A Study of Pixelwise Segmentation Metrics Using Clustering of Variables and Self-Organizing Maps
XXXI INTERNATIONAL HORTICULTURAL CONGRESS, IHC2022 III INTERNATIONAL SYMPOSIUM ON MECHANIZATION, PRECISION HORTICULTURE, AND ROBOTICS PRECISION AND DIGITAL HORTICULTURE IN FIELD ENVIRONMENTS(2023)
摘要
A considerable number of metrics can be used to evaluate the performance of machine learning algorithms. While much work is dedicated to the study and improvement of data quality and models' performance, much less research is focused on the study of these evaluation metrics, their intrinsic relationship, the interplay of influence among the metrics, the models, the data, and the conditions in which they are to be applied. While some works have been conducted on general machine learning tasks like classification, fewer have been dedicated to more complex problems such as object detection and image segmentation, in which the evaluation of performance can vary drastically depending on the objectives and domains of application. Working in an agricultural context, specifically on the problem of automatic detection of plants using image segmentation models, we present and study 12 evaluation metrics, which we use to evaluate three segmentation models on the same train and test sets of images. Within an exploratory framework, we study the relationship among these 12 metrics using clustering of variables and self-organizing maps. We identify three groups of highly linked metrics, each emphasizing a different aspect of the quality of segmentation, which are in alignment with both the theoretical definitions of the metrics, and human visual inspection. Finally, we provide interpretations of these metrics in our agricultural context and some clues to practitioners for understanding and choosing the metrics that are most relevant to their agricultural task.
更多查看译文
关键词
Self-Organizing Maps,Spectral Clustering,Ensemble Methods,Feature Selection,Variable Selection
AI 理解论文
溯源树
样例
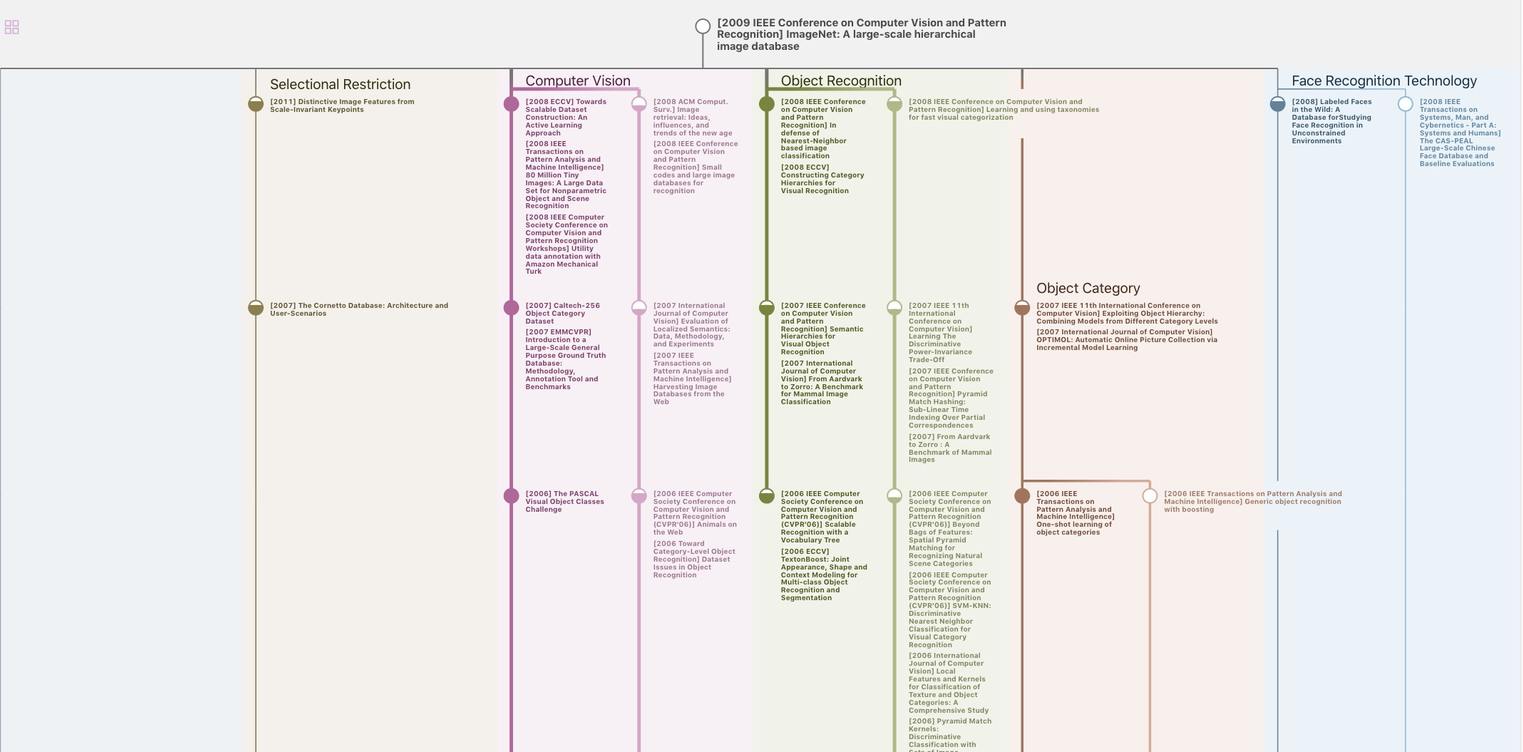
生成溯源树,研究论文发展脉络
Chat Paper
正在生成论文摘要