Polymer graph neural networks for multitask property learning
npj Computational Materials(2023)
摘要
The prediction of a variety of polymer properties from their monomer composition has been a challenge for material informatics, and their development can lead to a more effective exploration of the material space. In this work, PolymerGNN , a multitask machine learning architecture that relies on polymeric features and graph neural networks has been developed towards this goal. PolymerGNN provides accurate estimates for polymer properties based on a database of complex and heterogeneous polyesters (linear/branched, homopolymers/copolymers) with experimentally refined properties. In PolymerGNN , each polyester is represented as a set of monomer units, which are introduced as molecular graphs. A virtual screening of a large, computationally generated database with materials of variable composition was performed, a task that demonstrates the applicability of the PolymerGNN on future studies that target the exploration of the polymer space. Finally, a discussion on the explainability of the models is provided.
更多查看译文
关键词
Polymer characterization,Polymers,Theoretical chemistry,Materials Science,general,Characterization and Evaluation of Materials,Mathematical and Computational Engineering,Theoretical,Mathematical and Computational Physics,Computational Intelligence,Mathematical Modeling and Industrial Mathematics
AI 理解论文
溯源树
样例
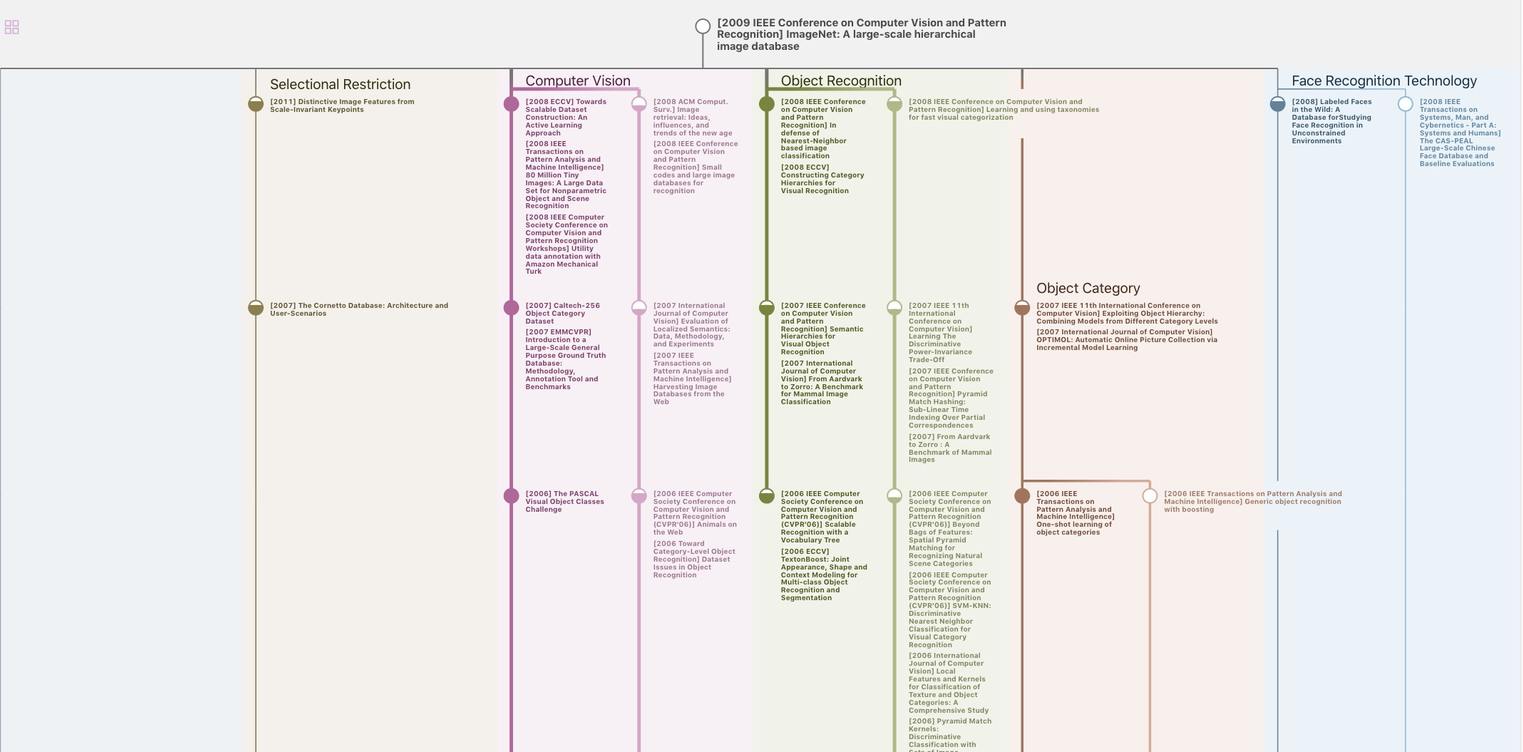
生成溯源树,研究论文发展脉络
Chat Paper
正在生成论文摘要