Unsupervised speech intelligibility assessment with utterance level alignment distance between teacher and learner Wav2Vec-2.0 representations
CoRR(2023)
摘要
Speech intelligibility is crucial in language learning for effective communication. Thus, to develop computer-assisted language learning systems, automatic speech intelligibility detection (SID) is necessary. Most of the works have assessed the intelligibility in a supervised manner considering manual annotations, which requires cost and time; hence scalability is limited. To overcome these, this work proposes an unsupervised approach for SID. The proposed approach considers alignment distance computed with dynamic-time warping (DTW) between teacher and learner representation sequence as a measure to separate intelligible versus non-intelligible speech. We obtain the feature sequence using current state-of-the-art self-supervised representations from Wav2Vec-2.0. We found the detection accuracies as 90.37\%, 92.57\% and 96.58\%, respectively, with three alignment distance measures -- mean absolute error, mean squared error and cosine distance (equal to one minus cosine similarity).
更多查看译文
关键词
unsupervised speech intelligibility assessment,utterance level
AI 理解论文
溯源树
样例
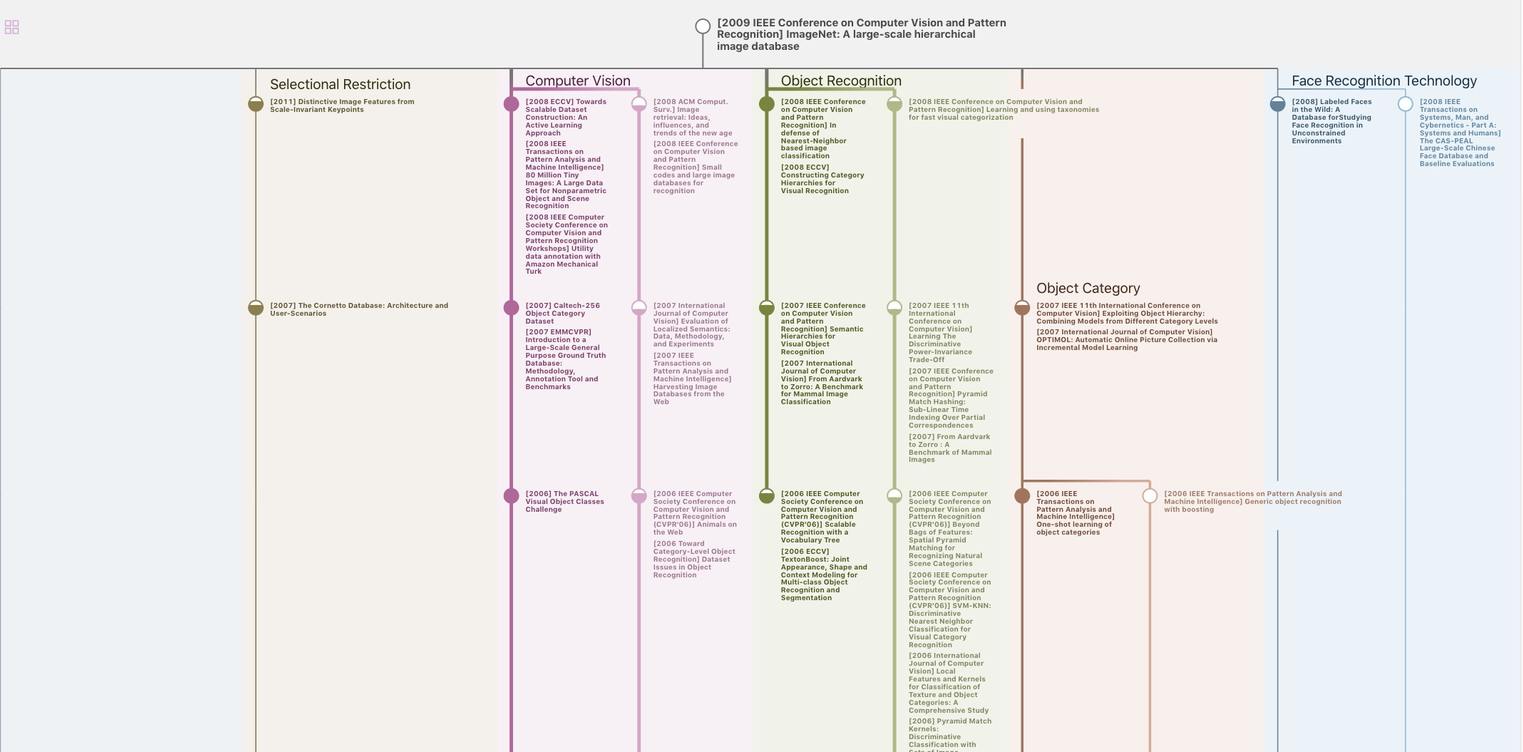
生成溯源树,研究论文发展脉络
Chat Paper
正在生成论文摘要