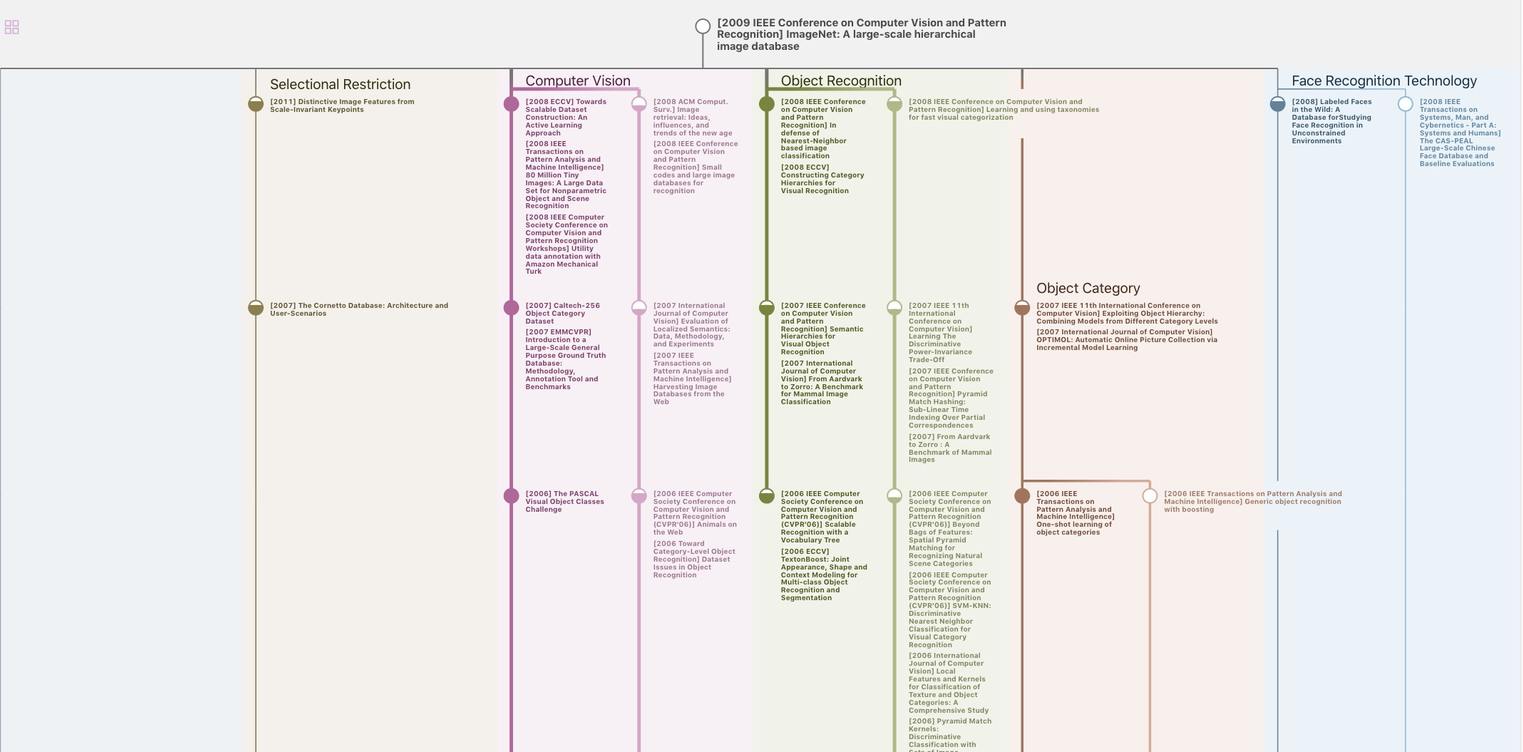
生成溯源树,研究论文发展脉络
Chat Paper
正在生成论文摘要