Vehicle Extreme Control based on Offline Reinforcement Leaning
2022 China Automation Congress (CAC)(2022)
摘要
This paper studies the extreme driving control of vehicles based on offline reinforcement learning (RL). Our work is mainly about the racing car, which has complex dynamic characteristics and is more difficult to control than steady-state drift. In order to ensure safety and fast convergence, we use the framework that takes a traditional control method as a basic policy and takes offline RL to fine-tune the imperfect traditional controller. For the basic policy, we use the principle of minimum curvature to generate a reference trajectory and a non-linear feedback controller to track the trajectory. For the fine-tune item, we use a simple yet state-of-the-art offline RL algorithm called TD3+BC to add some adjustive to the base policy. For the simulation platform, we use Matlab Simulink combined with the Silverstone track data as the environment, and use Carsim to provide vehicle dynamic models. The simulation results show that the proposed control policy can effectively shorten the time for the car to complete the track.
更多查看译文
关键词
extreme driving control,racing car,non-linear feedback control,offline RL
AI 理解论文
溯源树
样例
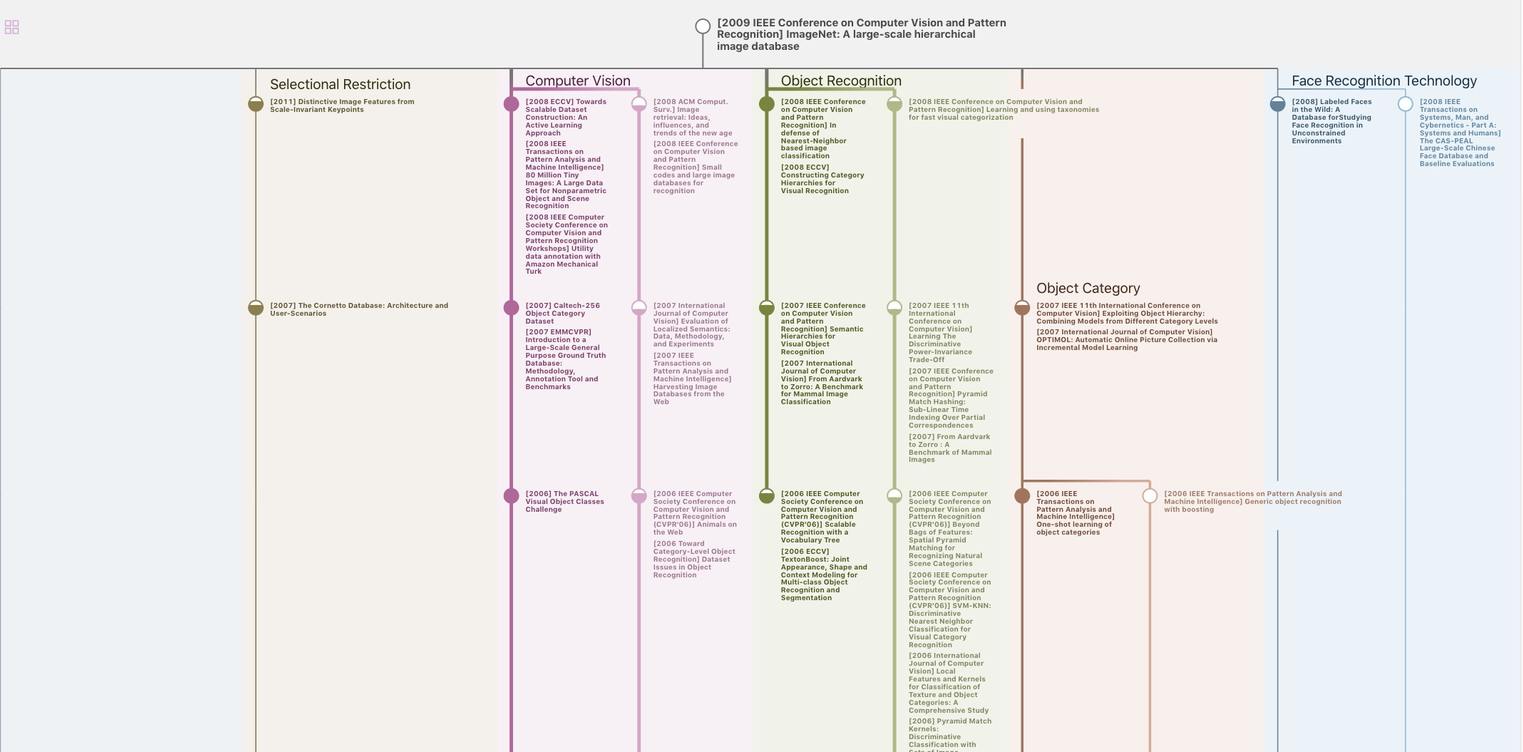
生成溯源树,研究论文发展脉络
Chat Paper
正在生成论文摘要