ORO-YOLO: An Improved YOLO Algorithm for On-Road Object Detection
Proceedings of 2022 International Conference on Autonomous Unmanned Systems (ICAUS 2022)Lecture Notes in Electrical Engineering(2023)
摘要
Environment perception and object detection are the foundation for unmanned ground vehicles (UGV) to autonomously complete various tasks, and also one of the important research topics in the field of unmanned systems. On-Road object detectors based on deep learning have made significant progress, but it is still difficult to balance the detection accuracy and speed. In this paper, an improved fast on-road object detection algorithm ORO-YOLO based on YOLOX network is proposed to solve this problem. First, we improve the backbone network by the reparameterization method to enhance the network feature extraction capability. Second, a feature enhancement module is presented for the actual road target aspect ratio, which enables the network to be more suitable for on-road object detection tasks. Moreover, an adaptive feature fusion module is introduced to replace the original feature pyramid module to further compress the network size. Comparative experiments on KITTI and BDD100k datasets demonstrate the effectiveness of the proposed algorithm, with up to 2% higher accuracy than the original network and about 10% reduction in the amount of network parameters.
更多查看译文
关键词
Unmanned ground vehicles, Real time, On-road object detection, YOLO
AI 理解论文
溯源树
样例
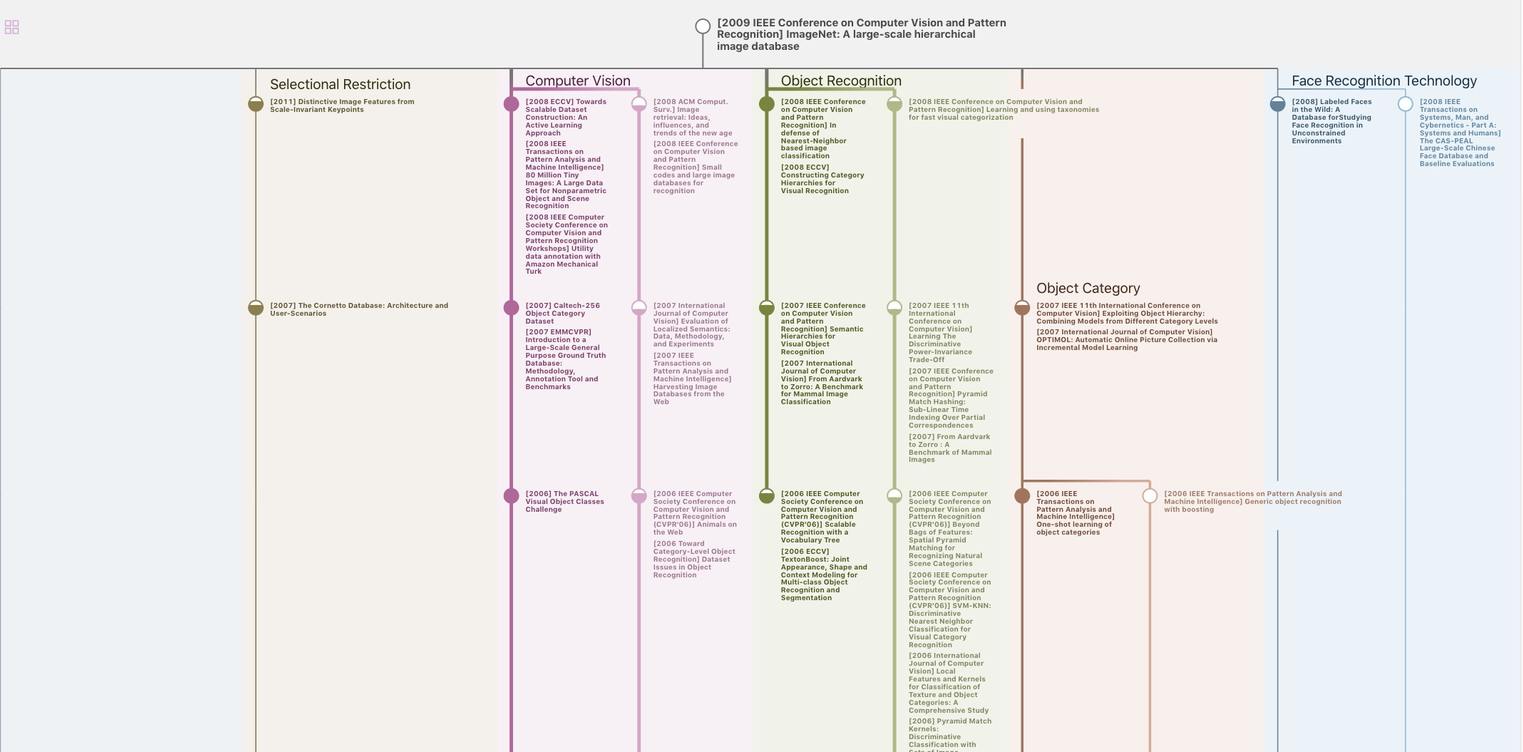
生成溯源树,研究论文发展脉络
Chat Paper
正在生成论文摘要