Regularization Strength Impact on Neural Network Ensembles.
Proceedings of the 2022 5th International Conference on Algorithms, Computing and Artificial Intelligence(2022)
摘要
In the last decade, several approaches have been proposed for regularizing deeper and wider neural networks (NNs), which is of importance in areas like image classification. It is now common practice to incorporate several regularization approaches in the training procedure of NNs. However, the impact of regularization strength on the properties of an ensemble of NNs remains unclear. For this reason, the study empirically compared the impact of NNs built based on two different regularization strengths (weak regularization (WR) and strong regularization (SR)) on the properties of an ensemble, such as the magnitude of logits, classification accuracy, calibration error, and ability to separate true predictions (TPs) and false predictions (FPs). The comparison was based on results from different experiments conducted on three different models, datasets, and architectures. Experimental results show that the increase in regularization strength 1) reduces the magnitude of logits; 2) can increase or decrease the classification accuracy depending on the dataset and/or architecture; 3) increases the calibration error; and 4) can improve or harm the separability between TPs and FPs depending on the dataset, architecture, model type and/or FP type.
更多查看译文
AI 理解论文
溯源树
样例
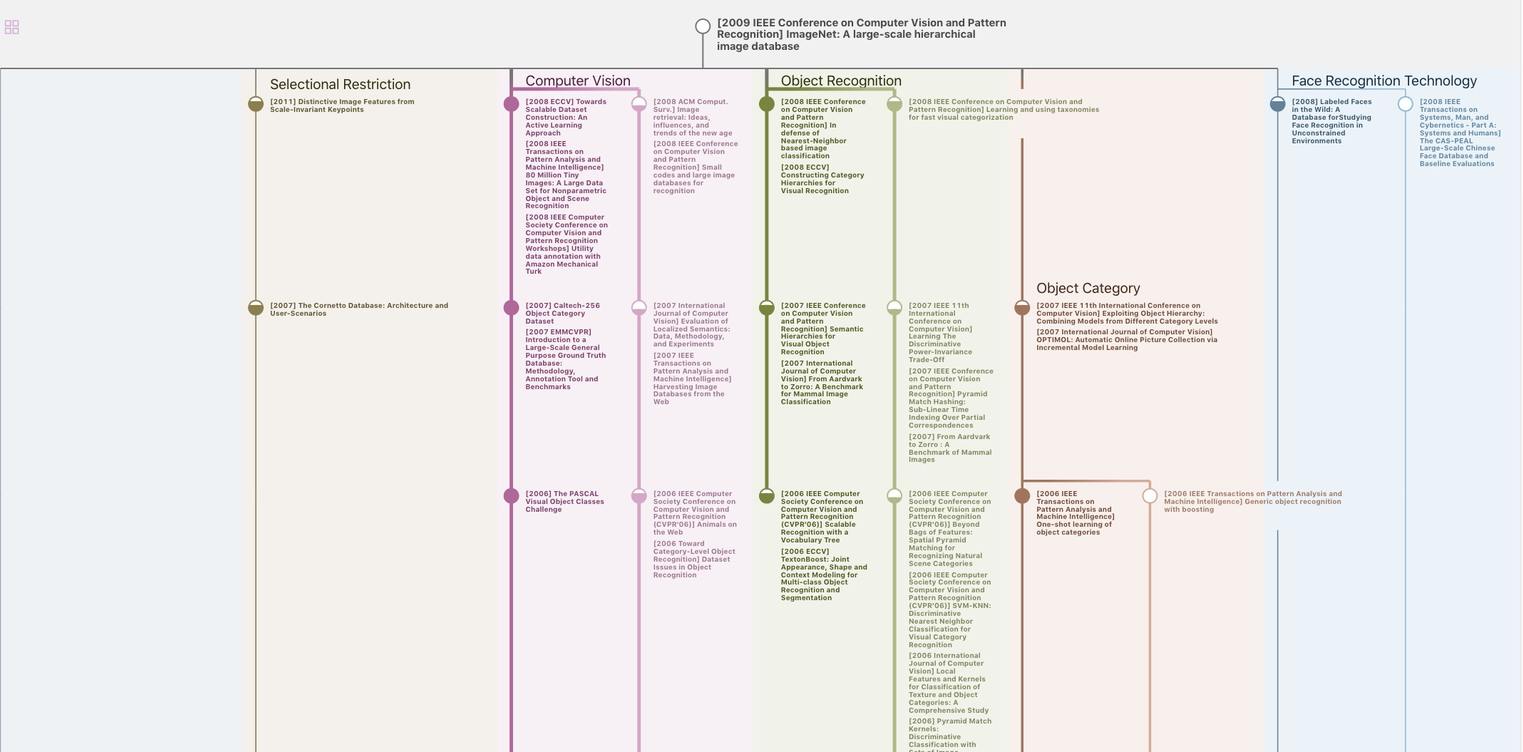
生成溯源树,研究论文发展脉络
Chat Paper
正在生成论文摘要