HOSSnet: an Efficient Physics-Guided Neural Network for Simulating Crack Propagation
CoRR(2023)
摘要
Hybrid Optimization Software Suite (HOSS), which is a combined finite-discrete element method (FDEM), is one of the advanced approaches to simulating high-fidelity fracture and fragmentation processes but the application of pure HOSS simulation is computationally expensive. At the same time, machine learning methods, shown tremendous success in several scientific problems, are increasingly being considered promising alternatives to physics-based models in the scientific domains. Thus, our goal in this work is to build a new data-driven methodology to reconstruct the crack fracture accurately in the spatial and temporal fields. We leverage physical constraints to regularize the fracture propagation in the long-term reconstruction. In addition, we introduce perceptual loss and several extra pure machine learning optimization approaches to improve the reconstruction performance of fracture data further. We demonstrate the effectiveness of our proposed method through both extrapolation and interpolation experiments. The results confirm that our proposed method can reconstruct high-fidelity fracture data over space and time in terms of pixel-wise reconstruction error and structural similarity. Visual comparisons also show promising results in long-term
更多查看译文
关键词
crack
AI 理解论文
溯源树
样例
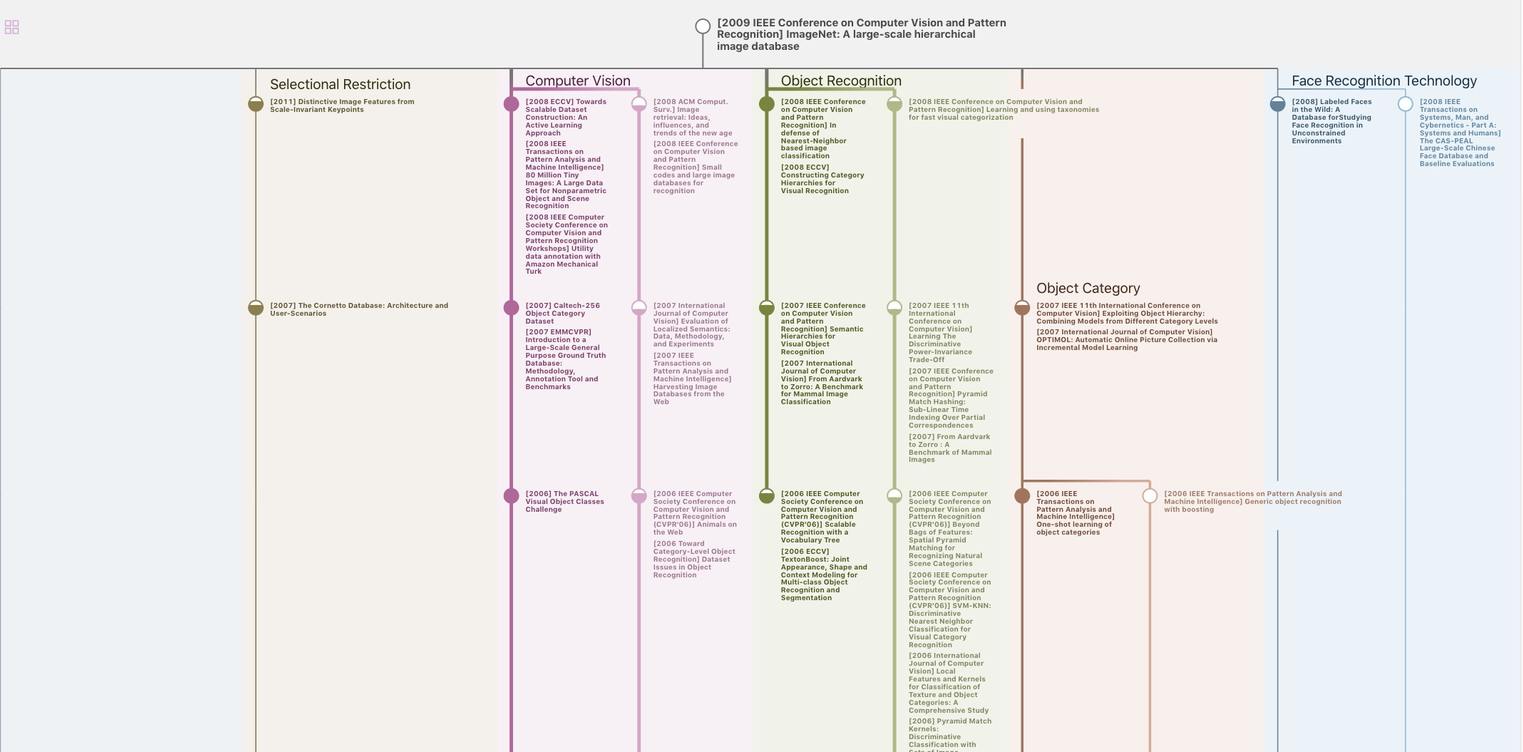
生成溯源树,研究论文发展脉络
Chat Paper
正在生成论文摘要