Initiatives, Concepts and Implementation Practices of FAIR Data Principles in Health Data Stewardship: A Scoping Review (Preprint)
crossref(2022)
摘要
BACKGROUND Thorough data stewardship drives health research. Processes such as data collection, storage, access, sharing, and analytics require that the researchers properly and consistently follow elaborate data management strategies. Studies have shown that ensuring data is findable, accessible, interoperable and reusable (FAIR) indeed leads to improved data sharing and harmonization in different facets of scientific domains. Comprehensive information about the concepts and implementation practices of the FAIR data principles as applied to health research data helps to gain a better understanding of the current state of affairs within the field. OBJECTIVE This scoping review aims to identify concepts, approaches, implementation experiences and lessons learned in FAIR initiatives in health research data. METHODS The Arksey and O’Malley stage-based methodological framework for scoping reviews was applied in this review. PubMed, Web of Science and Google Scholar were searched to access relevant publications. As indicated in our earlier scoping review protocol, articles written in English, published from 2014 to 2020 and addressing FAIR concepts or practices in the health domain were included. The three data sources were de-duplicated using a reference management software. The articles were then exported to a systematic review management software. Two independent authors reviewed the eligibility of each article based on defined inclusion and exclusion criteria. A charting tool was used to extract information from the full text papers. The results were reported using the PRISMA scoping review reporting guidelines. RESULTS 34 of 1,544 screened articles were included in the final review. Authors reported FARIfication approaches which include interpolation, inclusion of comprehensive data dictionaries, repository design, semantic interoperability, ontologies, data quality, linked data and requirements gathering for FAIRification tools. We also summarized the reported challenges and mitigation strategies associated with FAIRification such as high set up costs, data politics, technical issues, administrative issues, privacy concerns and difficulties encountered in sharing health data in spite of its sensitive nature. We also found various workflows, tools and infrastructure designed by different groups worldwide to facilitate the FAIRification of data in different specialities within the health domain. However, the steps involved in the workflows, tools and infrastructure vary. We also uncovered a wide range of problems and questions that researchers are trying to address by employing the different workflows, tools and infrastructure to FAIRify their data. Although the concept of FAIR data stewardship in the health research domain is relatively new, almost all the continents have been reached by at least one network that is trying to achieve health data FAIRness. Documented outcomes of the FAIRification efforts by different researchers include peer-reviewed publications, improved data sharing, facilitated data reuse, return on investment and new treatments. More work aiming to FAIRify data on a wider variety of diseases is ongoing since the COVID pandemic. Successful FAIRification of data informs the management and prognosis of various diseases such as Huntington disease, cancer, traumatic stress disorder and cardiovascular diseases. CONCLUSIONS Conducting this review led us to analyse the work done by various projects to FAIRify health research data. FAIRification leads to improved data management and governance. The addition of rich metadata does facilitate data discovery and allows that data to serve a greater audience. This comprehensive overview also shows that implementing FAIR concepts in health data stewardship supports both evidence-based clinical practice and research transparency in the era of big data and open research publishing. INTERNATIONAL REGISTERED REPORT RR2-10.2196/22505
更多查看译文
AI 理解论文
溯源树
样例
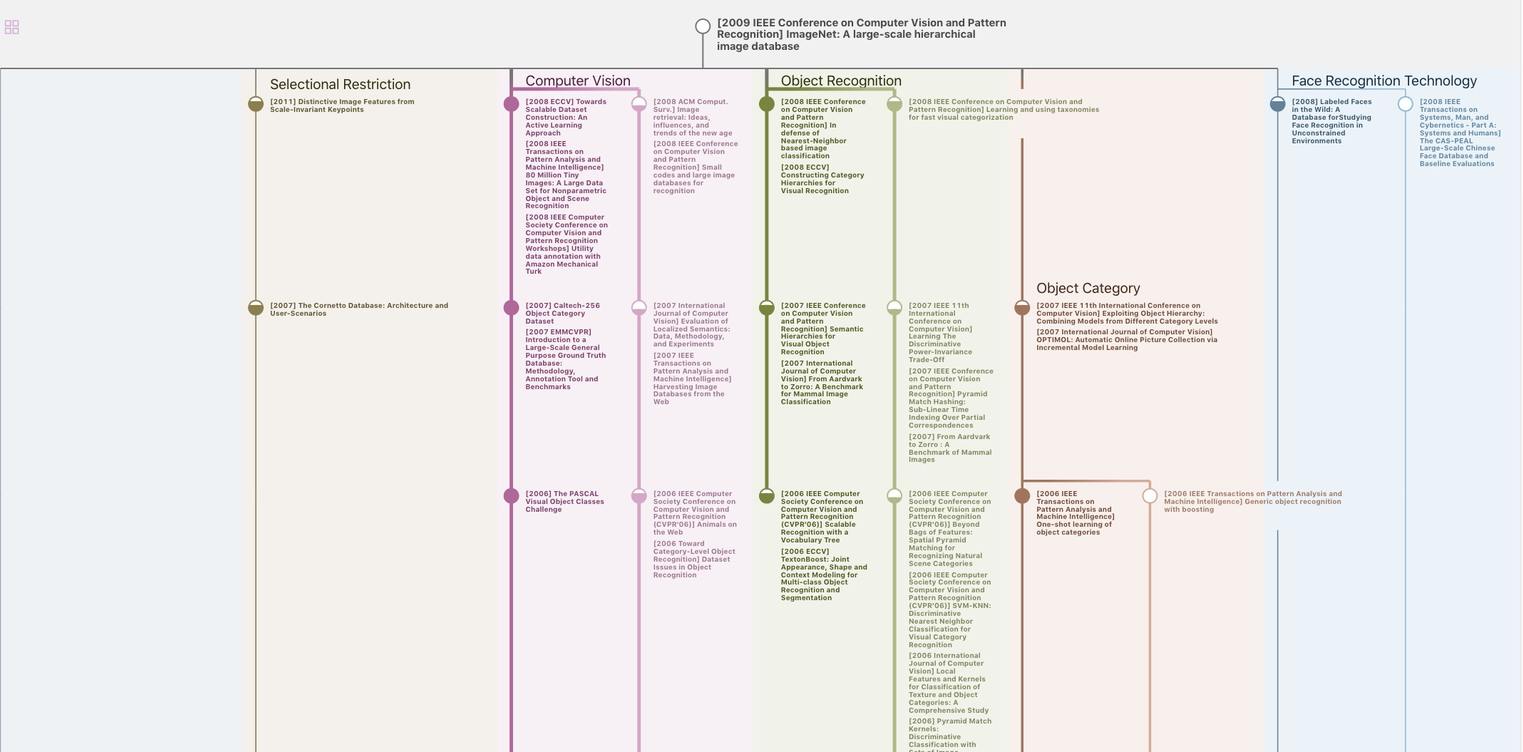
生成溯源树,研究论文发展脉络
Chat Paper
正在生成论文摘要