FPGA Accelerated Real-time Recurrent All-Pairs Field Transforms for Optical Flow
2022 China Automation Congress (CAC)(2022)
摘要
Optical flow algorithms based on deep learning have achieved excellent performance on multiple datasets, bringing new opportunity for optical flow estimation. Recurrent All-Pairs Field Transforms (RAFT) is one of the most powerful deep network based optical flow algorithms, but it is difficult to process in real time on the resource-limited embedded platform. In this paper, we propose RAFT-Lite by compressing the original RAFT model, which is more lightweight and suitable for hardware deployment. We further propose a hardware accelerating architecture on FPGA for RAFT-Lite, which provides an efficient scheduling strategy for the convolution in RAFT to achieve efficient pipeline and resource reuse. On Xilinx ZCU102 evaluation board, the accelerated hardware system can reach 10.4fps processing images with a resolution of 512*396, which is 6.8x of i7-10700@2.90GHz and 46x of ARM Cortex-A53@1.50GHz. Besides, the power consumption is 13.103W.
更多查看译文
关键词
Optical flow,FPGA,Deep learning,RAFT,hardware acceleration
AI 理解论文
溯源树
样例
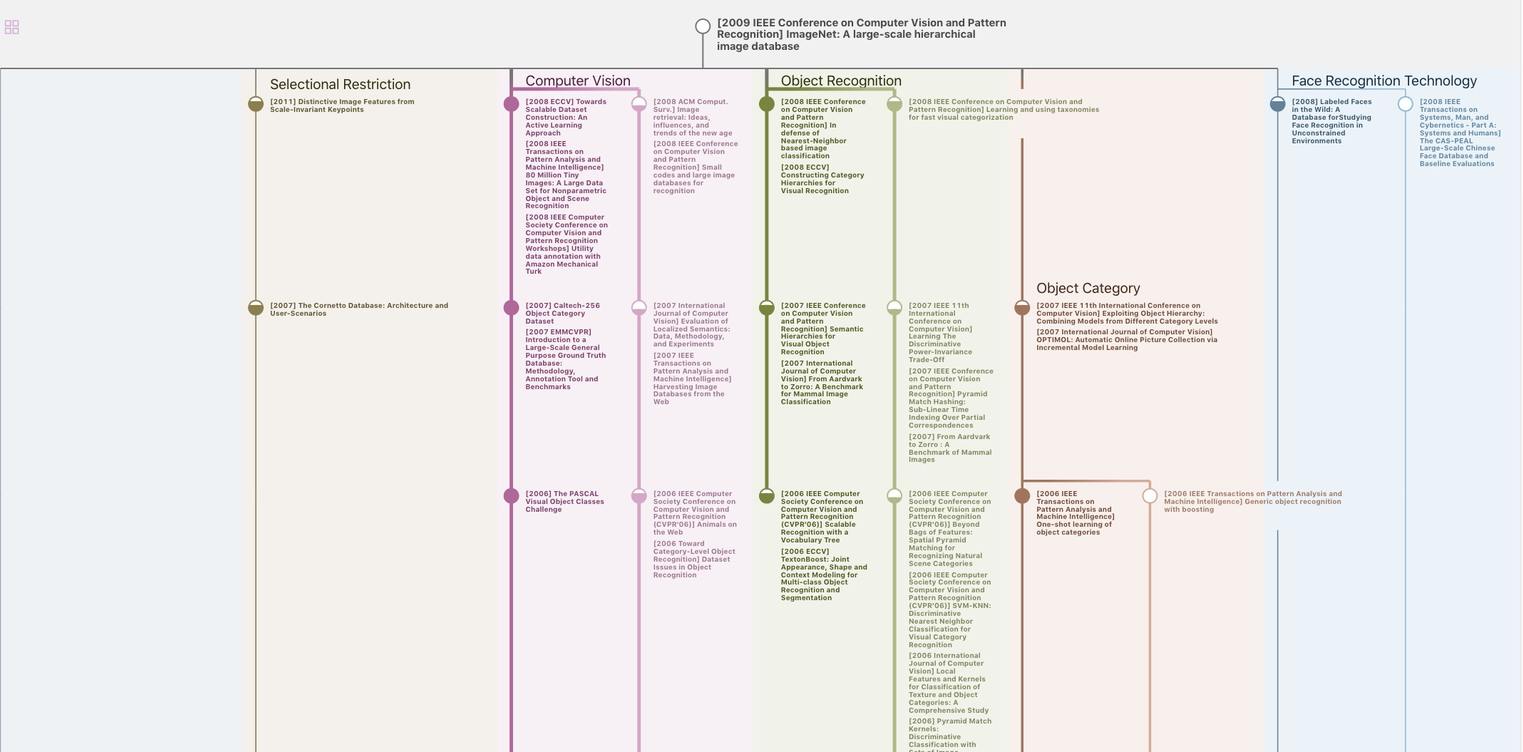
生成溯源树,研究论文发展脉络
Chat Paper
正在生成论文摘要