Least-Squares Neural Network (LSNN) Method For Linear Advection-Reaction Equation: Non-constant Jumps
CoRR(2023)
摘要
The least-squares ReLU neural network (LSNN) method was introduced and
studied for solving linear advection-reaction equation with discontinuous
solution in . The method is based on an
equivalent least-squares formulation and employs ReLU neural network (NN)
functions with ⌈log_2(d+1)⌉+1-layer representations for
approximating solutions. In this paper, we show theoretically that the method
is also capable of approximating non-constant jumps along discontinuous
interfaces that are not necessarily straight lines. Numerical results for test
problems with various non-constant jumps and interfaces show that the LSNN
method with ⌈log_2(d+1)⌉+1 layers approximates solutions
accurately with degrees of freedom less than that of mesh-based methods and
without the common Gibbs phenomena along discontinuous interfaces.
更多查看译文
关键词
neural network,lsnn,linear,least-squares,advection-reaction,non-constant
AI 理解论文
溯源树
样例
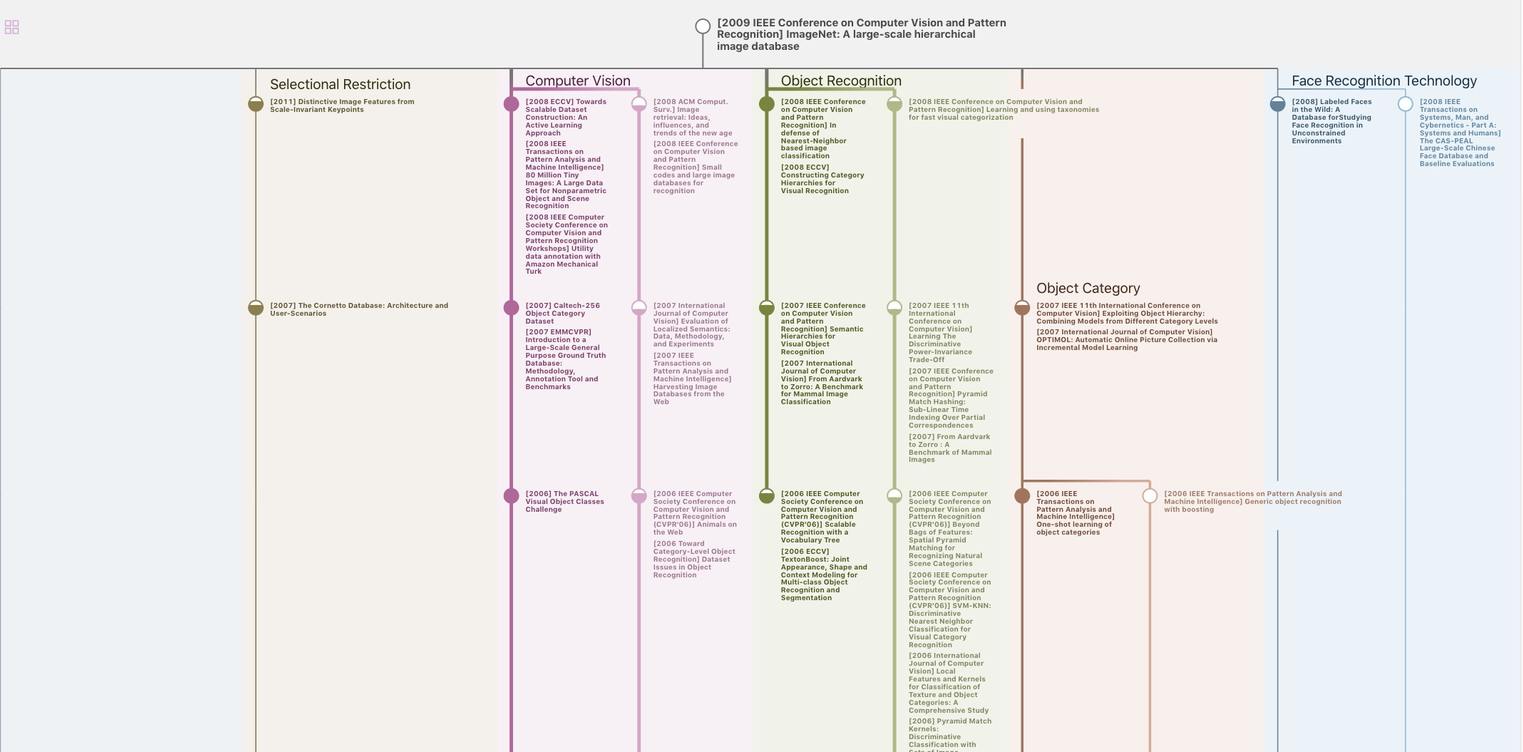
生成溯源树,研究论文发展脉络
Chat Paper
正在生成论文摘要