Predictive accuracy of computer-generated padua nephrometry scores based on continuous variables compared with categorical computer-generated scores and humangenerated scores in predicting oncologic and perioperative outcomes.
Journal of Clinical Oncology(2023)
摘要
624 Background: The Preoperative Aspects and Dimensions Used for Anatomical Classification (PADUA) score is a validated predictor of a patient’s perioperative outcomes following partial nephrectomy. The use of a fully automated, unbiased and time sensitive PADUA scoring system is a novel concept that may help circumvent PADUA’s widespread adoptability. We sought to automate the scoring of preoperative computed tomography scans (CT) using a machine-learning-generated PADUA score (P-AI). In doing so, we aimed to compare P-AI’s ability to predict meaningful perioperative and oncologic outcomes as compared to human generated PADUA scores using both categorical (P-H) and continuous variables (P-AI+). Methods: 300 patients with pre-operative CT scans were identified from a cohort of 544 consecutive patients undergoing surgical extirpation for suspected renal cancer at a single institution. A deep neural network approach was used to automatically segment kidneys and tumors and geometric algorithms were developed to estimate each component of PADUA based on the segmented regions (P-AI). Tumors were also manually scored by medical personnel blinded to the P-AI (P-H). The ability of P-AI and P-AI+ to predict meaningful perioperative and oncologic outcomes was compared to P-H using logistic regression and receiver operating characteristics (ROC) curve analyses and areas under the curve (AUC). Results: Median age was 60 years, 40% were female. Median tumor size was 4.2 cm, 91.3% had malignant tumors, including 27% and 37% with high-stage and high-grade, respectively. Both P-AI and P-H were able to predict the need for partial nephrectomy (p < 0.001). From an oncologic standpoint, P-AI and P-H were able to predict meaningful oncologic outcomes including the presence of malignancy, high grade and high stage disease (p < 0.004) although the ROC curves were not different from one another). Interestingly, when each PADUA component was left as a continuous rather than ordinal variables (P-AI+), this automated continuous score was able to predict surgical type (AUC 0.88), presence of malignancy (AUC 0.67), indolent tumors (AUC 0.79) and high grade (AUC 0.77)/high stage disease (AUC 0.82), better than both P-AI and P-H. Conclusions: When viewed along a continuous spectrum, AI generated PADUA scores (P-AI+) yields predictive surgical and oncologic outcomes superior to both categorical AI and human generated PADUA scores. If nephrometry can be calculated automatically, there is no longer a need to simplify the equations and the use of an AI generated PADUA scoring system, provides a reliable estimate of meaningful outcomes in a manner that is time sensitive and superior to human expert evaluation. Further prospective work and reproducibility from other centers is encouraged.
更多查看译文
关键词
padua nephrometry scores,perioperative outcomes,predictive accuracy,computer-generated,human-generated
AI 理解论文
溯源树
样例
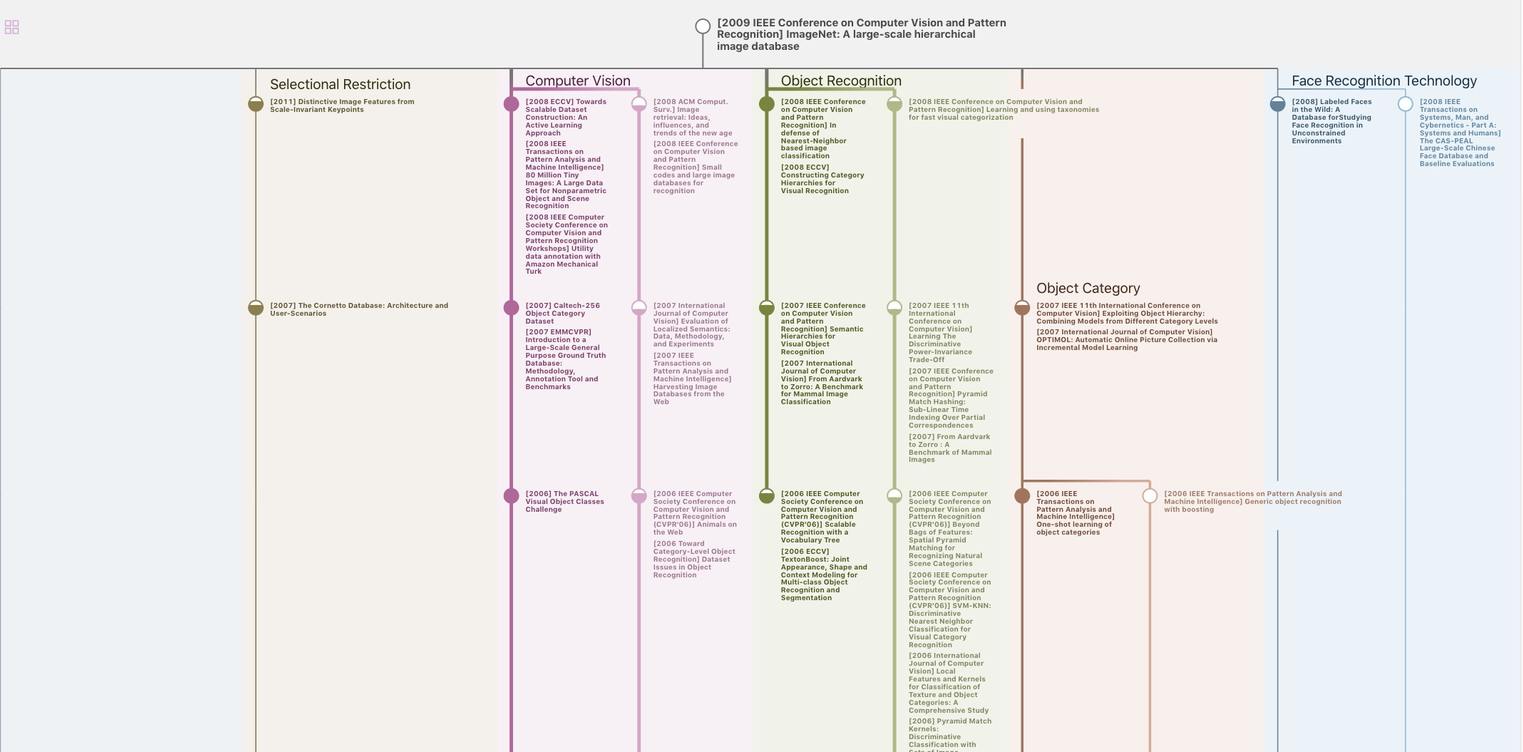
生成溯源树,研究论文发展脉络
Chat Paper
正在生成论文摘要