Pedestrian Trajectory Prediction by Modeling the Interactions using Social LSTM Extensions
2022 China Automation Congress (CAC)(2022)
摘要
In many driving situations, human mobility is an important topic in trajectory prediction. Considering the pedestrian trajectory as a sequence generative task, a prediction algorithm based on Social Long Short-Term Memory (Social LSTM) is implemented. In order to simulate the social interaction between pedestrians, Social Pooling (S-Pooling) is used to aggregate the hidden state of pedestrians, while the attention mechanism is utilized to aggregate information differently according to the importance of surrounding pedestrians. Furthermore, Convolutional Neural Networks (CNN) is introduced into Social LSTM model to consider both the interaction between people and the characteristic of scene scale in the prediction process. Experiments are carried out against baseline methods, and the results demonstrated that combining Social LSTM with attention mechanism or CNN can improve the performance of pedestrian trajectory prediction.
更多查看译文
关键词
Pedestrian Trajectory Prediction,Attention,CNN,LSTM
AI 理解论文
溯源树
样例
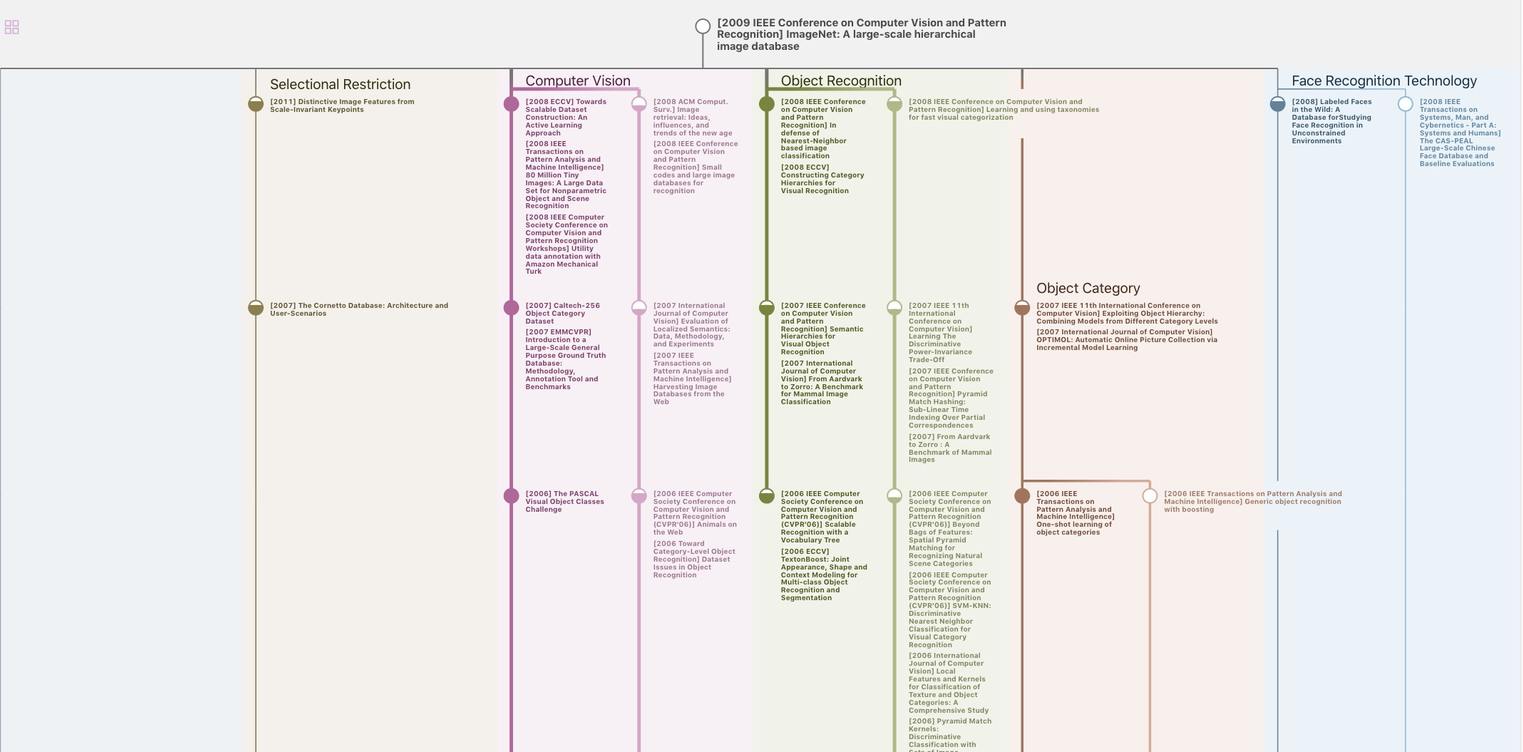
生成溯源树,研究论文发展脉络
Chat Paper
正在生成论文摘要