Safe Exploration of Reinforcement Learning with Data-Driven Control Barrier Function
2022 China Automation Congress (CAC)(2022)
摘要
Reinforcement learning relies on exploration and exploitation to find optimal policies. However, unconstrained exploration might lead to unsafe actions that jeopardize the system safety. To address this issue, this work presents a RL-based framework that integrates model-based CBF to ensure safe exploration during learning. Rather than synthesizing CBF by hand for complex dynamic systems, we exploit data-driven methods to learn CBFs from collected demonstrations of safe and desirable behavior. Unlike prior works that restrict on off-line collected expert demonstrations to train CBF, the CBF in this work is learned not only from preliminary expert demonstrations, but also from the on-line generated data at runtime, resulting in improved adaptation to complex environments. Numerical simulations and physical experiments using Crazyflie quadrotors are carried out to demonstrate the effectiveness of the developed safe RL framework. The experiment video is available at https://youtu.be/uscl-BQsLRo.
更多查看译文
关键词
Control barrier function,reinforcement learning,shield,data-driven
AI 理解论文
溯源树
样例
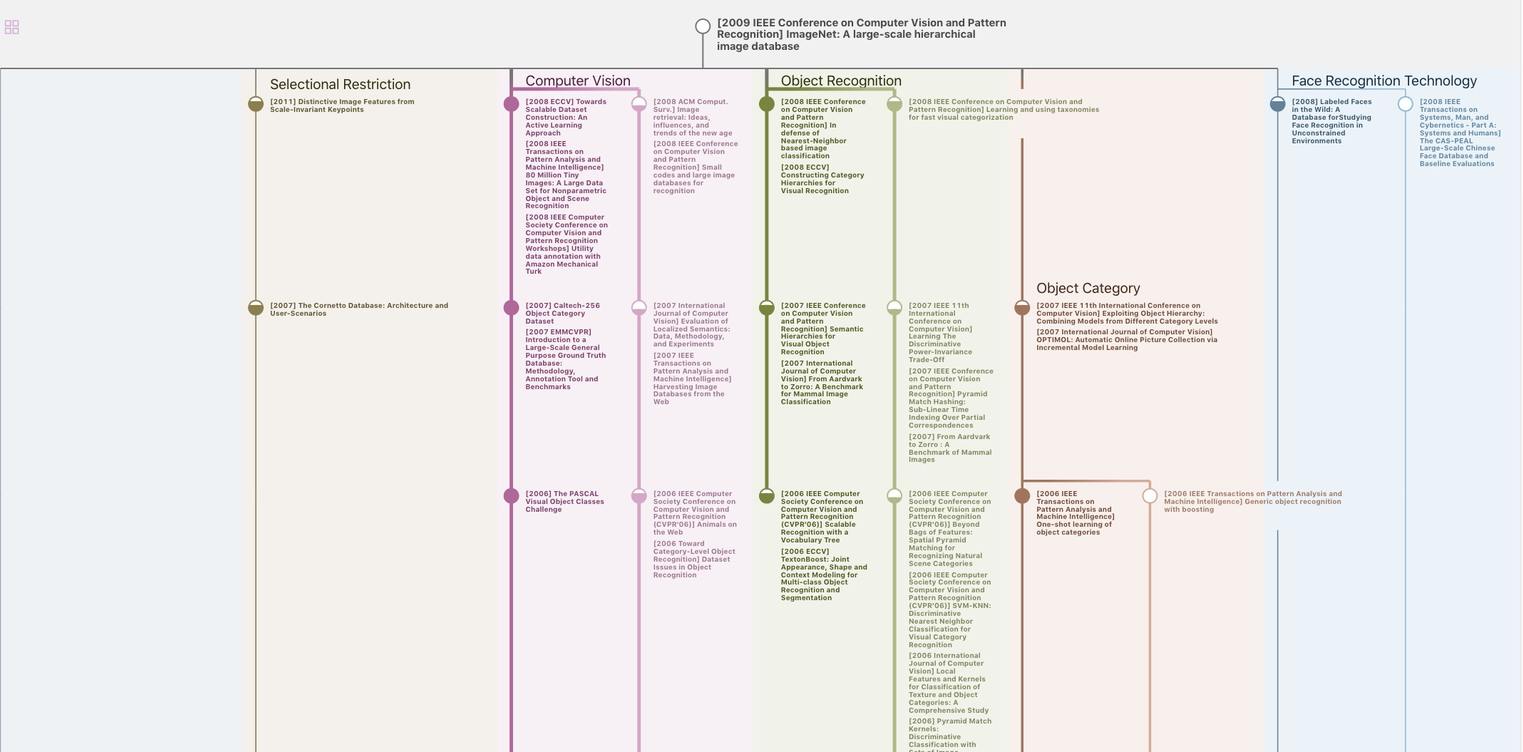
生成溯源树,研究论文发展脉络
Chat Paper
正在生成论文摘要