Cross-Modal Fusion Convolutional Neural Networks With Online Soft-Label Training Strategy for Mechanical Fault Diagnosis
IEEE TRANSACTIONS ON INDUSTRIAL INFORMATICS(2024)
摘要
Convolutional neural network (CNN)-based fault detection approaches based on multisource signals have attracted increasing interest from the research community and industrial practices, thanks to the powerful feature representation capability of CNN and the rapid development of sensor technology. Various strategies have been applied in existing CNN-based diagnostic models to learn features from 1-D real-valued multivariate data. However, the distribution gap and the intrinsic correlations among multisource mechanical signals during the learning process have been rarely considered, which may lead to suboptimal fault identification results. To tackle this issue, this article proposes a cross-modal fusion convolutional neural network (CMFCNN) for mechanical fault diagnosis, which performs modality-specific and cross-modal feature representation on multisource data. Specifically, CMFCNN adopts two parallel modality-specific networks and a cross-modal knowledge-sharing network to fully explore independent and shared features from the multisource mechanical signals. To achieve effective feature propagation and fusion, a cross-modal fusion module is introduced to integrate cross-modal features and pass the fused information to the next layer. Moreover, to alleviate overfitting and achieve a better diagnostic performance of the framework, an online soft-label training algorithm is adopted in the CMFCNN training phase. Extensive experimental results on the cylindrical rolling bearing dataset and the planetary gearbox dataset validate that the proposed CMFCNN outperforms seven state-of-the-art methods significantly, especially under strong noise conditions.
更多查看译文
关键词
Convolutional neural network (CNN),cross-modal fusion (CMF) module,fault diagnosis,multisource mechanical signals,online soft-label training (OSLT)
AI 理解论文
溯源树
样例
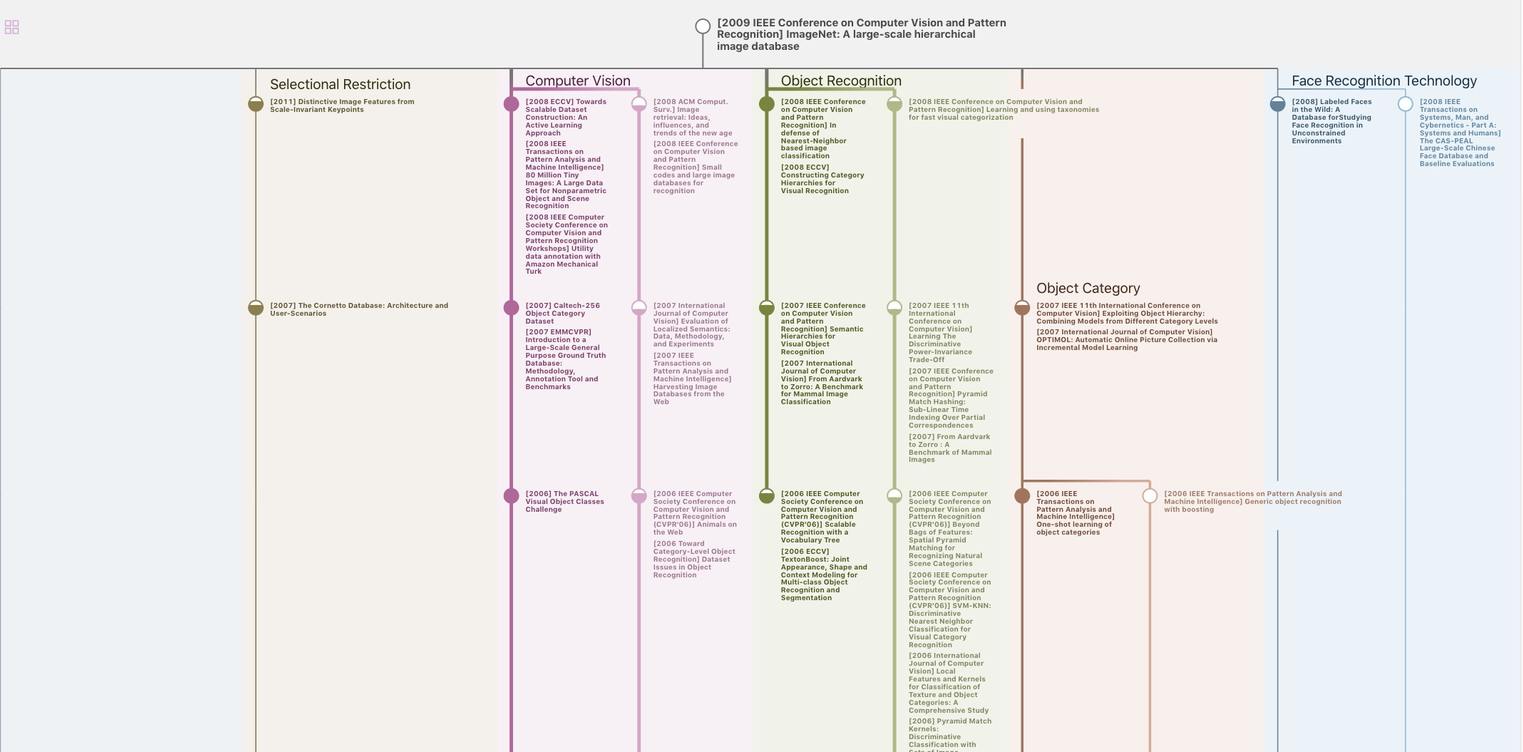
生成溯源树,研究论文发展脉络
Chat Paper
正在生成论文摘要