Graph Laplacian Learning with Exponential Family Noise
CoRR(2023)
摘要
A common challenge in applying graph machine learning methods is that the underlying graph of a system is often unknown. Although different graph inference methods have been proposed for continuous graph signals, inferring the graph structure underlying other types of data, such as discrete counts, is under-explored. In this paper, we generalize a graph signal processing (GSP) framework for learning a graph from smooth graph signals to the exponential family noise distribution to model various data types. We propose an alternating algorithm that estimates the graph Laplacian as well as the unobserved smooth representation from the noisy signals. We demonstrate in synthetic and real-world data that our new algorithm outperforms competing Laplacian estimation methods under noise model mismatch.
更多查看译文
关键词
exponential family noise,learning
AI 理解论文
溯源树
样例
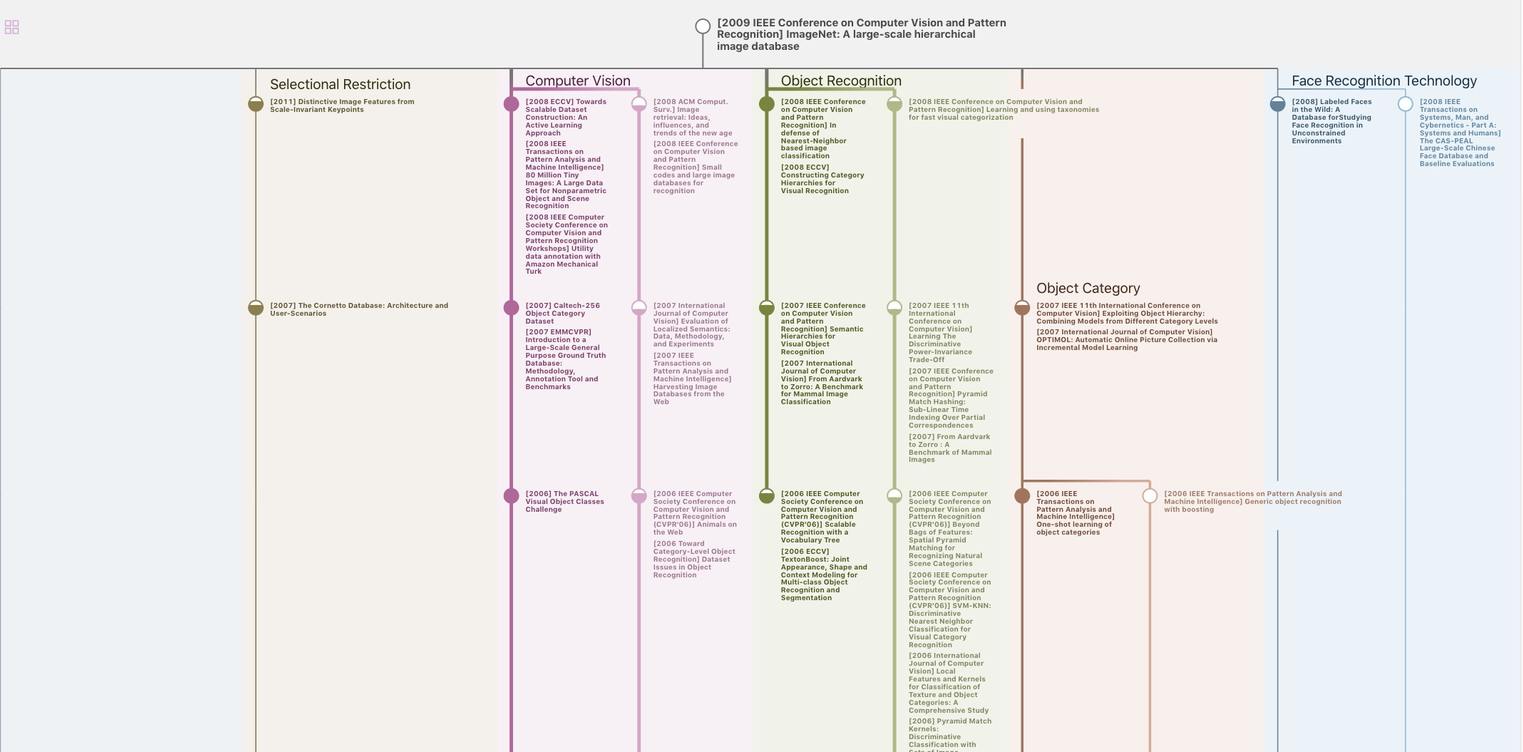
生成溯源树,研究论文发展脉络
Chat Paper
正在生成论文摘要