Assessing the impact of weather forecast uncertainties in crop water stress model predictions
AGRICULTURAL AND FOREST METEOROLOGY(2024)
摘要
Since agriculture is highly exposed to weather-related risks such as drought constraints, Decision Support Tools (DSTs) are now frequently used in irrigation management. The current use of DSTs mainly relies on deterministic weather forecasts that do not account for the associated weather uncertainties. Few irrigation DST users take uncertainty into consideration by utilizing an ensemble of historical weather observations (EHO) as input to their models. In order to account for the atmospheric state uncertainties, weather centers are progressively shifting to ensemble predictions. This is done by running several times the numerical weather prediction model with slightly perturbed initial conditions and model formulations. The final forecast hence takes the form of an ensemble instead of a deterministic single value. However, ensemble forecasts could present bias and dispersion errors and therefore need post-processing. In this work, we introduce a novel approach that uses ensemble weather forecasts as input to a vine irrigation DST, named "Water baLance for Intercropped Systems"(WaLIS), that computes a crop water stress index as output. Our results show that this approach has significantly better probabilistic performance than the use of EHO as input to the WaLIS model, especially between forecast days 5 and 7 (similar to 35% of gain in performance). We also investigate the effect of statistical post-processing on the probabilistic skill of the water stress index with two different approaches. In the first case, we directly post-process the output of the WaLIS model. In the second case, only weather forecasts are post-processed, before being used as inputs of the WaLIS model. Our results show that locally in 4 out of 10 tested sites in southern France, the raw ensemble forecasts could be significantly improved by the post-processing, and that generally there is no significant performance difference between the two competing post-processing approaches.
更多查看译文
关键词
Vineyard water stress,Ensemble prediction,Weather data,Forecast uncertainty,Post-processing
AI 理解论文
溯源树
样例
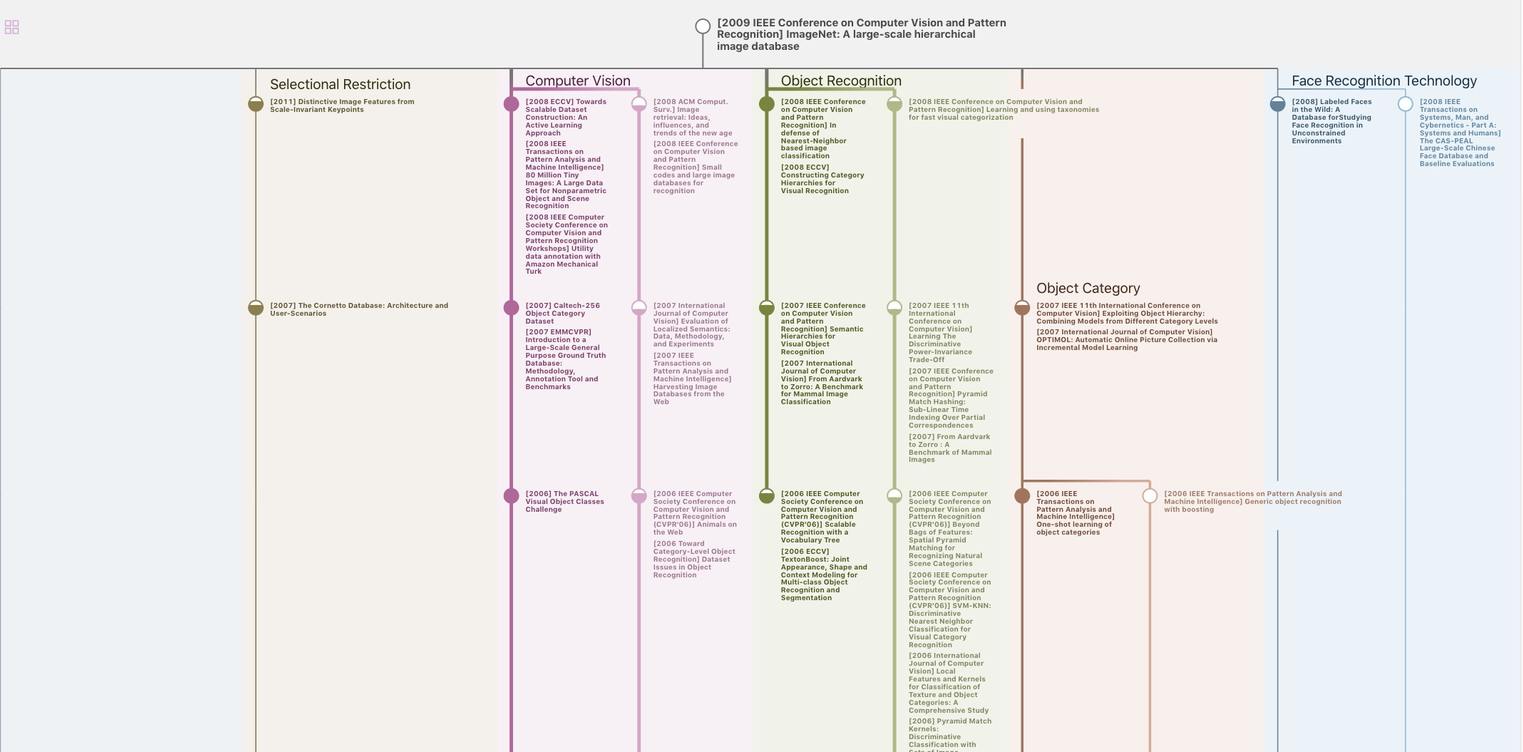
生成溯源树,研究论文发展脉络
Chat Paper
正在生成论文摘要