Proper Reuse of Features Extractor for Real-time Continual Learning
2022 China Automation Congress (CAC)(2022)
摘要
Real-time continual learning which could continually learn a series of computer vision tasks, has received more attention on robotic platforms and embedded systems with on-device training manner. However, most existing real-time continual learning systems need more training time when learning new visual perception tasks; the class imbalance issues are neglected amongst most real-time continual learning systems, which could degrade the generalization performance among past learned tasks, as well as the new tasks. To address these challenges above, we in this work propose to preserve and reuse the learned knowledge to achieve real-time continual learning. To be specific, when encountering a new visual perception task, we choose to freeze the learned backbone weights of all the past tasks, which could speedup the training process for new tasks. Moreover, we also store several activations volumes from some intermediate layer, which could further reduce the computational cost. For the improved perception performance, a focal loss is employed to guide the attention for poorly-identified sample categories and mitigate the class imbalance issue. Experimental results on popularly-used continual learning justify the efficiency and effectiveness of our proposed method.
更多查看译文
关键词
Continual learning,Knowledge replay,Real-time systems,light computational devices,stored activations
AI 理解论文
溯源树
样例
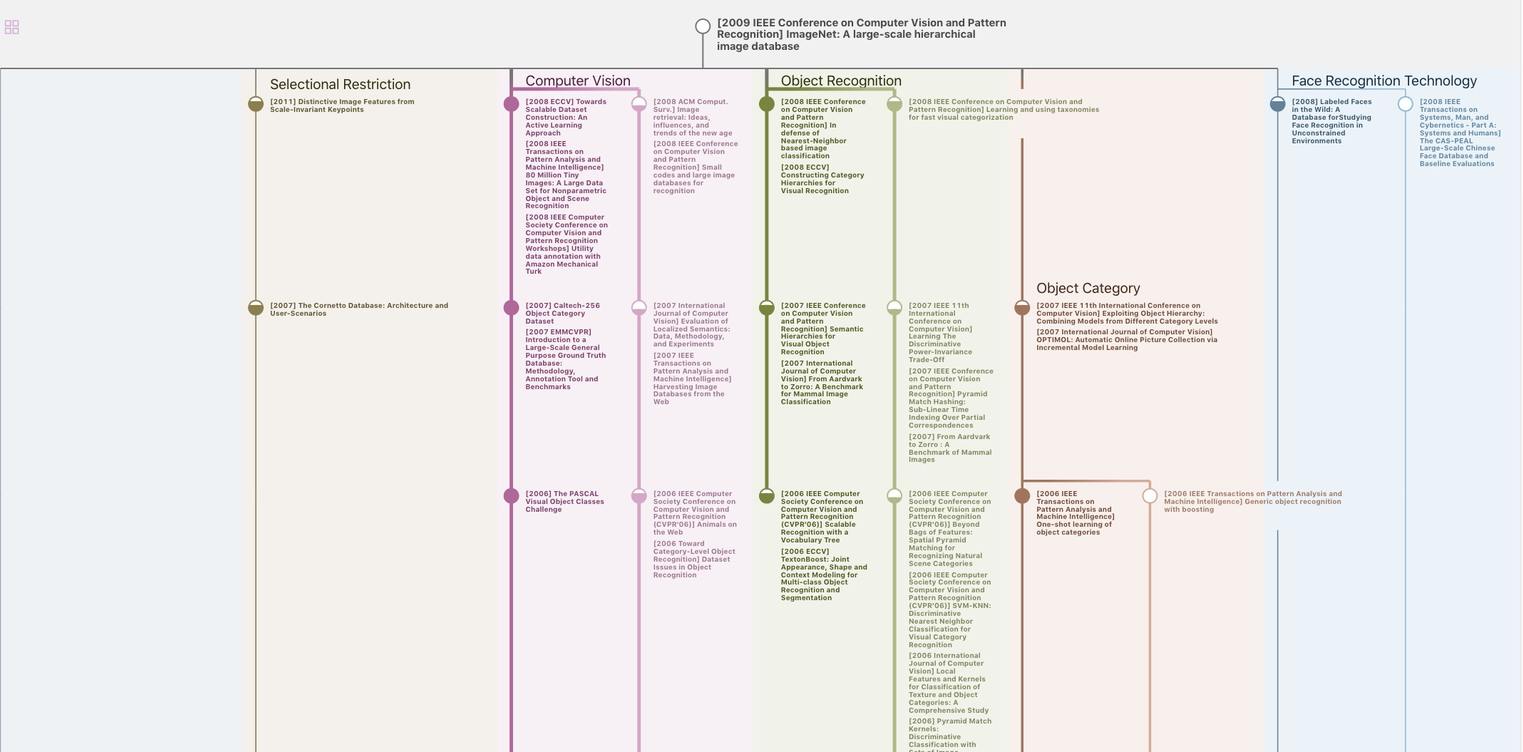
生成溯源树,研究论文发展脉络
Chat Paper
正在生成论文摘要