Radiation-Less Monitoring of Scoliosis Based on Single Back Photographs Using Smartphones and an Open System Powered by Deep Learning: System Development and Longitudinal Evaluation (Preprint)
crossref(2022)
摘要
BACKGROUND Routine check-ups for adolescent idiopathic scoliosis are critical to monitor progression and prescribe interventions. AIS is primarily screened via physical examination. If there are features of deformity, radiographs are necessary for diagnosis or follow-up, guiding further management, i.e., bracing corrections for moderate deformities and spine surgeries for severe deformities. However, this subjects children to repetitive radiation and routine practices can be disturbed. OBJECTIVE We aim to develop and prospectively validate an open mobile platform powered by validated deep learning models known as ScolioNets, for AIS severity and curve type classifications as well as progression identifications, to facilitate timely management of AIS with no extra radiation exposure. METHODS During the technology development stage, ScolioNets was trained and validated by 1780 back photos, consisting of heterogeneous severities and curve types. The ground truth (GT) labels for severity (follow-up vs. considering surgery), curve type (thoracic, thoracolumbar/lumbar, or mixed curve types) and progression (progress vs. non-grogress) were manually annotated by experienced deformity surgeons based on the radiographic examinations of the participants’ spines. Further prospective testing on 378 patients was conducted after the validated ScolioNets was deployed on our open platform AlignProCARE. In addition to comparing with the GTs, the results were also compared with two spine surgeons’ assessments of nude back appearance, while they were blinded to the GT measurements. Quantitative statistical analyses were performed to assess the performance of the open platform in classifying the deformity as well as in distinguishing progression during follow-up. RESULTS The platform recommended follow-up or surgery with the area under curves (AUCs) of receiver operating characteristic curves being 0.839, and 0.902, while distinguishing among thoracic, thoracolumbar/lumbar, or mixed curve types with AUCs of 0.777, 0.760 and 0.860 respectively. For follow-ups, we distinguished subjects with or without curve progression with an AUC of 0.757. All results were comparable with the senior surgeon’s assessment results and superior to the junior spine surgeon’s results. CONCLUSIONS Our open platform has the potential for out-of-hospital accessible and radiation-free applications in managing children with AIS, with comparable performance as a spine surgeon experienced in AIS management.
更多查看译文
AI 理解论文
溯源树
样例
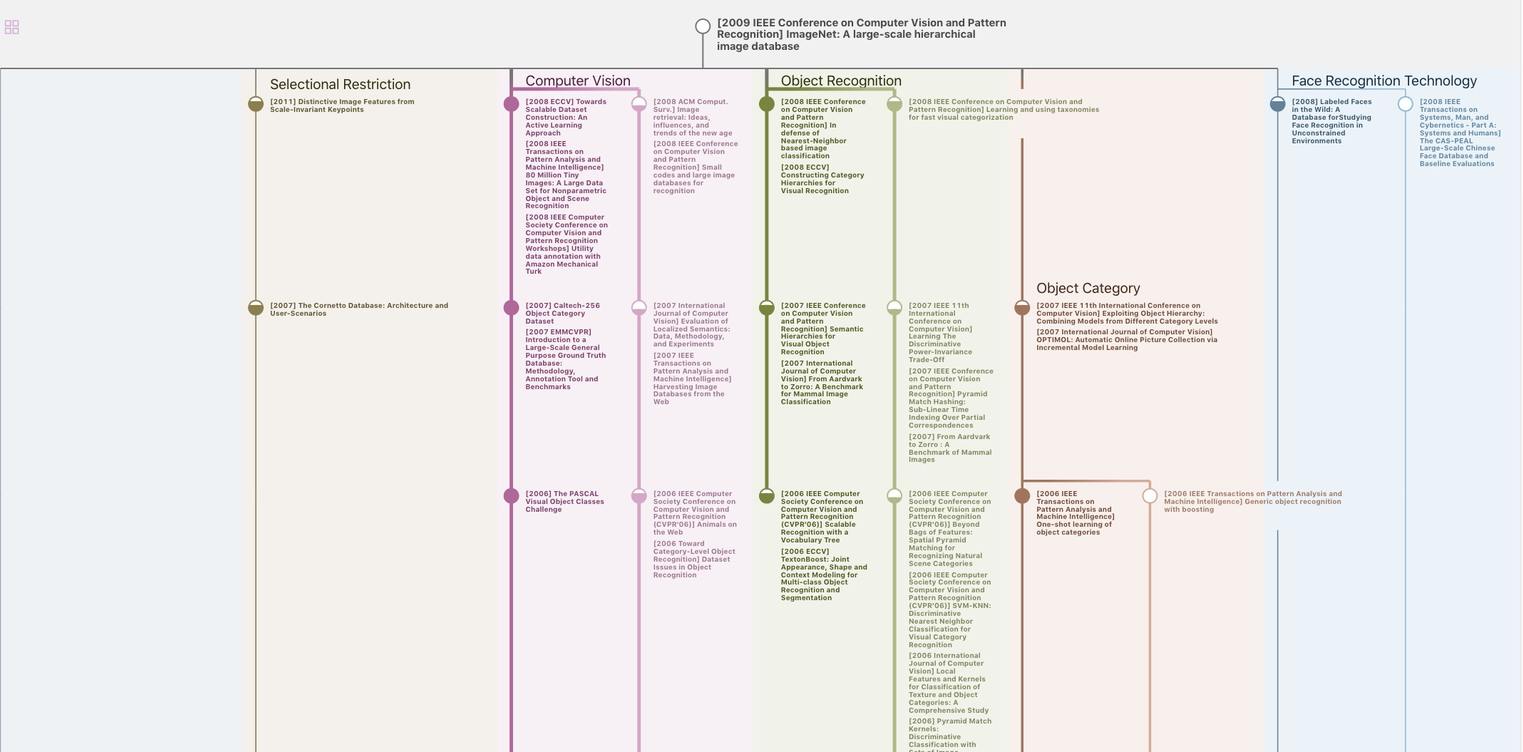
生成溯源树,研究论文发展脉络
Chat Paper
正在生成论文摘要