Efficient Learning of Minimax Risk Classifiers in High Dimensions
CoRR(2023)
摘要
High-dimensional data is common in multiple areas, such as health care and genomics, where the number of features can be tens of thousands. In such scenarios, the large number of features often leads to inefficient learning. Constraint generation methods have recently enabled efficient learning of L1-regularized support vector machines (SVMs). In this paper, we leverage such methods to obtain an efficient learning algorithm for the recently proposed minimax risk classifiers (MRCs). The proposed iterative algorithm also provides a sequence of worst-case error probabilities and performs feature selection. Experiments on multiple high-dimensional datasets show that the proposed algorithm is efficient in high-dimensional scenarios. In addition, the worst-case error probability provides useful information about the classifier performance, and the features selected by the algorithm are competitive with the state-of-the-art.
更多查看译文
关键词
minimax risk classifiers,learning,high dimensions
AI 理解论文
溯源树
样例
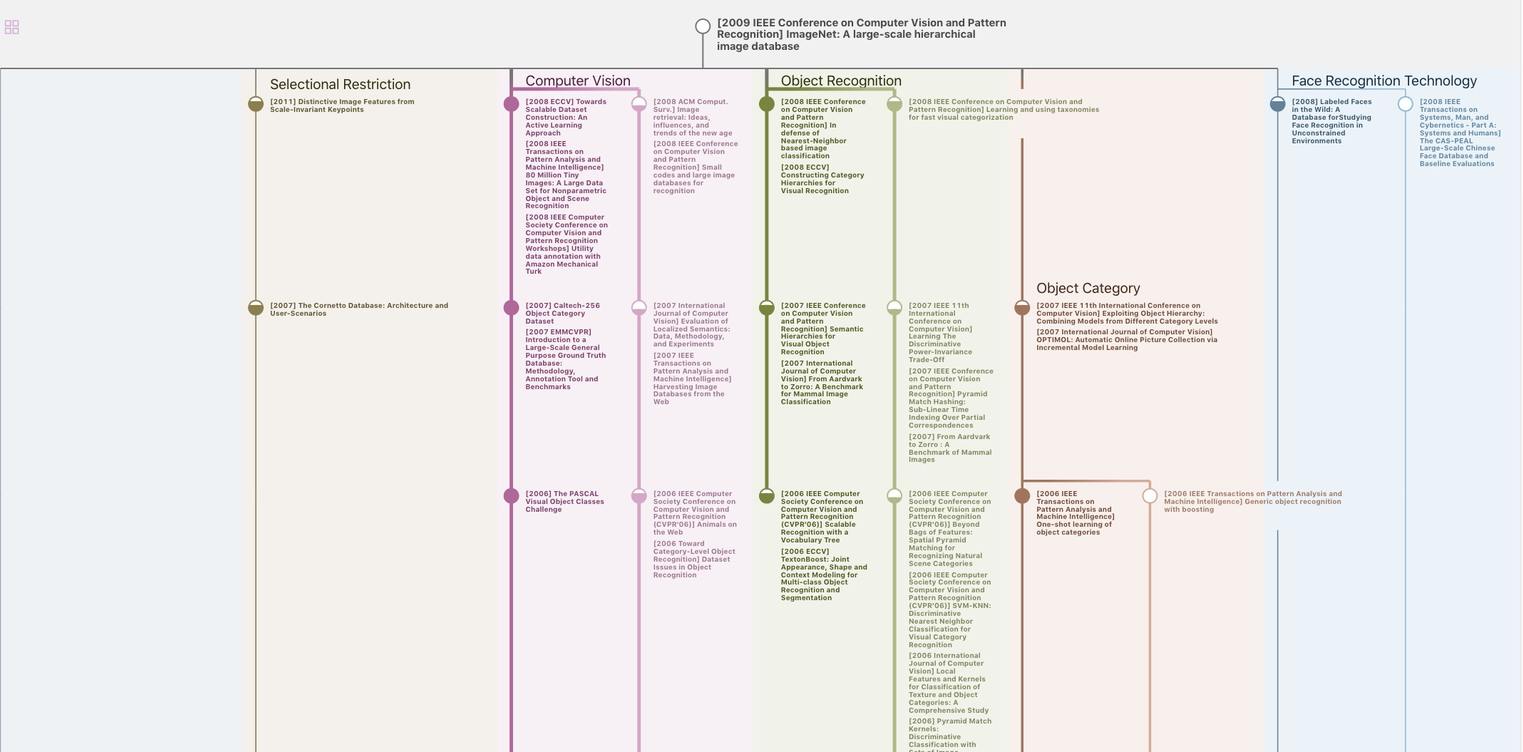
生成溯源树,研究论文发展脉络
Chat Paper
正在生成论文摘要