Unlocking Foundation Models for Privacy-Enhancing Speech Understanding: An Early Study on Low Resource Speech Training Leveraging Label-guided Synthetic Speech Content
CoRR(2023)
摘要
Automatic Speech Understanding (ASU) leverages the power of deep learning models for accurate interpretation of human speech, leading to a wide range of speech applications that enrich the human experience. However, training a robust ASU model requires the curation of a large number of speech samples, creating risks for privacy breaches. In this work, we investigate using foundation models to assist privacy-enhancing speech computing. Unlike conventional works focusing primarily on data perturbation or distributed algorithms, our work studies the possibilities of using pre-trained generative models to synthesize speech content as training data with just label guidance. We show that zero-shot learning with training label-guided synthetic speech content remains a challenging task. On the other hand, our results demonstrate that the model trained with synthetic speech samples provides an effective initialization point for low-resource ASU training. This result reveals the potential to enhance privacy by reducing user data collection but using label-guided synthetic speech content.
更多查看译文
关键词
speech,privacy-enhancing,label-guided
AI 理解论文
溯源树
样例
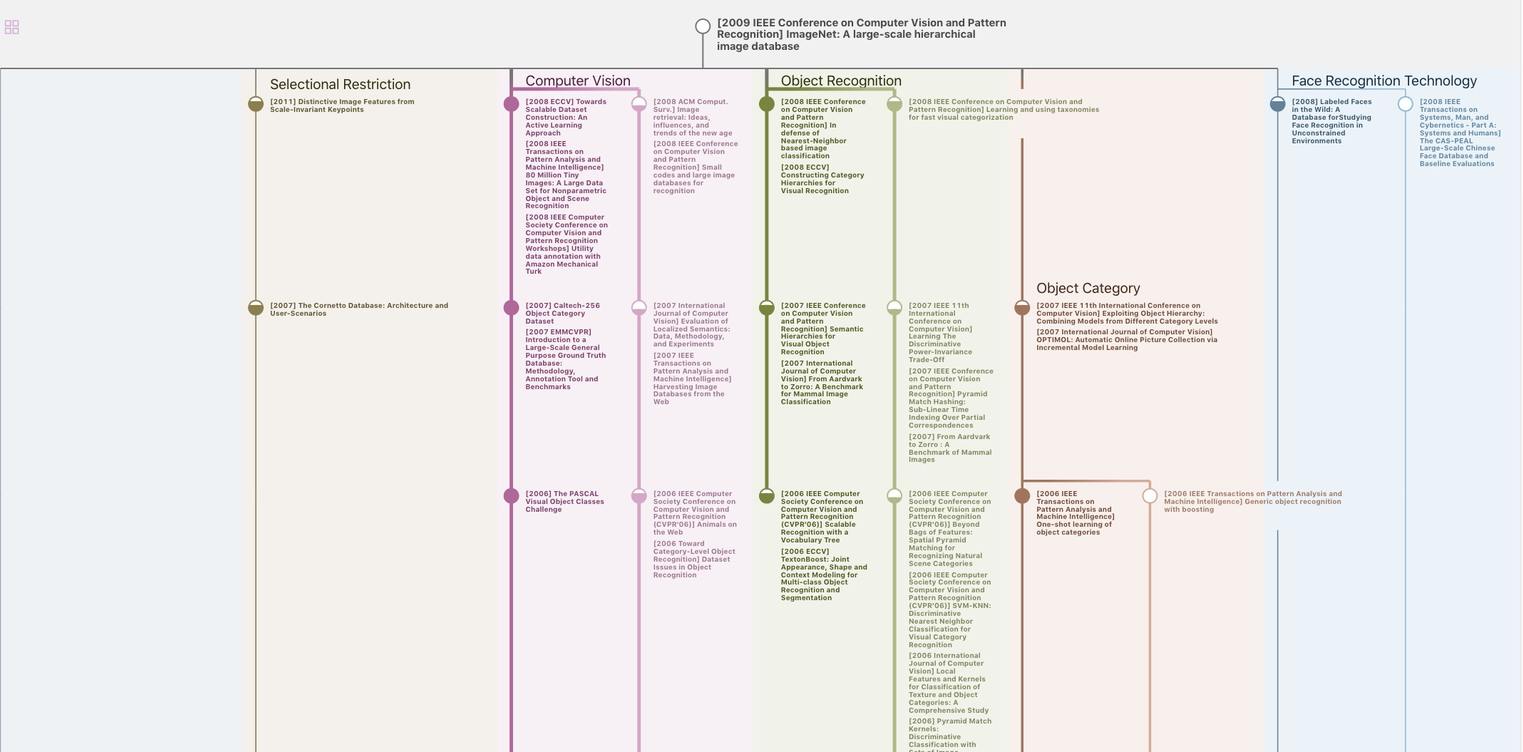
生成溯源树,研究论文发展脉络
Chat Paper
正在生成论文摘要