MedGAN: An adaptive GAN approach for medical image generation
Computers in Biology and Medicine(2023)
摘要
Generative adversarial networks (GANs) and their variants as an effective method for generating visually appealing images have shown great potential in different medical imaging applications during past decades. However, some issues remain insufficiently investigated: many models still suffer from model collapse, vanishing gradients, and convergence failure. Considering the fact that medical images differ from typical RGB images in terms of complexity and dimensionality, we propose an adaptive generative adversarial network, namely MedGAN, to mitigate these issues. Specifically, we first use Wasserstein loss as a convergence metric to measure the convergence degree of the generator and the discriminator. Then, we adaptively train MedGAN based on this metric. Finally, we generate medical images based on MedGAN and use them to build few -shot medical data learning models for disease classification and lesion localization. On demodicosis, blister, molluscum, and parakeratosis datasets, our experimental results verify the advantages of MedGAN in model convergence, training speed, and visual quality of generated samples. We believe this approach can be generalized to other medical applications and contribute to radiologists' efforts for disease diagnosis. The source code can be downloaded at https://github.com/geyao-c/MedGAN.
更多查看译文
关键词
Generative adversarial network,Medical imaging,Image synthesis,Deep learning
AI 理解论文
溯源树
样例
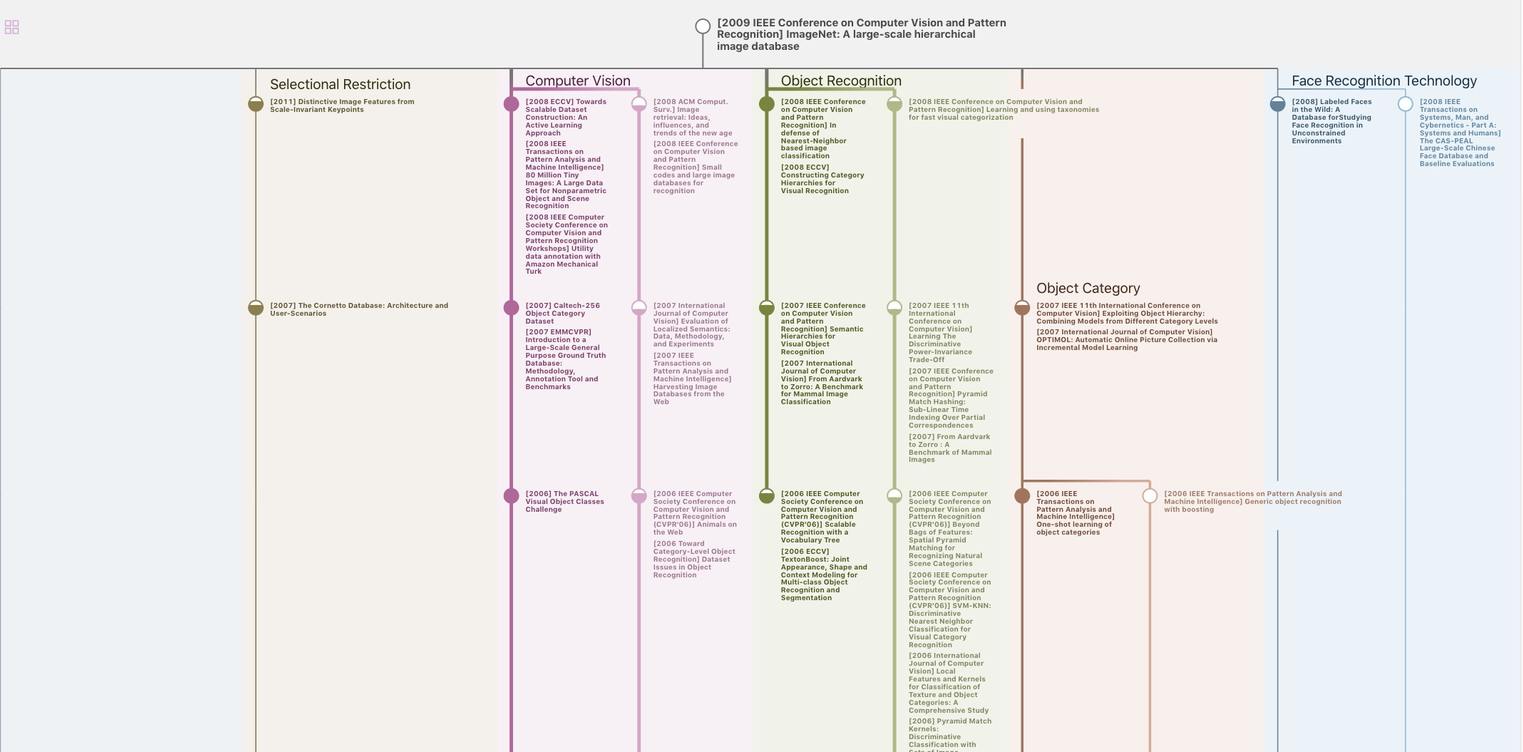
生成溯源树,研究论文发展脉络
Chat Paper
正在生成论文摘要