Distilling Invariant Representations With Domain Adversarial Learning for Cross-Subject Children Seizure Prediction
IEEE TRANSACTIONS ON COGNITIVE AND DEVELOPMENTAL SYSTEMS(2024)
摘要
Seizure prediction based on electroencephalogram (EEG) has great potential to improve patients' life quality. Due to the high heterogeneity in distributions of EEG signals among different patients, conventional studies usually show poor generalization ability when transferring the model to new patients, which also leads to difficulties in clinical applications. To alleviate the challenging issue concerning cross-subject domain shift, we propose a transformer-based domain adversarial model. Our model first adopts a pretrained general neural network to extract common features from the EEG signals of available patients. Then, we design a distiller module and a domain discriminator module to perform domain adaptation training based on a small amount of labeled data from the new-coming patient. During the adaptation process, conditional domain adversarial training with the addition of label information is employed to remove patient-related information from the extracted features to learn a common seizure feature space among different patients. Our proposed seizure prediction method is evaluated on the CHB-MIT EEG database. The proposed model achieves a sensitivity of 79.5%, a false alarm rate (FPR) of 0.258/h, and an AUC of 0.814. Experimental results demonstrate that the proposed method can effectively reduce interpatient domain disparity compared to state-of-the-art methods.
更多查看译文
关键词
Feature extraction,Electroencephalography,Brain modeling,Transformers,Training,Task analysis,Predictive models,Adversarial learning,domain adaptation (DA),electroencephalogram (EEG),seizure prediction,transformer
AI 理解论文
溯源树
样例
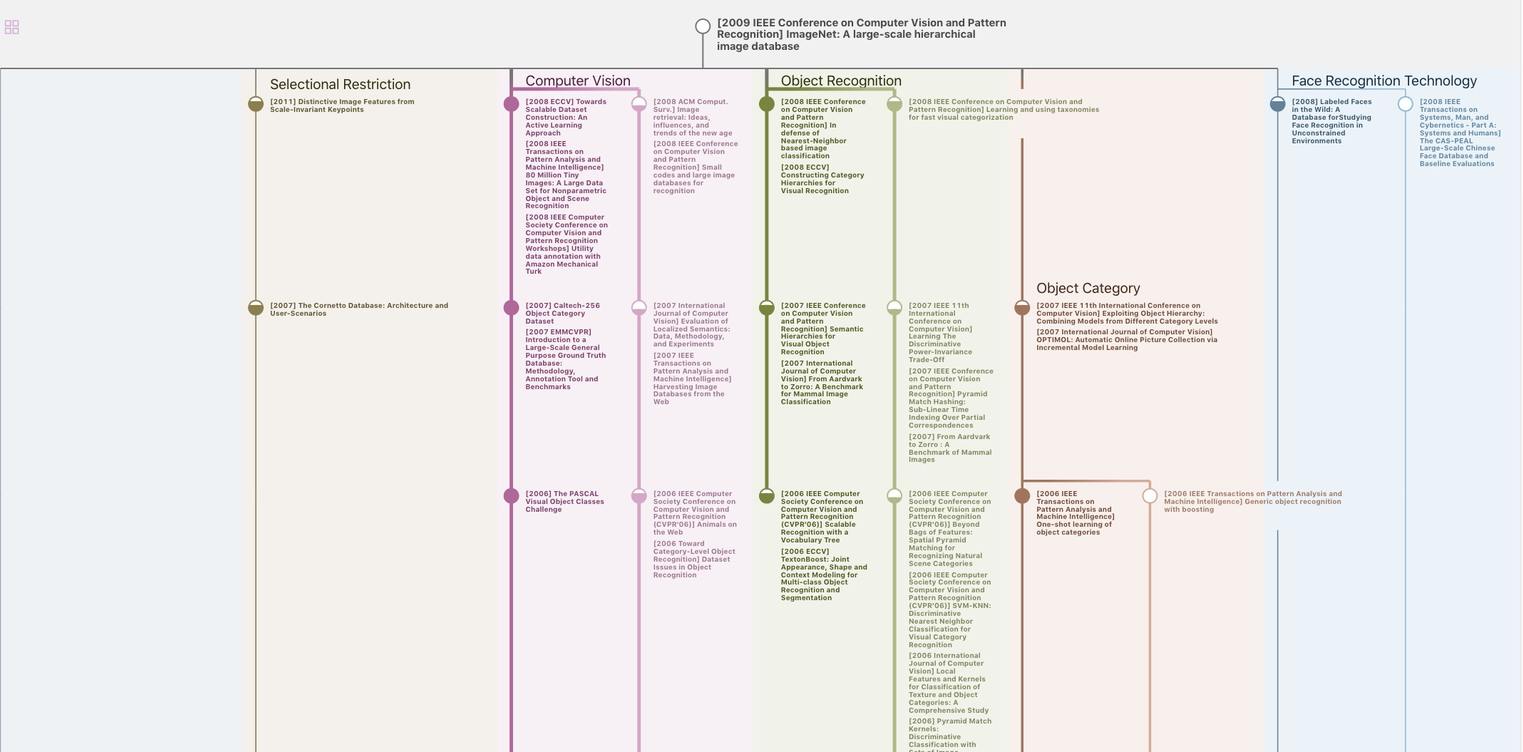
生成溯源树,研究论文发展脉络
Chat Paper
正在生成论文摘要