Prediction of Urban Water Demand Based on Improved PCA-SSA-Elman Dynamic Neural Network
Research Square (Research Square)(2022)
摘要
Abstract In principal component analysis, data logarithm transformation and row vector centralization improvement are carried out, reducing the input, and simplifying the network model. Use sparrow search algorithm for optimization, and compare the performance with cuckoo algorithm, seagull algorithm, and whale algorithm. To avoid problems as slow convergence speed and insufficient exploration ability, an improved sparrow search algorithm integrating refraction inverse learning mechanism, sin-cosine and Cauchy variation is proposed, a high-precision PCA-SCSSA-Elman neural network prediction model is established. The results show that SSA-Elman neural network has the fastest convergence speed and the optimal performance value is 0.1391 higher. Combined with the extracted principal component data, compared with PCA-Elman, PCA-SSA-Elman and PCA-SineSSA-Elman prediction models, the mean absolute error is higher than the other three models [45.6850%, 14.9168%, 11.7448%], the prediction error is minimal. The results show that the improved sparrow search algorithm can significantly improve the convergence speed and optimization accuracy.
更多查看译文
关键词
urban water demand,neural network,prediction,pca-ssa-elman
AI 理解论文
溯源树
样例
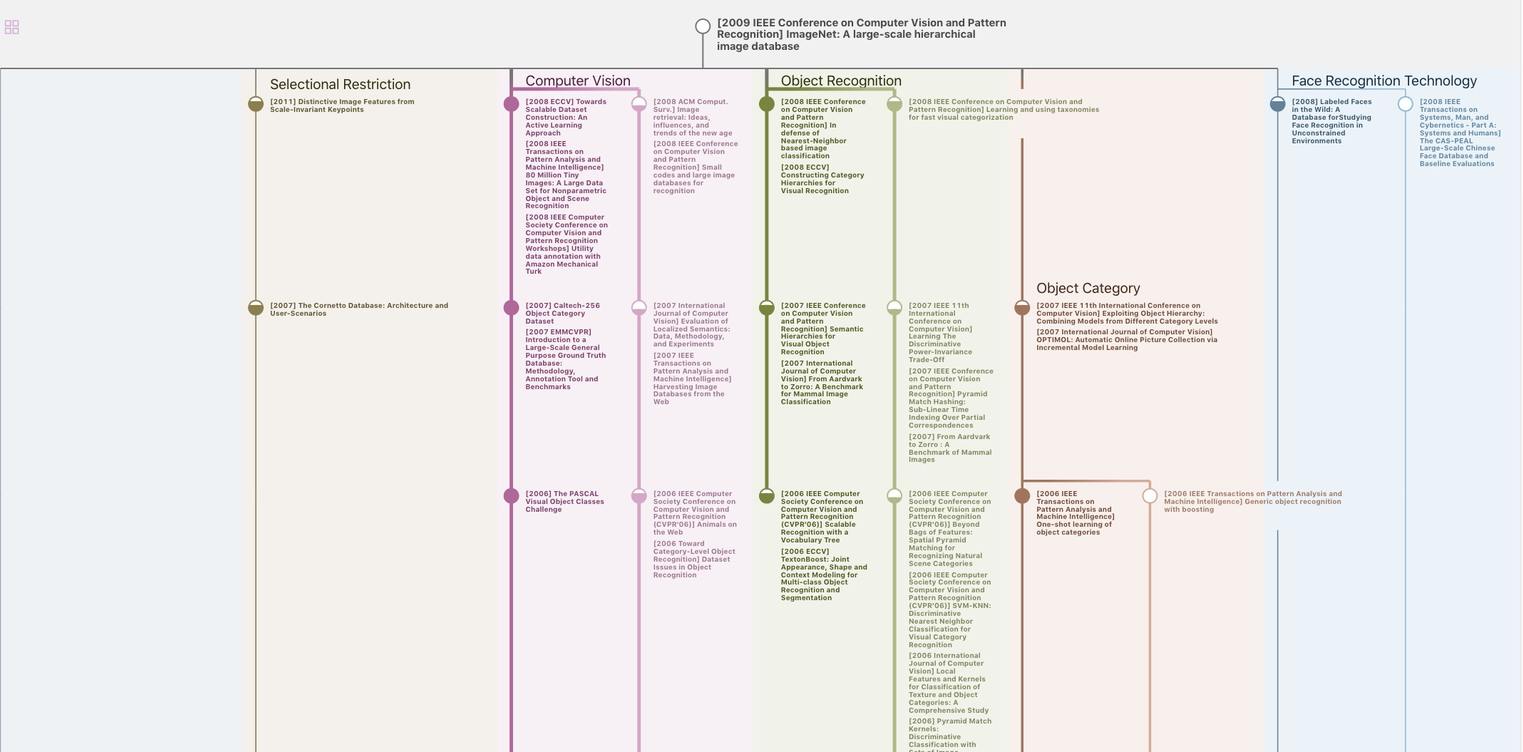
生成溯源树,研究论文发展脉络
Chat Paper
正在生成论文摘要